In today’s fast-paced Fintech world, scams and fraud, especially in credit risk evaluation and lending, have been growing rapidly. Traditional credit scoring models often fail to identify high-risk borrowers, leaving lenders vulnerable to loan default risks and fraudulent applications. This is where predictive analytics, powered by artificial intelligence (AI), comes into play. By analyzing vast datasets and detecting patterns that traditional methods lack, predictive analytics offers a more secure, accurate way to assess borrower risk, mitigate fraud, and improve overall risk management. This article delves into the impact of AI in evaluating credit risk and provides insights on how financial institutions can implement these tools to stay competitive.
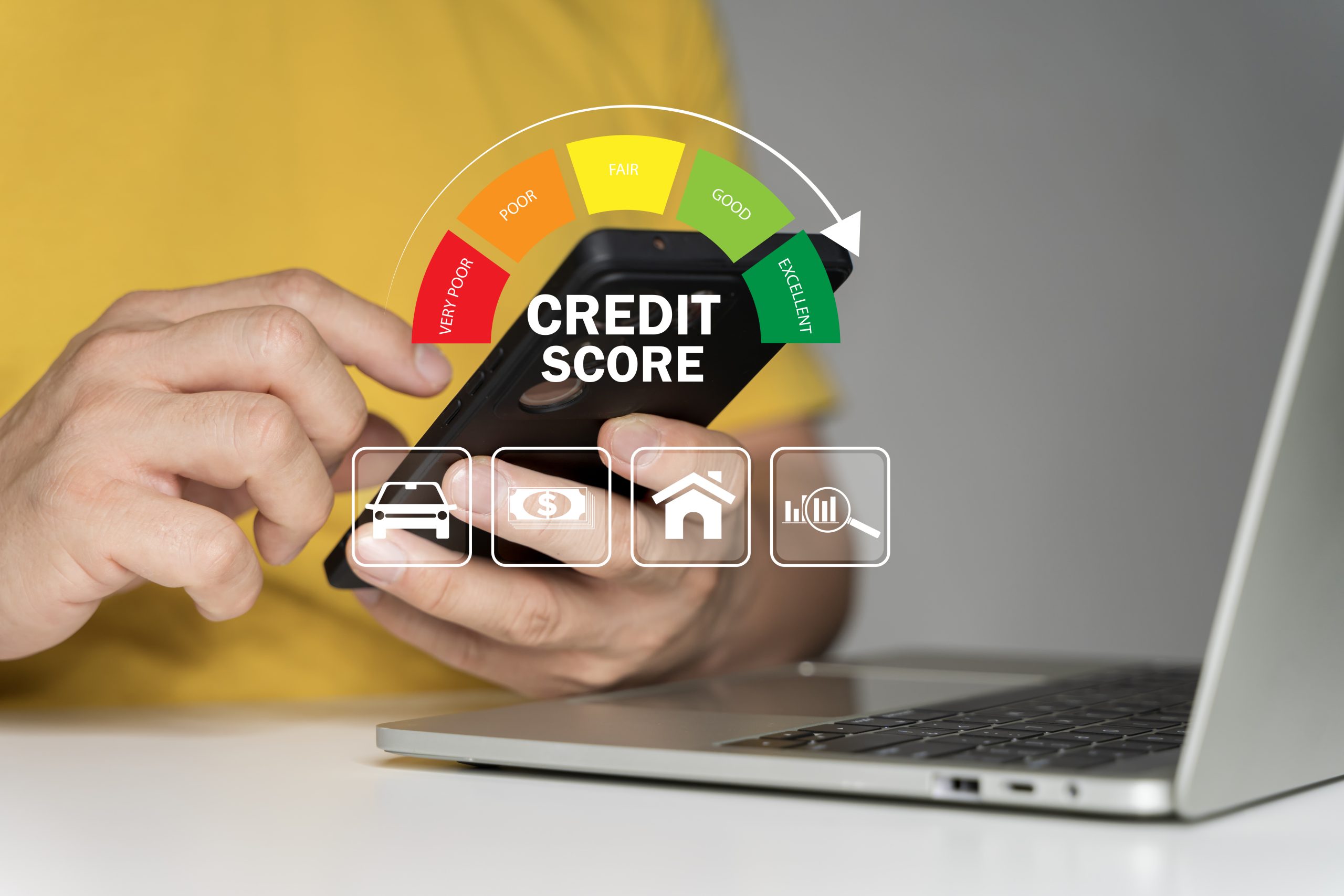
1. Understanding Predictive Analytics in Fintech
Understanding predictive analytics in fintech is essential for grasping how financial institutions assess credit risk and make informed lending decisions. Before diving into the subject, we must understand the initial development of credit evaluation activities to understand further context.
a. Historical Perspective
In the early stages of credit risk evaluation, financial institutions primarily focused on assessing a borrower’s creditworthiness through traditional metrics. Lenders would analyze factors to gauge the likelihood of legitimacy such as financial history, credit scores, income stability, and existing debt levels. However, this process often over-relies heavily on historical data and personal interviews, where lenders would evaluate the borrower’s character and financial behavior. The objective was to develop a comprehensive understanding of the borrower’s ability and willingness to repay loans. But this approach had various drawbacks at that point in time that hindered high risks such as:
- Lack of accuracy and transparency in forecasting
- Subjective judgment from the evaluator
- Time-consuming processes
- Out-dated data
But, thanks to the advent of big data and machine learning in the 21st century, credit risk evaluation has further revolutionized. Financial institutions began leveraging vast datasets and complex algorithms to analyze patterns and behaviors that traditional models could not capture. Today, the integration of alternative data sources—such as transaction histories and behavioral analytics—continues to enhance the precision and effectiveness of credit risk assessments.
b. Today’s Definition and Impact
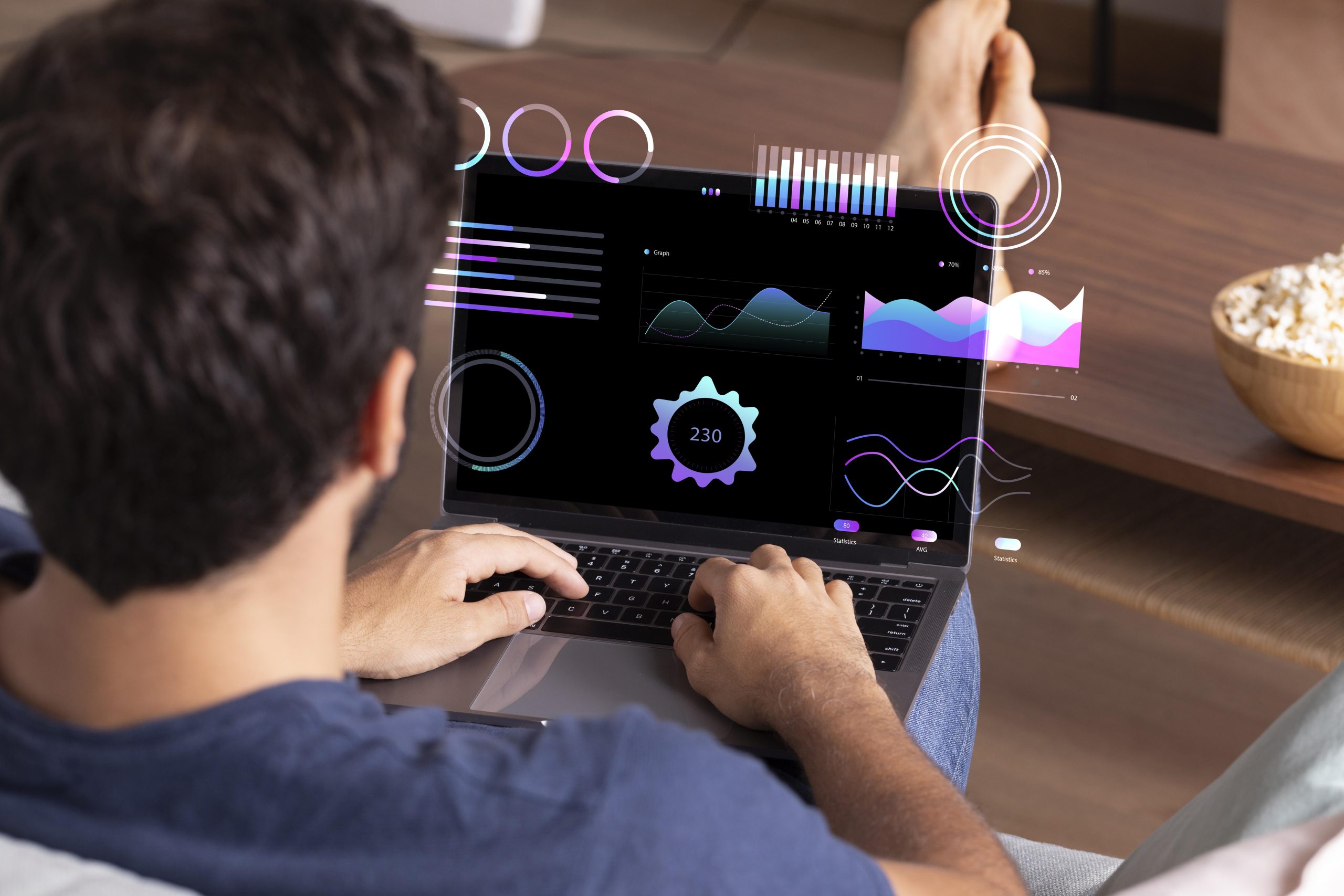
Subsequently, predictive analytics is a branch of advanced analytics that employs statistical techniques, machine learning algorithms, and data mining to forecast future outcomes based on historical and current data.
From an IT perspective, predictive analytics involves the integration of various data sources and sophisticated computational methods to analyze patterns and trends within large datasets. This process typically includes data collection, cleaning, modeling, and validation, enabling organizations to derive actionable insights from complex information. The technological advancements in computing power and data storage have made it possible for businesses to analyze vast amounts of data more efficiently than ever before.
From a Fintech perspective, predictive analytics plays a crucial role in assessing credit risk and enhancing decision-making processes related to lending. It enables financial institutions to identify potential risks associated with borrowers by analyzing diverse data points beyond traditional credit scores—such as transaction histories, social media activity, and even economic indicators. By leveraging predictive models, fintech companies can make informed decisions about loan approvals, interest rates, and risk management strategies while improving customer experiences through tailored offerings.
c. Key Roles in the Fintech Field
Overall, the fundamental benefits of predictive analytics in fintech are multifaceted and crucial for effective credit risk management:
- Enhance the accuracy of credit assessment
- Facilitate real-time decision-making
- Monitor and improve
- Personalize customer experiences
- Inform risk management strategies
2. AI’s Impact on Risk Management
a. Mechanisms of AI in Predictive Analytics
AI-powered predictive analytics has transformed risk management in Fintech. Its ability to process large datasets, detect patterns, and continuously improve has led to more accurate credit risk assessments. Here’s how AI mechanisms work:
- Machine Learning (ML): ML models learn from historical data and continuously improve, making them highly effective at predicting loan defaults by analyzing borrower credit scores and repayment history.
- Natural Language Processing (NLP): NLP helps AI systems analyze unstructured data like social media activity, which can reveal financial stress or changes in a borrower’s behavior.
- Neural Networks: These models excel at recognizing complex patterns, allowing them to predict a borrower’s risk by simultaneously analyzing various factors, including spending habits and income stability.
- Decision Trees and Random Forests: These models make predictions by splitting data into branches based on different factors like credit scores and income levels, helping lenders make more accurate decisions.
b. Case Studies: AI in Predictive Analytics for Risk Management
Upstart
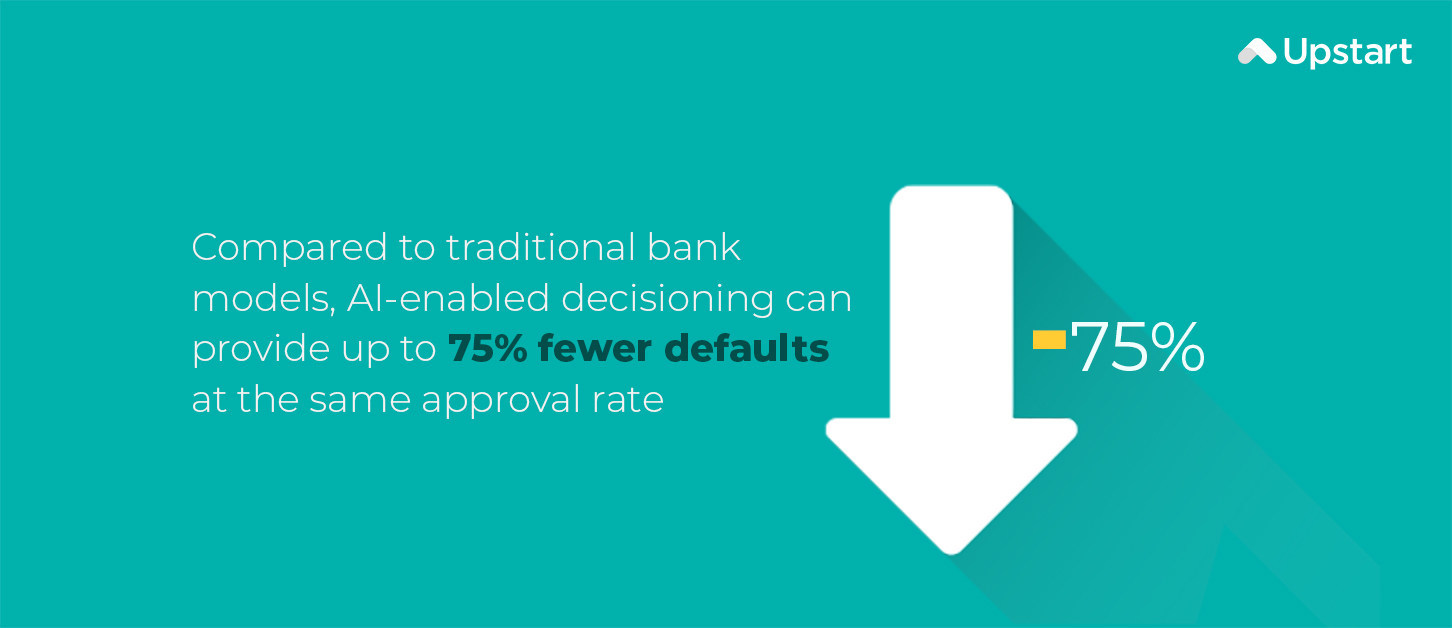
Problems
Upstart, a fintech company founded by former Google employees, recognized significant challenges in the traditional personal loan market. Traditional credit scoring models relied heavily on limited data, primarily credit scores, which often excluded many potential borrowers, particularly younger individuals and those with limited credit histories. This approach not only restricted access to credit for deserving applicants but also resulted in higher default rates for lenders, as they were unable to accurately assess the risk of these underserved populations.
Solutions
To address these challenges, Upstart implemented an AI-driven predictive analytics platform that utilizes machine learning algorithms to evaluate loan applicants more comprehensively. Instead of relying solely on credit scores, Upstart’s model incorporates a broader range of data points, including education, employment history, field of study, and even GPA. By analyzing these unconventional variables alongside traditional metrics, Upstart can create a detailed risk profile for each borrower.
This innovative approach has allowed Upstart to significantly improve its loan approval process. The company claims a 75% improvement in default prediction accuracy compared to traditional methods. As a result, Upstart can offer lower interest rates to borrowers while maintaining low default rates, thereby democratizing access to personal loans for a broader audience.
Zest AI
Problems
Zest AI, a fintech company specializing in machine learning solutions for credit risk assessment, faced significant challenges in the lending landscape. Traditional credit scoring methods often relied on limited data, such as credit scores and income levels, which failed to capture the full financial picture of borrowers. This resulted in high default rates and a lack of inclusivity in lending practices. Many potential borrowers, particularly those with thin credit files or non-traditional financial backgrounds, were unfairly denied loans, leading to lost opportunities for both the lender and the consumers.
Solutions
To address these challenges, Zest AI developed an AI-driven predictive analytics platform that analyzes thousands of variables about each borrower, including alternative data such as rent payments, utility bills, and transaction histories. By employing machine learning algorithms, Zest AI’s models can identify patterns and predict borrower behavior more accurately than traditional methods.
One notable case involved a large U.S. auto lender that partnered with Zest AI to implement these predictive models. The lender utilized Zest AI’s technology to enhance its underwriting process, allowing for more inclusive lending decisions while effectively managing risk. As a result, the lender was able to make data-driven decisions that not only reduced default rates but also increased loan approvals.
Key Learning
- Comprehensive Data Utilization: Both Zest AI and Upstart demonstrate the importance of leveraging diverse data sources beyond traditional credit metrics. By incorporating alternative data points—such as rent payments, utility bills, education, and employment history—lenders can develop a more nuanced understanding of borrower risk profiles.
- Increased Access to Credit: The innovative approaches taken by these fintech firms enable them to extend credit to underserved populations who may have been excluded by traditional lending practices. This inclusivity not only benefits borrowers but also opens new revenue streams for lenders.
- Measurable Outcomes: Both companies achieved impressive results through their predictive analytics implementations. Zest AI reported a 15% reduction in default rates and a 30% increase in loan approvals, while Upstart noted a 75% improvement in default prediction accuracy. These metrics highlight the tangible benefits of using AI-driven solutions in lending.
- Proactive Risk Management: Predictive analytics allows lenders to identify potential risks early in the application process, enabling proactive measures to mitigate defaults before they occur. This capability is crucial for maintaining financial health and stability.
- Scalability and Continuous Improvement: The machine learning models used by both Zest AI and Upstart are designed to evolve continuously with new data inputs. This adaptability ensures that their predictive models remain accurate and relevant over time, allowing the companies to stay competitive in the dynamic fintech landscape.
- Enhanced Customer Engagement: By utilizing predictive analytics, both firms can engage with customers proactively, offering tailored financial products and support based on individual risk profiles. This personalized approach fosters stronger relationships and improves overall customer satisfaction.
In summary, the cases of Zest AI and Upstart illustrate how predictive analytics can transform credit risk management within fintech firms. By adopting innovative solutions that leverage comprehensive data, financial institutions can improve risk assessment, enhance customer experiences, and drive business growth in an increasingly competitive environment.
3. Implementing AI Predictive Analytics
To implement AI-powered predictive analytics, financial institutions must first build a solid data infrastructure by gathering and organizing both traditional and alternative data, such as credit scores and transaction records. Ensuring data is well-structured and securely stored is critical for successful analysis.
The next step is developing AI models tailored to specific needs, such as predicting loan defaults or managing credit risk. Models like decision trees and neural networks can be trained using historical data and updated regularly to stay relevant to changing trends.
Integration is key—these AI models must seamlessly connect with existing systems like loan origination and risk management platforms to provide real-time insights. Ongoing monitoring is essential to maintain model accuracy, and ensuring compliance with data privacy regulations like GDPR is critical to protect sensitive financial information. By following this approach, institutions can effectively use AI to enhance credit risk assessment and financial management strategies.
4. Advice for Managers
Financial managers looking to implement AI-driven predictive analytics should start by ensuring their organization has a solid data infrastructure. This includes collecting and managing high-quality, comprehensive data, both traditional and non-traditional, to feed AI models. Investing in the right talent, such as data scientists and IT professionals, is essential for developing and maintaining these models. If expertise is lacking, partnering with Fintech or ITO firms specializing in AI can be highly beneficial.
Begin with small, focused projects, like using AI to assess loan default risks, and scale gradually as success is demonstrated. Transparency and regulatory compliance are critical, especially in how AI-driven decisions are communicated to customers. It’s also important to continuously monitor and update AI models to ensure they remain accurate and relevant over time. By taking a strategic and phased approach, financial managers can reduce risk and enhance decision-making effectively.
5. What’s Next?
As we look to the future, the application of AI predictive analysis tools in the financial sector will undoubtedly continue to evolve, providing innovative solutions to mitigate credit risks. Financial institutions must not only adopt these technologies but also strategically integrate them into their risk management frameworks. By leveraging advanced predictive analytics, businesses can enhance their decision-making processes, minimize defaults, and ultimately secure a competitive edge in the market.
At SmartDev, we recognize the critical role that robust AI solutions play in this transformation. Our team of experts is dedicated to delivering tailored services that meet the unique needs of our financial clients. We specialize in developing and implementing cutting-edge AI predictive analysis tools designed to enhance credit risk assessment, streamline operations, and improve overall financial health. Our services include:
- Custom AI Solutions: Tailored predictive analytics tools that fit your specific business needs.
- Risk Assessment Models: Advanced models that improve accuracy in evaluating credit risk.
- Data Integration Services: Seamless integration of various data sources for comprehensive analysis.
- Consultation and Support: Expert guidance throughout the implementation process.
- Training and Workshops: Educational sessions to empower your team in utilizing AI tools effectively.
Let us partner with you in this journey toward a more secure financial future. Together, we can unlock the full potential of AI predictive analysis to transform your credit risk management strategies and propel your business to new heights. Contact SmartDev today to explore how our services can benefit your organization and position you at the forefront of the financial technology revolution.
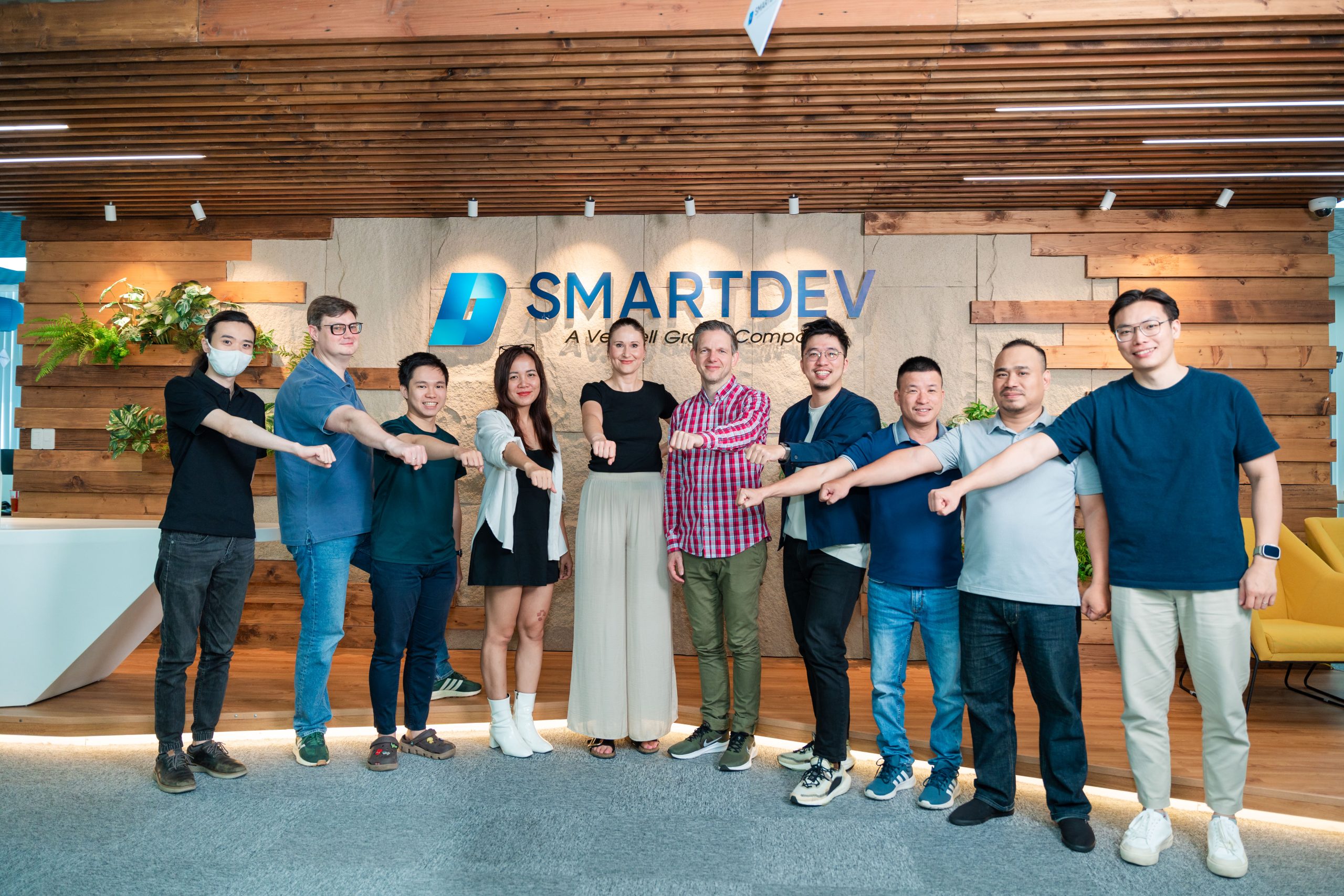