The financial services industry is undergoing a big shift, with machine learning (ML) leading the charge. According to Fortune Business Insights, the global machine learning market is projected to reach USD 209.91 billion by 2030, reflecting a compound annual growth rate (CAGR) of 38.8% from 2023. This explosive growth is driven by ML’s potential to revolutionize processes across multiple sectors, and asset management is no exception.
For asset managers, ML is not just a buzzword—it’s a transformative tool that is fundamentally changing how investments are managed, risks are mitigated, and opportunities are identified. In asset management, machine learning is helping companies transition from reactive decision-making to proactive, data-driven strategies.
By analyzing vast amounts of data in real-time, ML algorithms provide predictive insights that can reveal opportunities and threats before they become obvious. Moreover, machine learning’s ability to automate processes, reduce operational costs, and optimize investment strategies has made it a key differentiator for those seeking a competitive edge in an increasingly complex financial landscape. From enhancing decision-making to improving operational efficiency, machine learning is reshaping the asset management industry—and those who adapt will be the ones who thrive in this new era.
What is Machine Learning in Asset Management?
Machine learning in the context of asset management refers to the use of sophisticated algorithms and data models to analyze and predict market trends, optimize portfolios, and manage risk. Unlike traditional analytics, which relies on static, human-defined rules, machine learning learns autonomously from large datasets, making it a crucial tool for identifying opportunities and managing risk in dynamic markets.
Traditional data analytics often falls short when adapting to rapid market changes, as it is limited by predefined rules. Machine learning, on the other hand, uses advanced techniques to analyze vast amounts of data, uncovering trends and insights that may be missed by human analysts. This capability allows asset managers to make faster, more informed decisions, significantly improving the accuracy of investment strategies. Machine learning continually learns and adapts, providing evolving insights that align with changing market conditions.
Machine learning systems process both structured and unstructured data, such as historical prices, trading volumes, economic indicators, and even text from news or social media. By leveraging these diverse data sources, ML models offer a comprehensive view of the market in real time. This adaptability is especially valuable in fast-moving financial markets, where strategies must evolve quickly. Machine learning keeps asset managers ahead of the competition by enabling both proactive and reactive responses to market shifts.
Key Benefits of Machine Learning for Asset Managers
Machine learning is reshaping asset management by simplifying complex tasks, enhancing data-driven insights, and optimizing portfolio strategies. By leveraging these capabilities, asset managers can make smarter decisions faster, ultimately gaining a competitive edge in an ever-changing market.
1. Enhanced Decision-Making
ML algorithms process large volumes of data, including market indicators, news, and social media, to provide actionable insights. Unlike traditional methods, ML-based analysis adapts as new data comes in, keeping asset managers informed.
The speed at which ML operates provides a big advantage, enabling quicker and more accurate decisions. ML also identifies hidden relationships in data that lead to deeper insights, such as predicting stock price changes based on historical performance, market sentiment, and macroeconomic trends.
2. Risk Management
Managing market risks is an essential part of asset management. It analyzes historical data and real-time activity to identify risks early. By combining different data types, ML can detect subtle risk indicators that might be overlooked by traditional approaches.
ML allows asset managers to adjust their strategies before problems arise. Machine learning can also simulate various scenarios to predict asset behavior, helping managers identify weak spots and prepare accordingly. Anomaly detection tools can flag unusual activities, helping reduce risks and maintain portfolio stability.
3. Operational Efficiency
Machine learning greatly boosts operational efficiency. Repetitive tasks like portfolio rebalancing and trade execution can be automated, reducing human error and improving consistency.
Automation allows asset managers to focus on more strategic work. ML also provides real-time market monitoring, ensuring that decisions are timely and effective. By processing news and extracting key data, ML keeps managers informed without overwhelming manual effort.
Applications of Machine Learning in Asset Management
Machine learning is revolutionizing asset management by enabling faster trade execution, smarter portfolio optimization, and deeper insights into market sentiment. These capabilities help asset managers stay ahead, make informed decisions, and guard against fraud—all while staying agile in an ever-changing market environment.
Algorithmic Trading
- Transforms trading with real-time insights and ultra-fast execution.
- Continuously learns from data to adapt strategies and identify opportunities.
- Uses reinforcement learning for split-second decisions in volatile markets.
Portfolio Optimization
- Analyzes market trends, investor goals, and risk factors for better asset allocation.
- Makes real-time adjustments to maximize returns and minimize risks.
- Employs predictive modeling and clustering to maintain an optimal risk-reward balance.
Sentiment Analysis
- Processes data from news, social media, and financial reports to gauge market sentiment.
- Predicts sentiment shifts, enabling proactive responses from asset managers.
- Utilizes natural language processing (NLP) to assess news sentiment as positive, negative, or neutral.
Fraud Detection
- Enhances fraud detection by recognizing unusual patterns in financial data.
- Learns to identify behaviors that deviate from the norm for early fraud detection.
- Combines supervised learning for known fraud patterns with unsupervised learning for new threats.
Machine Learning Techniques Used in Asset Management
Machine learning in asset management uses supervised, unsupervised, and deep learning to make predictions, find patterns, and analyze data.
Supervised Learning
Supervised learning involves using labeled historical data to predict outcomes, such as stock prices or portfolio performance. By learning the relationship between input factors like economic indicators and target outcomes, ML models can generate data-driven investment insights.
- Common Techniques: Linear regression is used for predicting trends, while decision trees help classify financial data.
- Use Cases: These methods are effective when there are clear patterns between historical data and future events, helping asset managers make more informed decisions.
Unsupervised Learning
Unsupervised learning, unlike supervised learning, works with data that lacks pre-defined labels. This method is especially useful for discovering hidden relationships and grouping assets in ways that are not obvious to human analysts.
- Clustering: In asset management, clustering is often used to group stocks with similar characteristics, aiding diversification and portfolio construction.
- Benefits: By breaking down complex data into manageable groups, unsupervised learning enables asset managers to identify new opportunities and make better decisions based on emerging patterns.
Deep Learning and Neural Networks
Deep learning and neural networks are particularly powerful for analyzing unstructured data, such as news articles, financial reports, and social media feeds. These models can learn complex, non-linear relationships within data, offering an edge when predicting market trends or analyzing investor sentiment.
- Tools: Convolutional neural networks (CNNs) excel at extracting meaningful information from large datasets, while recurrent neural networks (RNNs) are ideal for time-series predictions.
- Impact: This forward-looking analysis helps asset managers stay ahead of market movements and make timely, strategic investment decisions.
Challenges of Implementing Machine Learning in Asset Management
1. Data Quality and Availability
High-quality data is essential for ML but often challenging to obtain. Gaps or errors in data can lead to inaccurate outcomes, making investment in data infrastructure critical.
Privacy regulations also add complexity, requiring careful compliance. Many firms are now adopting data governance frameworks and collaborating with specialized providers to ensure quality.
2. Model Interpretability
ML models, particularly deep learning, can be difficult to interpret. In finance, where transparency is key, the “black box” nature of ML is a challenge.
Explainable AI (XAI) helps make these models more transparent. By understanding which factors drive decisions, asset managers can foster trust and meet regulatory needs.
3. Regulatory Compliance
Compliance is another major hurdle. ML models must be auditable and compliant with regulations like GDPR. This requires rigorous documentation and validation processes.
A robust governance framework helps asset managers ensure their models meet evolving regulatory requirements while still benefiting from ML capabilities.
Case Studies: Machine Learning in Action
Example 1: BlackRock’s Aladdin Platform
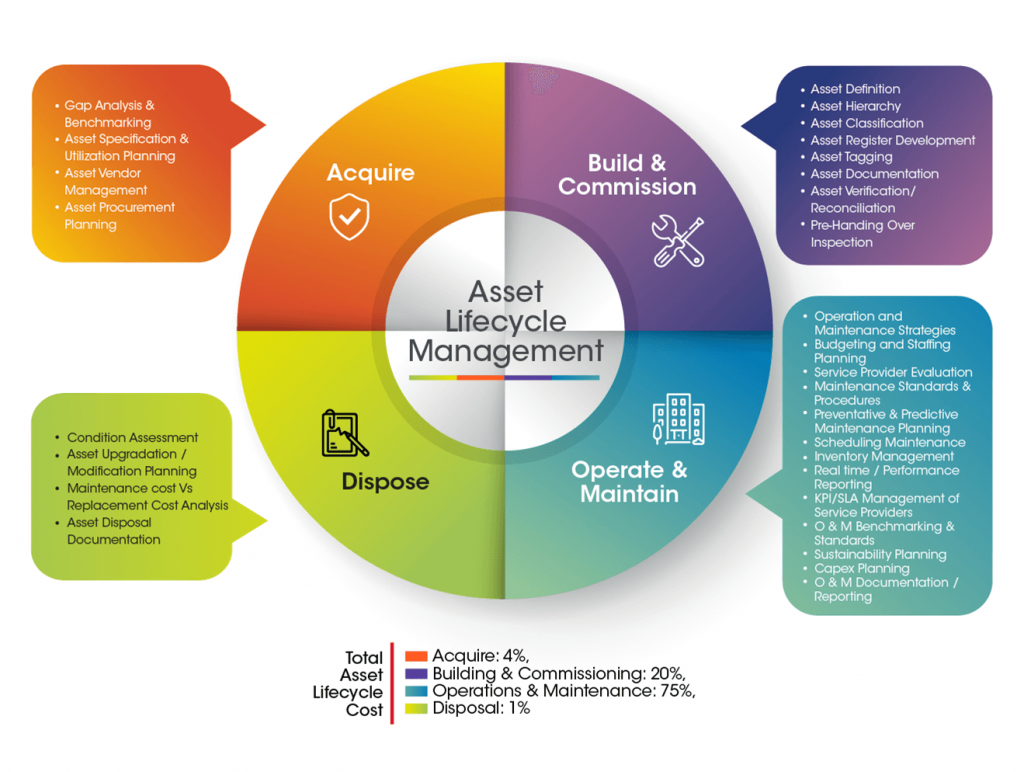
Asset Lifecycle Management by Aladdin
Background: BlackRock, the world’s largest asset manager, leverages its Aladdin platform to revolutionize its investment management process. Aladdin, powered by machine learning, integrates various data types, such as economic indicators, historical performance, and alternative datasets like satellite imagery and social media sentiment, to generate predictive insights that help optimize portfolio performance.
Capabilities: Aladdin provides BlackRock with a comprehensive view of the market by combining structured and unstructured data. This advanced analysis allows the platform to anticipate shifts before they fully materialize.
For example, Aladdin can analyze satellite data to estimate retail foot traffic, which helps predict consumer spending trends and gives BlackRock a competitive advantage in forecasting stock performance.
Results: The platform’s early predictive capabilities also enhance risk management by allowing BlackRock to identify and mitigate potential risks before they affect portfolio performance. Machine learning models in Aladdin continuously learn from real-time market conditions, enabling dynamic adjustments to portfolios. This proactive approach helps BlackRock reduce exposure to high-risk assets and pivot towards more stable investments, ensuring long-term portfolio stability.
Example 2: JPMorgan’s Use of Sentiment Analysis
Background: JPMorgan, one of the largest and most innovative financial institutions, utilizes machine learning-powered sentiment analysis to gain a deeper understanding of market sentiment and predict investor reactions. The bank’s sentiment analysis platform processes large volumes of data from news articles, social media posts, and financial reports to gauge the mood of the market.
Capabilities: By leveraging natural language processing (NLP), JPMorgan’s sentiment analysis model can classify news and social media sentiment as positive, negative, or neutral. This allows their trading teams to gain real-time insights into how current events may affect market dynamics.
For example, JPMorgan can quickly assess the impact of breaking news—such as changes in government policy or major corporate announcements—and adjust its trading strategies to capitalize on these developments.
Results: One of the key advantages of JPMorgan’s approach is its ability to identify nuanced shifts in market sentiment that may not be immediately apparent. By using historical data and context-based sentiment analysis, the model can determine how similar news events have affected markets in the past and use that information to make predictive adjustments. This proactive strategy enables JPMorgan to make informed trades ahead of competitors who rely solely on traditional analysis methods.
Future Trends: The Evolving Role of Machine Learning in Asset Management
Machine learning will continue to shape the future of asset management. One major trend is the rise of Explainable AI (XAI), which will make ML models easier to understand and more trustworthy. This will help meet regulatory demands and make ML accessible to more industry professionals.
Another trend is AI-driven ETFs, which use ML to manage asset allocations in real time. These funds can quickly adapt to market changes, offering better returns and risk management. As more firms adopt AI-driven products, we will see a new generation of investment tools that are both sophisticated and cost-effective.
Additionally, the blend of quantitative finance and ML will lead to hybrid strategies that improve on traditional models. By using ML to optimize parameters, asset managers can achieve more accurate predictions and enhanced returns, marking a new era for quantitative investment management.
How to Get Started with Machine Learning in Asset Management
Step 1. Develop a Data Strategy
Gather, clean, and validate large datasets to ensure high-quality inputs for ML models. Reliable data is the foundation for successful machine learning outcomes.
Step 2. Collaborate with Data Scientists
Partner with data scientists who have the technical expertise to build and refine models. Cross-functional teams are crucial to align ML with business goals and make it practical for real-world investment use.
Step 3. Set Up the Right Technology Stack
Invest in the right technology, including cloud infrastructure and tools like TensorFlow or Scikit-learn. Using cloud-based ML platforms helps scale operations without heavy upfront costs.
Step 4. Upskill Your Team
Provide training for managers and analysts to understand ML basics. Upskilling the team improves collaboration and enables them to effectively leverage ML models in their decision-making processes.
The Path Forward
Machine learning is transforming asset management by improving decision-making, operational efficiency, and risk management. Those who embrace these technologies will lead the industry into the future.
Now is the time to innovate. At SmartDev, we can help you harness the power of machine learning for asset management. Contact us today to learn how we can support your journey with cutting-edge solutions.
References: