Einführung in die KI-Anomalieerkennung
AI-powered anomaly detection identifies unusual data patterns, improving accuracy over traditional methods. AI for anomaly detection adapts to complex, evolving data, addressing system limitations.
Applied in industries like finance, healthcare, and cybersecurity, anomaly detection AI helps detect fraud, diagnose early, and optimize operations, offering precise, real-time insights.
Was ist Anomalieerkennung?
Anomaly detection is the process of identifying patterns or behaviors in data that deviate significantly from expected norms. This technique is commonly applied across various domains, including fraud detection, network security, and manufacturing, to spot unusual events or trends that may indicate potential issues.
AI anomaly detection is increasingly used to improve the precision of identifying outliers in vast datasets. According to IBM’s guide on anomaly detection, it is crucial for enhancing predictive maintenance and optimizing operational efficiency in industries such as healthcare and finance.
Die Rolle der KI bei der Anomalieerkennung
Artificial Intelligence (AI) enhances anomaly detection by enabling systems to learn from vast amounts of data and identify complex, hidden patterns. Traditional methods may struggle with large datasets or evolving anomalies, but AI’s ability to adapt to new information allows for more accurate and dynamic detection.
Research from MIT emphasizes AI’s role in advancing anomaly detection by providing deeper insights and real-time responses, particularly when combined with machine learning models for anomaly detection. With AI, industries can improve the detection of previously undetected irregularities.
Warum traditionelle Methoden nicht ausreichen
Traditional anomaly detection methods, such as rule-based systems, often lack the flexibility and scalability required in today’s data-driven world. These methods are limited in detecting subtle anomalies or those that evolve over time.
According to Gartner’s research, while traditional methods may suffice for simpler tasks, AI anomaly detection’s adaptive nature outperforms them in detecting increasingly complex and dynamic patterns in modern datasets. The rise of AI allows businesses to address these gaps, offering far more precision in handling high-dimensional data and variable data trends.
Wichtige Anwendungen und Branchen
AI-powered anomaly detection has found applications in numerous industries, including healthcare, finance, cybersecurity, and manufacturing.
For instance, AI can help identify potential fraud in financial transactions or detect unusual patterns in medical data, which are crucial for early diagnosis and intervention.
Forbes outlines how industries are leveraging anomaly detection AI to improve operational efficiency and enhance security by identifying issues before they escalate. With AI, organizations can not only spot fraud but also detect anomalies in network traffic and predict equipment failures, thereby saving costs and improving system reliability.
Anomalien verstehen: Arten und Herausforderungen
AI-powered anomaly detection is essential for identifying irregular patterns in data that could indicate significant issues. With the rise of anomaly detection AI, detecting these outliers has become more efficient and accurate, allowing businesses to act proactively.
However, detecting anomalies involves understanding different types and overcoming challenges such as data imbalance and real-time processing needs.
This section explores the different types of anomalies and the key challenges that come with detecting them.
Was definiert eine Anomalie?
An anomaly refers to a data point or pattern that significantly deviates from the expected behavior or distribution. These outliers can indicate rare or significant events, such as fraudulent activities, system malfunctions, or unforeseen market shifts.
According to Data Science Central, anomalies play a vital role in identifying potential risks or opportunities across various domains. AI anomaly detection enables the efficient identification of such deviations, providing actionable insights in real-time.
Arten von Anomalien
Not all anomalies are created equal—and knowing the difference matters. In this section, you’ll explore the three key types of anomalies: point anomalies, which are single outliers that don’t fit the pattern; contextual anomalies, which depend on timing or circumstances (think unusually high web traffic at 3 a.m.); and collective anomalies, where a group of normal-looking data points combine to signal something unusual.
Grasping these distinctions helps you build smarter, more reliable AI systems that catch what really matters.
Up next, we’ll break down real-world challenges in anomaly detection—and how to overcome them. Keep reading to sharpen your edge.
Punktanomalien
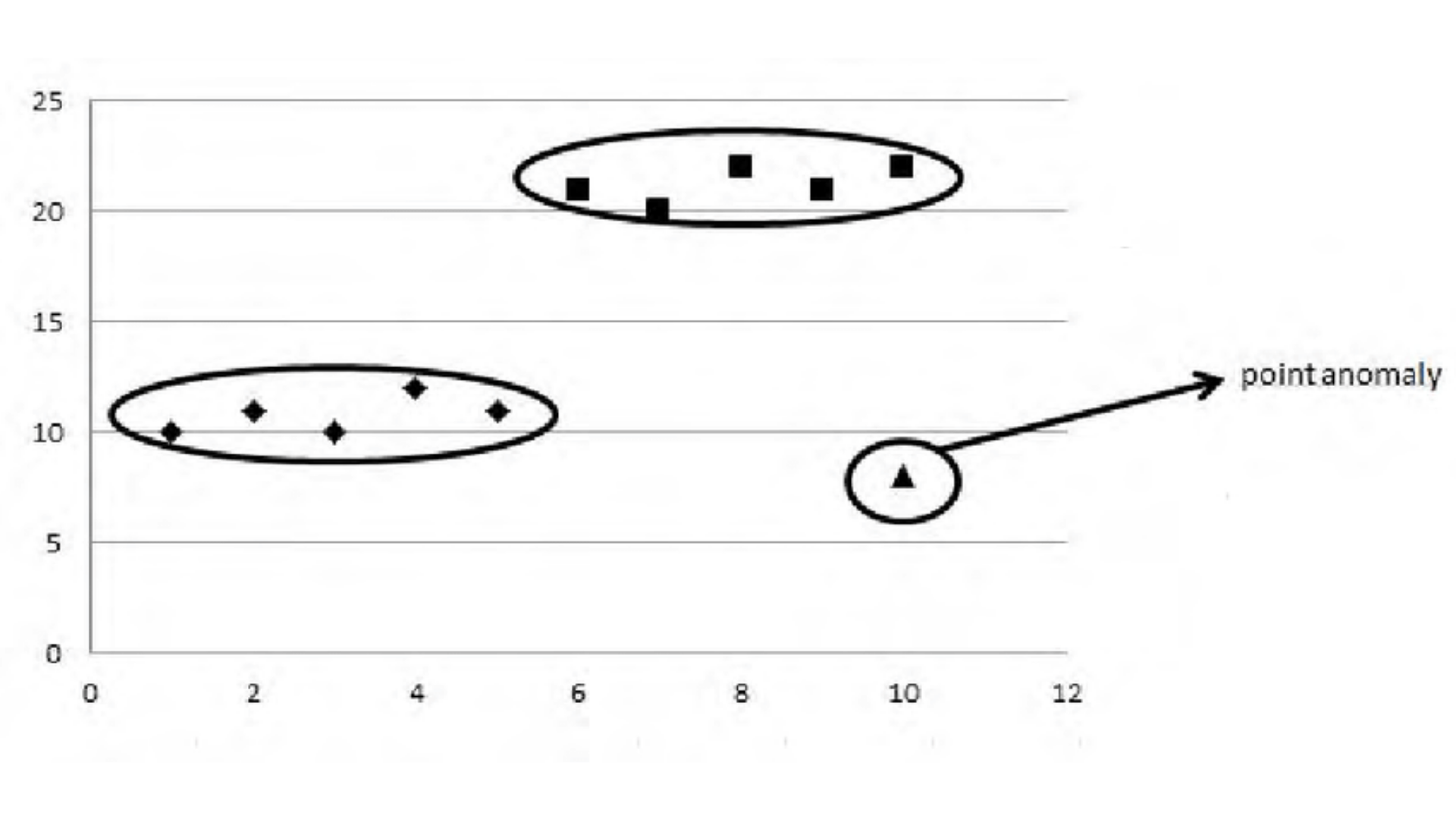
Quelle: Ein Beispiel für eine Punktanomalie – ResearchGate
Point anomalies occur when a single data point deviates significantly from the norm. These anomalies are easy to detect when the data is well-defined and isolated.
As noted in KDnuggets, point anomalies are the simplest form of anomaly and are commonly observed in fraud detection or quality control, especially with the use of AI for anomaly detection.
Kontextuelle Anomalien
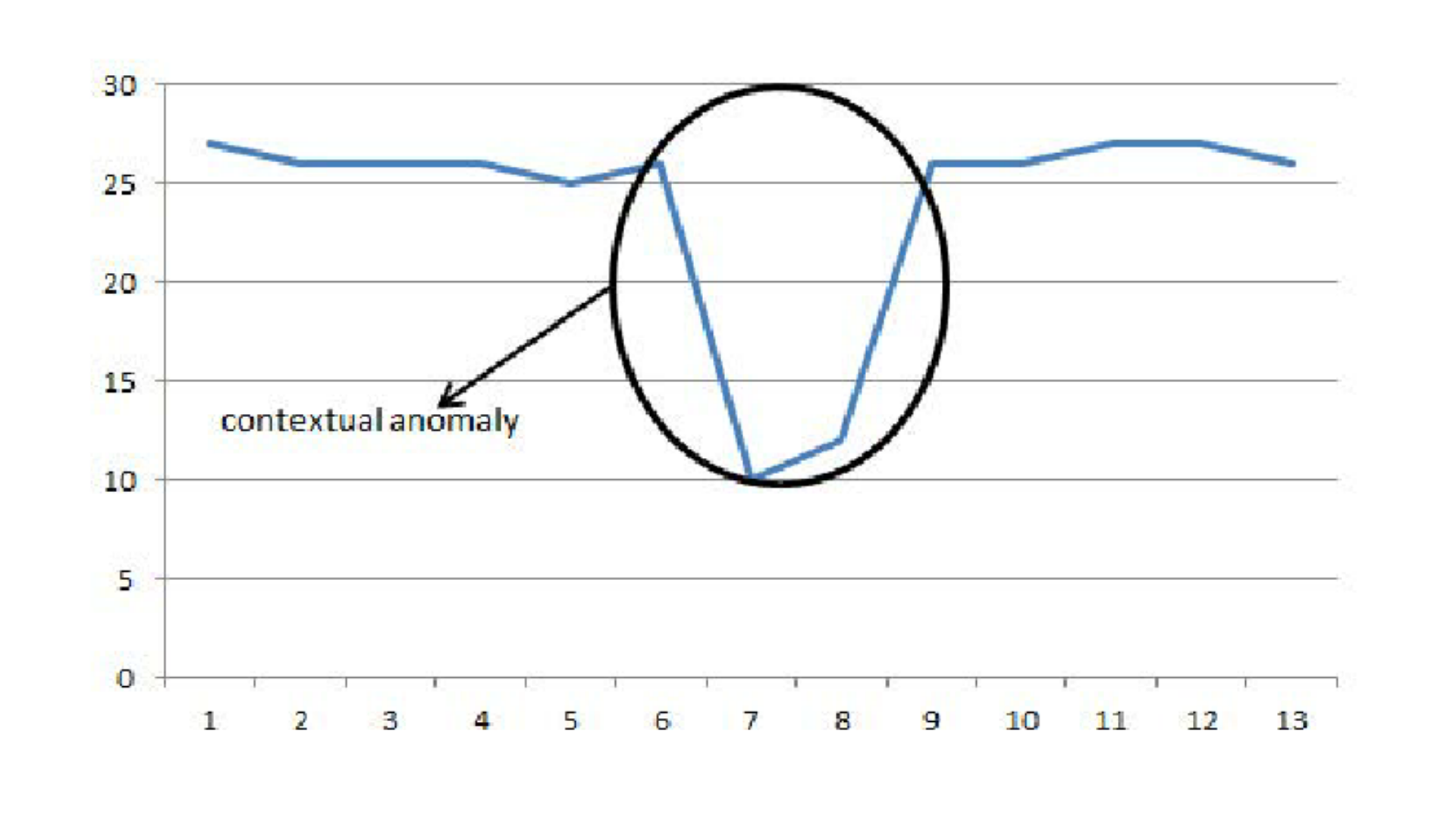
Quelle: Ein Beispiel für eine Kontextanomalie – ResearchGate
Contextual anomalies are data points that may be normal in one context but abnormal in another. For example, an unusually high temperature might be normal during summer but abnormal in winter.
According to Towards Data Science, contextual anomalies are often harder to detect because they depend on the context in which the data occurs. Anomaly detection AI can address these complexities by analyzing the context and adapting accordingly.
Kollektive Anomalien
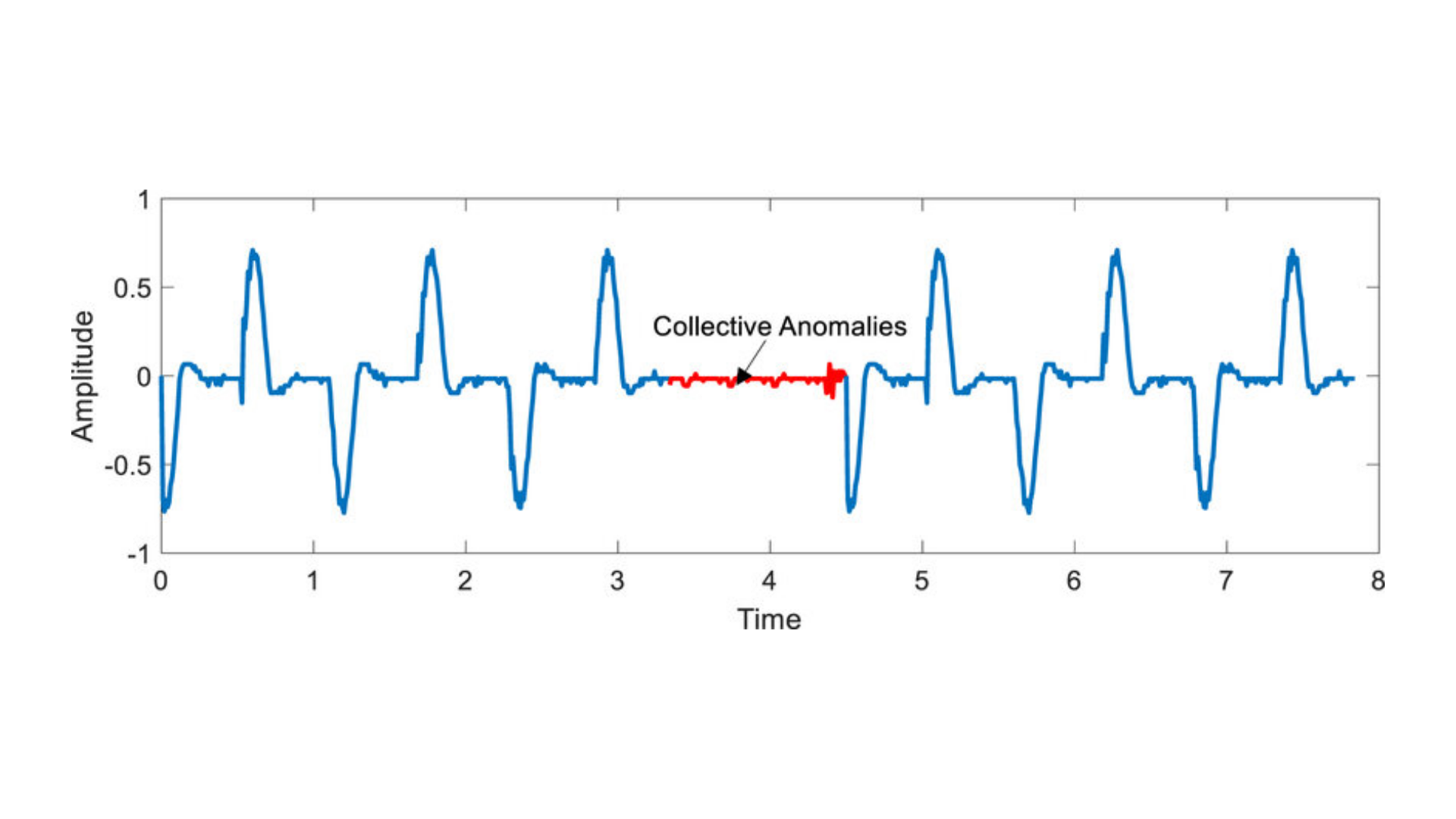
Quelle: Ein Beispiel für kollektive Anomalie – ResearchGate
Collective anomalies refer to a group of data points that, when considered together, deviate from the expected pattern, although individual points may not be anomalies on their own. This type of anomaly is often found in time-series data, where the sequence of events is more important than individual occurrences.
SpringerLink explains how collective anomalies are crucial for detecting trends in areas like cyber-attack recognition and fraud detection, which are enhanced by AI anomaly detection systems.
Herausforderungen bei der Erkennung von Anomalien
Spotting anomalies isn’t always easy—and AI systems face some serious hurdles. First, there’s data imbalance: anomalies are rare, which makes them harder to detect accurately. Then comes high dimensionality, where too many variables can cloud the picture and confuse even advanced models. Finally, the pressure of real-time detection means your system has to act fast—no second chances.
These challenges can derail even the smartest AI if not handled right.
Coming up next: Discover the AI techniques that overcome these obstacles and power next-level anomaly detection. Let’s dive in.
Datenungleichgewicht
One significant challenge in anomaly detection is data imbalance, where anomalies are rare compared to normal instances, making them difficult to identify. As pointed out by Microsoft’s Azure blog, this imbalance can result in high false positive rates or missed anomalies, especially in sensitive applications like cybersecurity.
AI models trained for anomaly detection AI can help mitigate this imbalance by learning from both normal and abnormal data effectively.
Hohe Dimensionalität
High dimensionality, or the presence of many features in a dataset, complicates the detection of anomalies due to the “curse of dimensionality.” As noted in SpringerLink, the higher the number of dimensions, the more challenging it becomes to define what constitutes an anomaly, leading to potential inaccuracies in detection.
Advanced AI for anomaly detection algorithms can effectively handle these challenges, improving the accuracy of detecting subtle anomalies in high-dimensional datasets.
Anforderungen an die Echtzeiterkennung
Many applications require real-time anomaly detection, which introduces complexities in model performance, scalability, and efficiency. The need to process and analyze data in real-time adds pressure to anomaly detection systems, particularly in environments like financial transactions or network security.
TechRadar discusses how real-time detection places a significant burden on systems, requiring robust AI-driven anomaly detection solutions capable of fast processing and decision-making.
Wie KI die Anomalieerkennung unterstützt
AI-powered anomaly detection is revolutionizing how businesses identify and address irregularities in their data. By leveraging AI anomaly detection, machine learning models adapt to new patterns, providing more flexibility and precision compared to traditional rule-based systems.
With the rise of deep learning and various learning approaches, AI is making anomaly detection more scalable and efficient, especially in complex, high-dimensional datasets.
This section explores the key differences in techniques and the role of deep learning in advancing anomaly detection.
Maschinelles Lernen vs. regelbasierte Anomalieerkennung
Machine learning-based anomaly detection relies on algorithms that can learn from data and adapt over time to recognize patterns, whereas rule-based systems follow predefined criteria to flag anomalies.
While rule-based systems are simple and effective for well-defined problems, they are limited when it comes to handling large or complex datasets. AI anomaly detection models, especially those based on machine learning, offer the advantage of adapting to new data patterns without requiring manual updates.
Entsprechend arXivMaschinelles Lernen bietet mehr Flexibilität und Skalierbarkeit und eignet sich daher besser für dynamische Umgebungen, in denen sich die Art der Anomalien im Laufe der Zeit ändert.
Überwachte, unüberwachte und halbüberwachte Lernansätze
AI-powered anomaly detection can be categorized into three main approaches: supervised, unsupervised, and semi-supervised learning.
In supervised learning, the model is trained on labeled data, allowing it to identify anomalies based on prior knowledge.
Unsupervised learning, on the other hand, does not require labeled data and relies on detecting patterns in data that differ significantly from the rest.
Semi-supervised learning combines both approaches, using a small set of labeled data and a larger set of unlabeled data. A study on arXiv highlights the growing importance of unsupervised and semi-supervised methods in anomaly detection AI, particularly in scenarios where labeled data is scarce or unavailable.
Die Rolle von Deep Learning bei der erweiterten Anomalieerkennung
Deep learning has become increasingly important in advanced anomaly detection due to its ability to process complex, high-dimensional data and identify patterns that traditional methods may miss.
Deep learning models, such as autoencoders and recurrent neural networks (RNNs), are particularly effective in detecting anomalies in time-series data and unstructured data such as images or text.
Research from arXiv demonstrates that deep learning models can significantly outperform traditional methods, especially in detecting subtle and evolving anomalies across diverse domains like cybersecurity and fraud detection. With the rise of AI anomaly detection, these models are reshaping industries by providing more accurate, real-time insights.
Kerntechniken und Algorithmen
AI anomaly detection utilizes various techniques and algorithms to identify unusual patterns in data. By combining statistical methods, machine learning models, and deep learning approaches, AI is making anomaly detection more accurate and scalable. Hybrid models that integrate different techniques offer enhanced performance in complex scenarios.
This section explores the core techniques used in AI anomaly detection, along with their strengths and applications.
Statistische Methoden
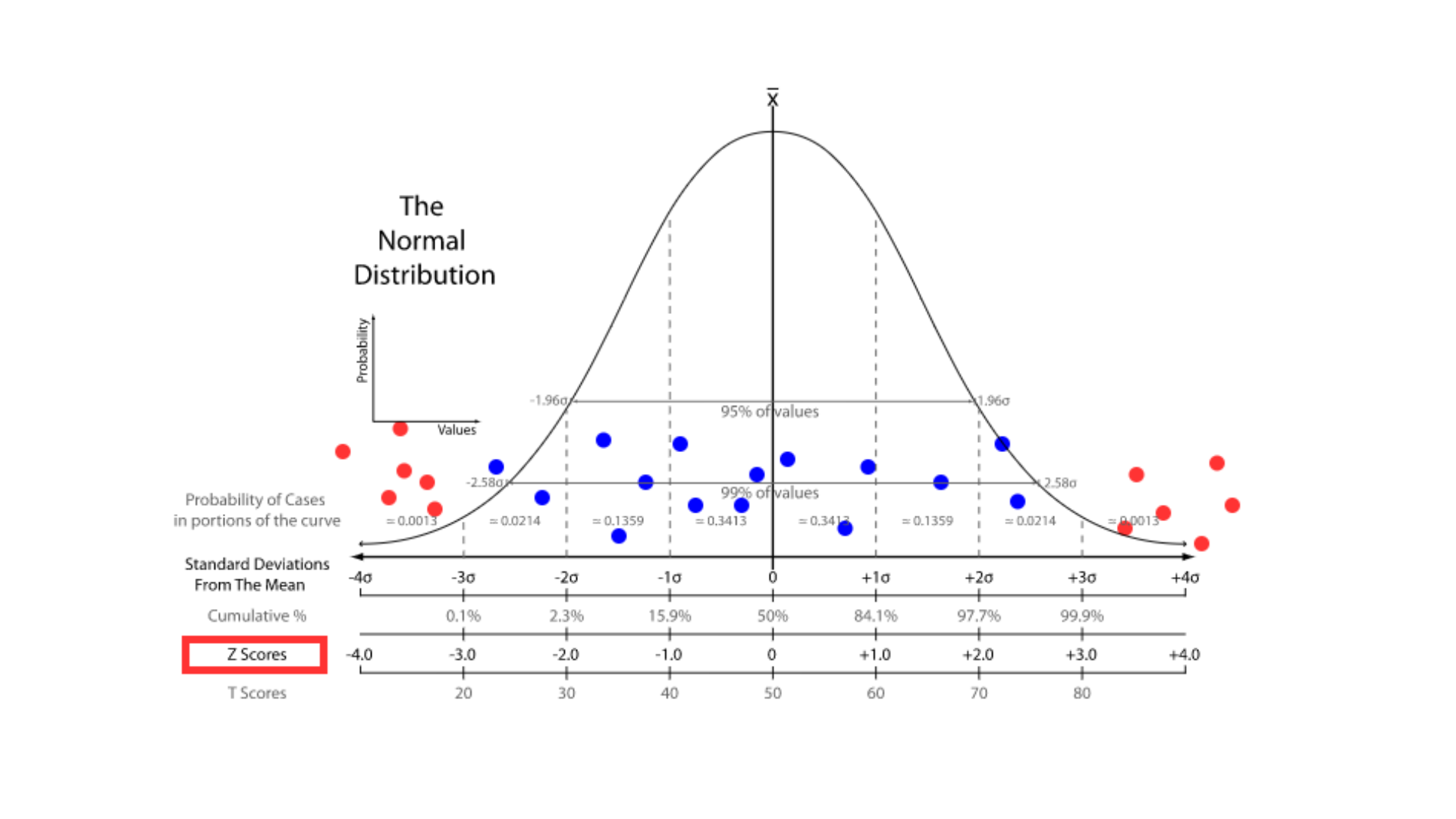
Quelle: Anomalieerkennung mit Z-Score: Pick The Low Hanging Fruits – Mittel
Statistical methods for anomaly detection are based on the assumption that normal data follows a specific statistical distribution. Anomalies are detected when data points significantly deviate from this distribution. Common techniques include z-scores, Gaussian mixture models, and hypothesis testing.
According to a study in arXiv, statistical methods are useful for simpler applications but can struggle with high-dimensional or non-linear data, limiting their effectiveness in more complex scenarios where anomaly detection AI is needed for higher accuracy.
Modelle für maschinelles Lernen
Isolationswald
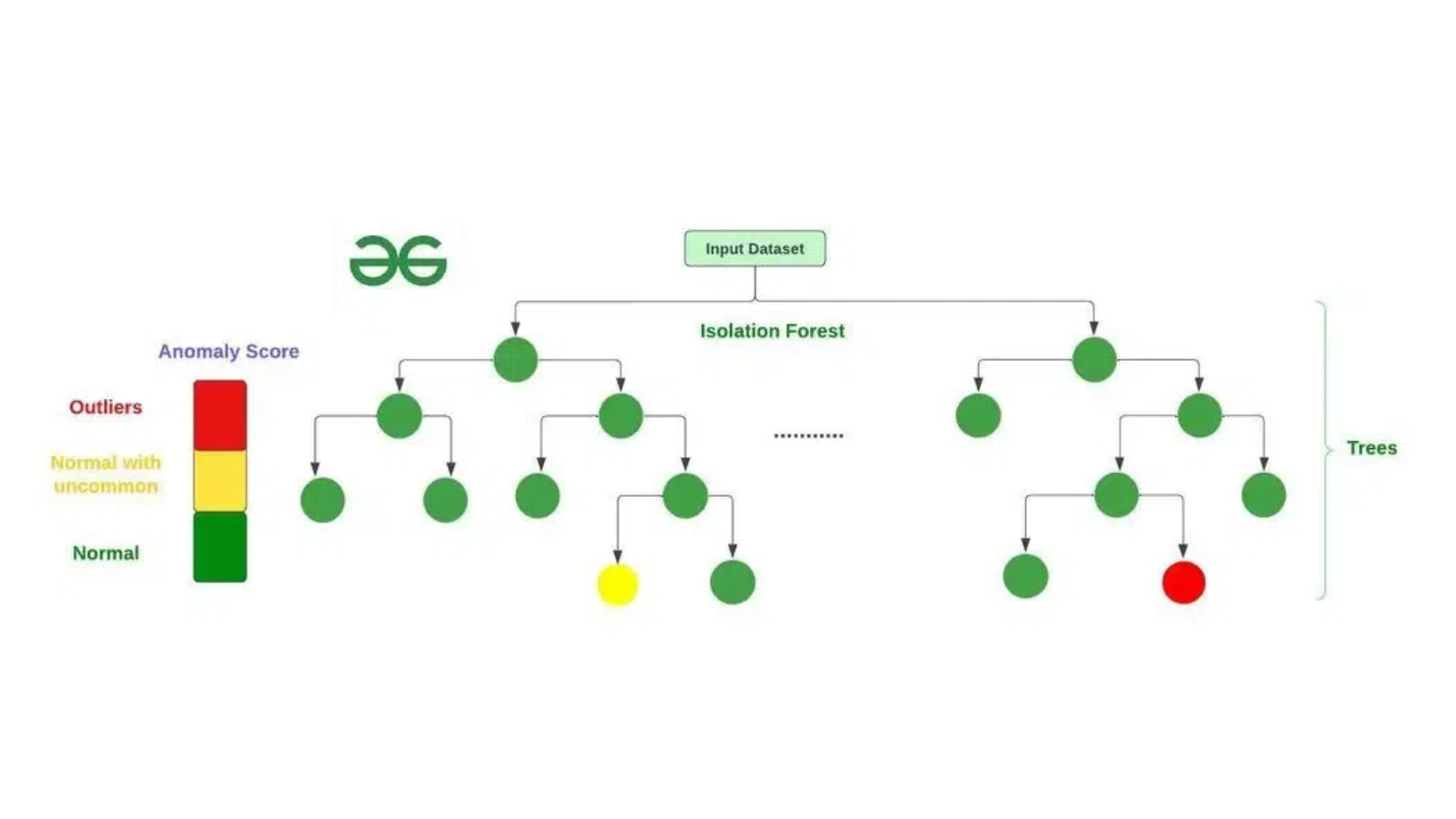
Quelle: Was ist Isolation Forest? – GeeksforGeeks
Isolation Forest is a machine learning model that isolates anomalies rather than profiling normal data points. It is particularly effective for high-dimensional datasets, as it constructs decision trees to isolate data points that are far from the norm.
Research from arXiv demonstrates the efficiency of Isolation Forest in anomaly detection tasks involving large datasets and sparse features, making it a powerful tool for AI for anomaly detection.
Ein-Klassen-SVM
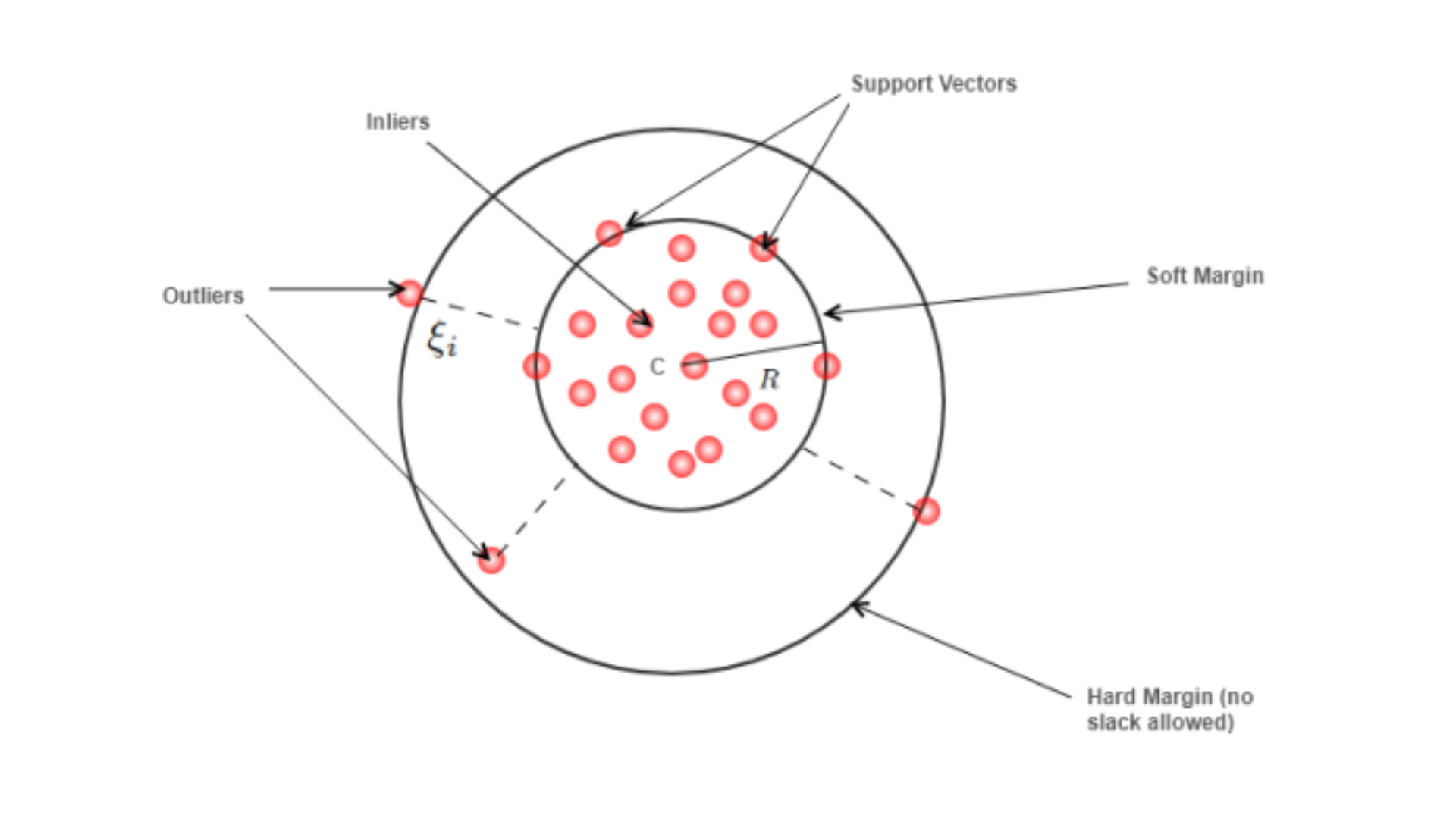
Quelle: One Class SVM (OC-SVM) – Mittel
One-Class Support Vector Machine (SVM) is a variant of the standard SVM algorithm that is trained to recognize only the normal class of data. It creates a boundary around normal data and flags anything outside this boundary as an anomaly.
Studies in SpringerLink show that One-Class SVM is effective in situations where labeled data is limited and anomaly patterns are less obvious, particularly in fraud detection and anomaly detection AI.
K-Means-Clustering
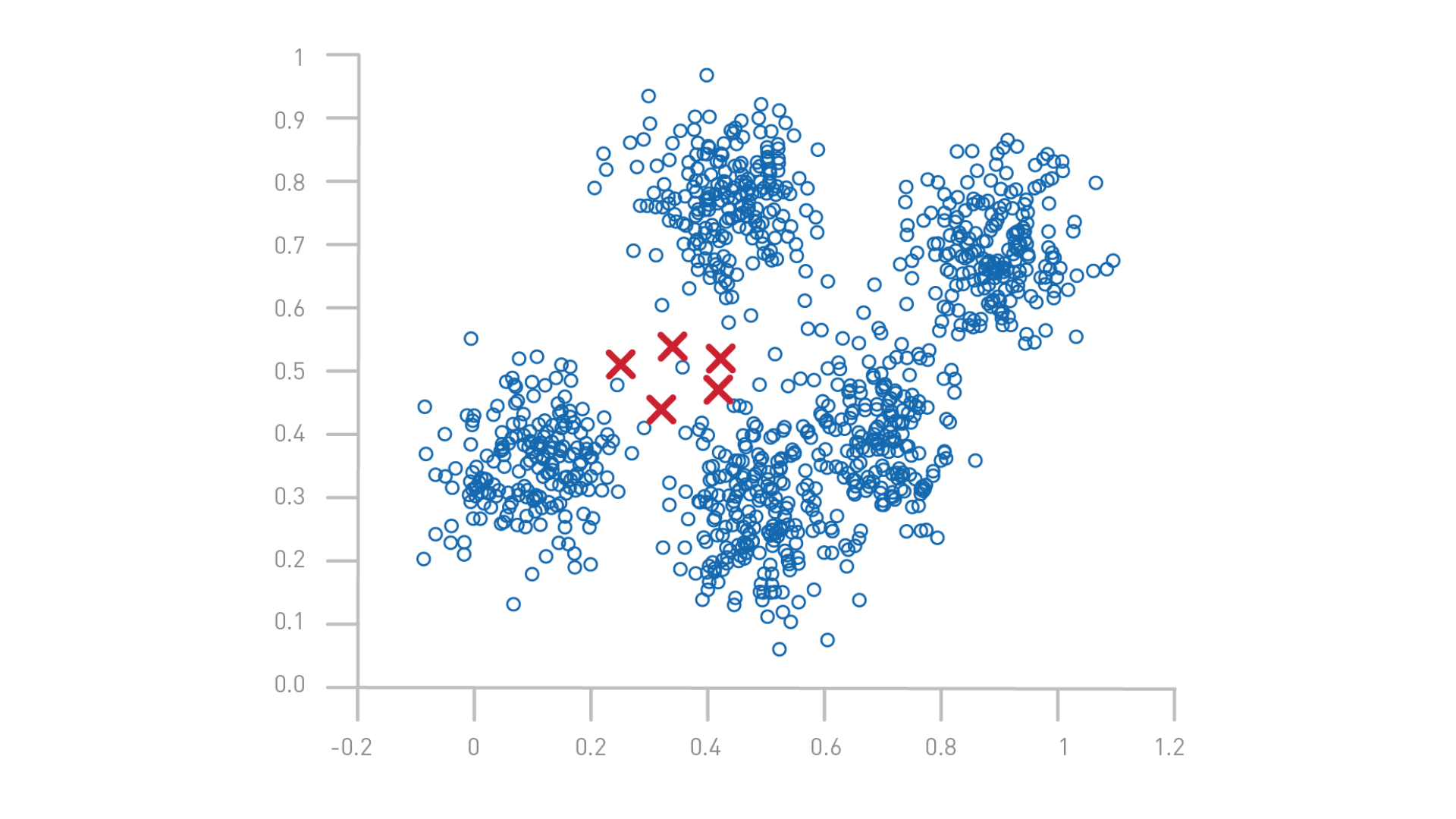
Quelle: K-Means-Clustering-Algorithmus – NVDIA
K-Means clustering is an unsupervised machine learning algorithm that groups similar data points together. In anomaly detection, points that do not belong to any cluster or are far from the centroid of their cluster are identified as anomalies.
According to a study on arXiv, K-Means is useful for applications with clear groupings in the data but may struggle with complex or overlapping clusters. It is commonly used in anomaly detection AI for clustering tasks in data mining.
Autoencoder
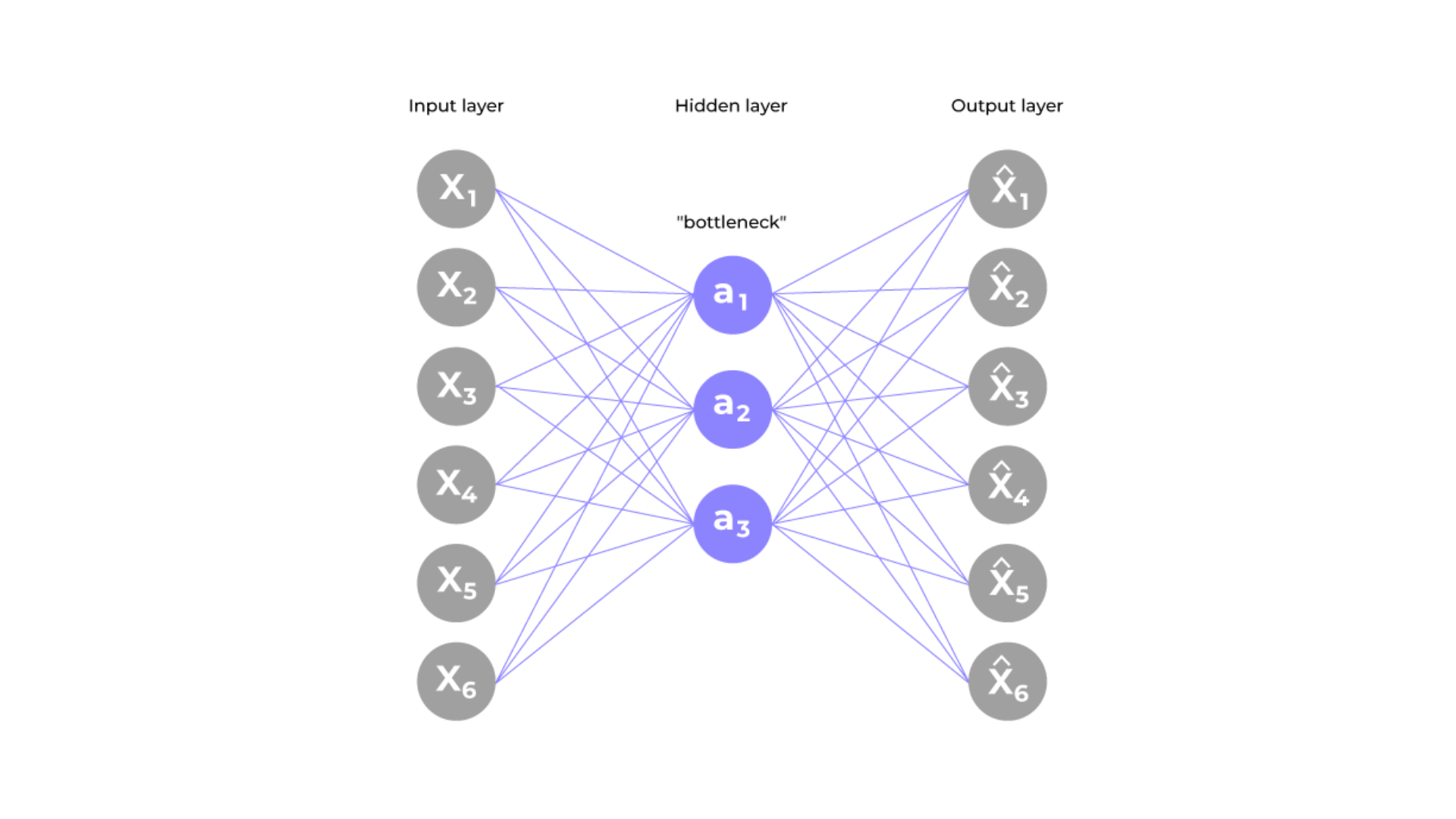
Quelle: Autoencoder im maschinellen Lernen – GeeksforGeeks
Autoencoders are neural network architectures used for unsupervised learning. They compress input data into a lower-dimensional representation and then reconstruct it. Anomalies are detected by measuring the reconstruction error, with high errors indicating potential anomalies.
Research from arXiv highlights the effectiveness of autoencoders in detecting anomalies in high-dimensional data such as images and sensor data, making them invaluable for AI anomaly detection in complex datasets.
Deep Learning-Ansätze
LSTMs zur Erkennung von Zeitreihenanomalien

Quelle: Das Framework des LSTM-basierten Ansatzes zur Anomalieerkennung – ResearchGate
Long Short-Term Memory networks (LSTMs) are a type of recurrent neural network (RNN) designed to handle sequential data, making them ideal for time-series anomaly detection. LSTMs are capable of learning long-term dependencies and detecting temporal anomalies that would be difficult for traditional methods.
A study in arXiv emphasizes LSTMs’ strong performance in applications such as stock market analysis and predictive maintenance, demonstrating the potential of AI for anomaly detection in dynamic data environments.
GANs zur synthetischen Anomalieerkennung
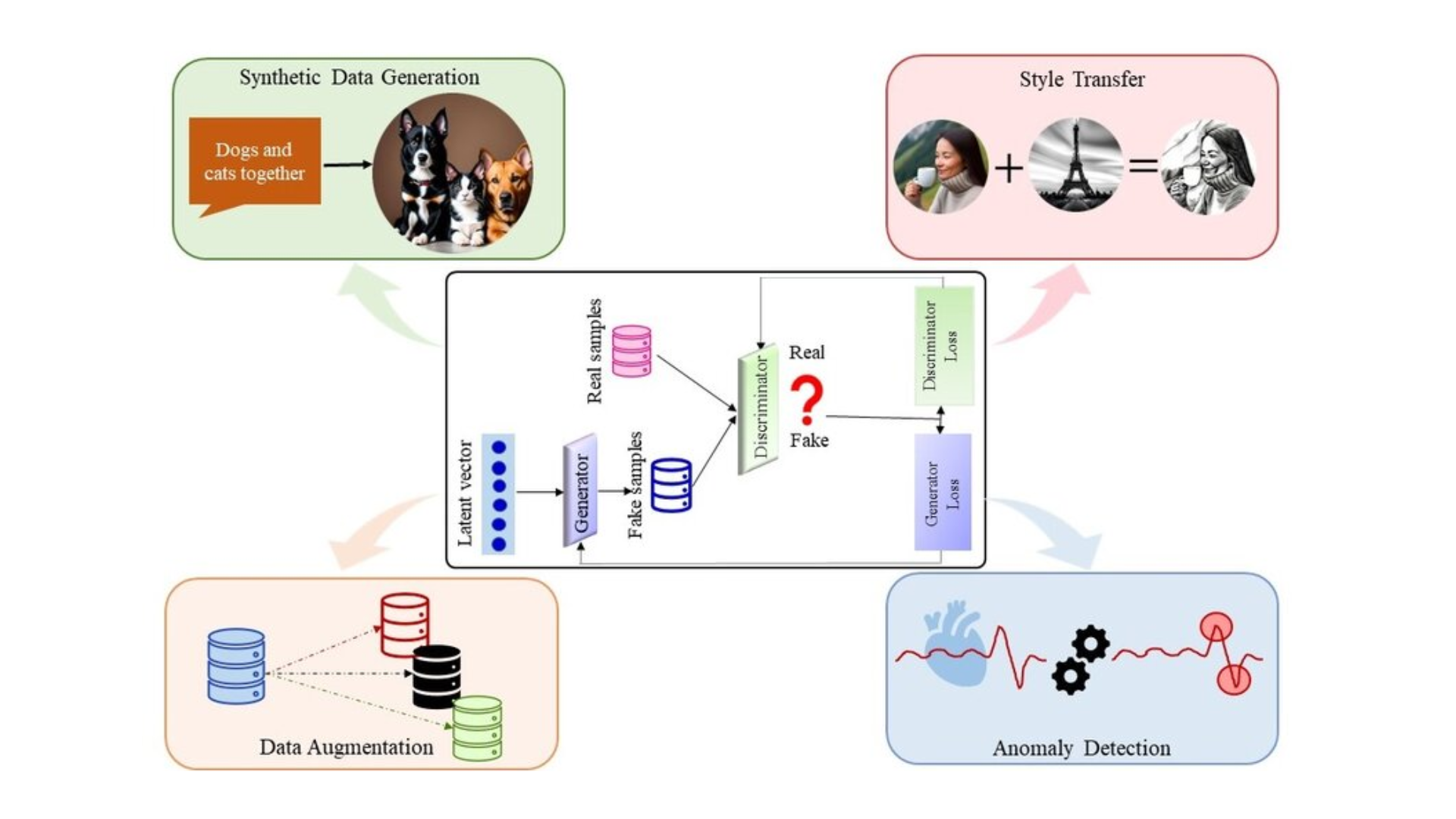
Quelle: Architektur von GANs und ihre Hauptfunktionen – ResearchGate
Generative Adversarial Networks (GANs) are used in synthetic anomaly detection by generating realistic data distributions and detecting anomalies through the discriminator network. GANs are particularly useful in generating synthetic data for training anomaly detection models when real data is scarce or difficult to obtain.
According to research in arXiv, GANs have shown promising results in anomaly detection tasks, especially in industries like cybersecurity and fraud detection, where anomaly detection AI is increasingly important.
Hybridmodelle für verbesserte Genauigkeit
Hybrid models combine multiple algorithms to leverage the strengths of different techniques and improve detection accuracy.
For instance, combining machine learning models with statistical methods or deep learning approaches can provide more robust and accurate anomaly detection, particularly in complex datasets.
Research from SpringerLink shows that hybrid models often outperform single-model approaches by improving generalization and reducing false positives, making them ideal for large-scale applications and advanced AI anomaly detection systems.
Reale Anwendungen der KI-Anomalieerkennung
AI-powered anomaly detection is transforming industries by providing advanced solutions for detecting unusual patterns and potential risks. From cybersecurity to healthcare, AI is being used to identify fraud, monitor system irregularities, and improve decision-making across a variety of sectors.
This section explores the real-world applications of AI anomaly detection and how it is enhancing efficiency and security.
Cybersicherheit: Betrug und Eindringlinge erkennen
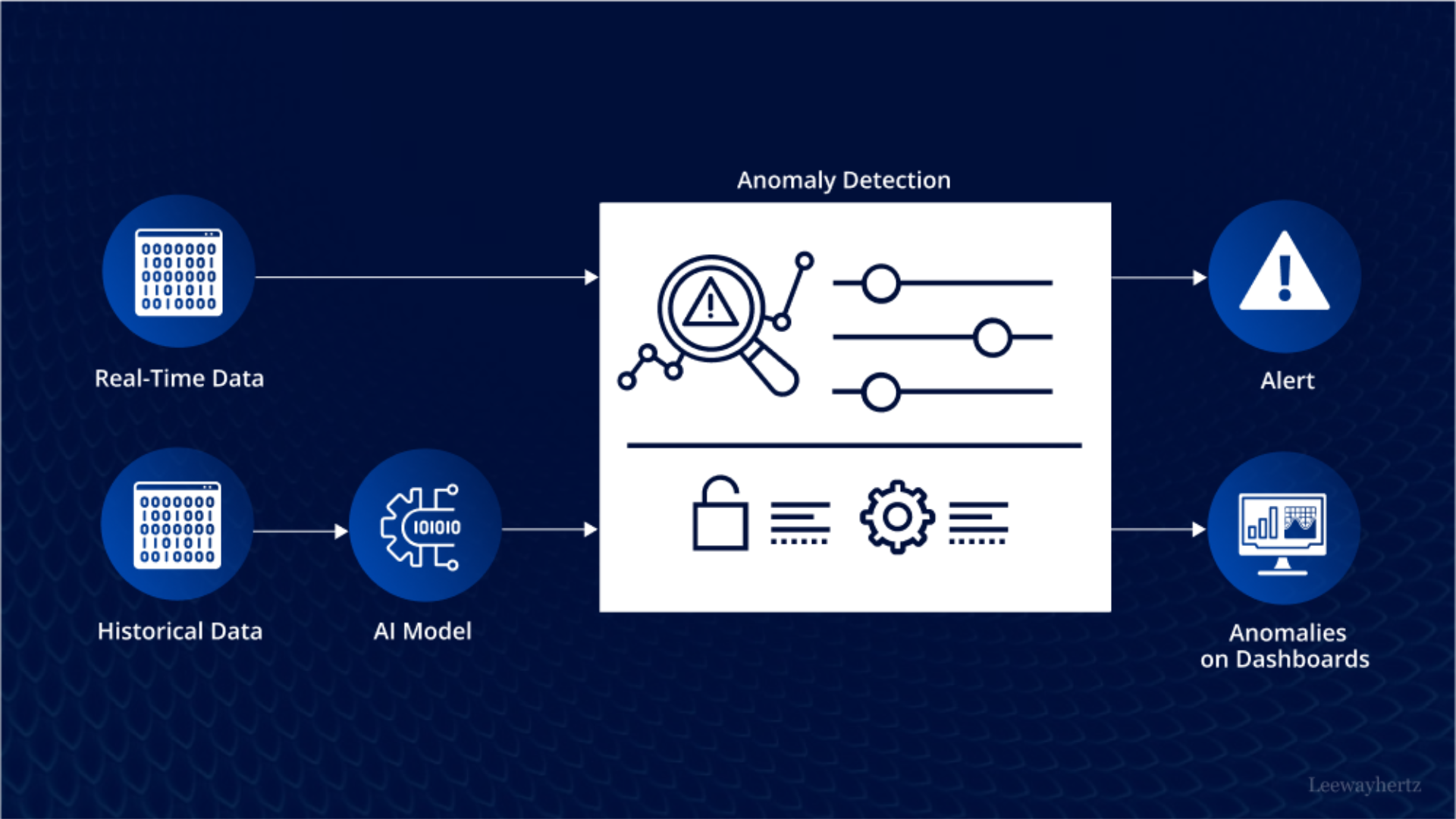
Quelle: KI-Anomalieerkennung: Schutz von Systemen und Daten – Medium
AI-based anomaly detection is critical in cybersecurity for identifying fraud and intrusions by analyzing network traffic, user behaviors, and system logs.
AI anomaly detection models, particularly those based on machine learning, can detect unusual patterns in real-time, which may indicate cyberattacks, such as malware or unauthorized access.
According to arXiv, AI models are increasingly used for detecting sophisticated attacks that traditional methods might miss, improving threat detection and reducing false positives in real-time environments.
Gesundheitswesen: Erkennen medizinischer Anomalien
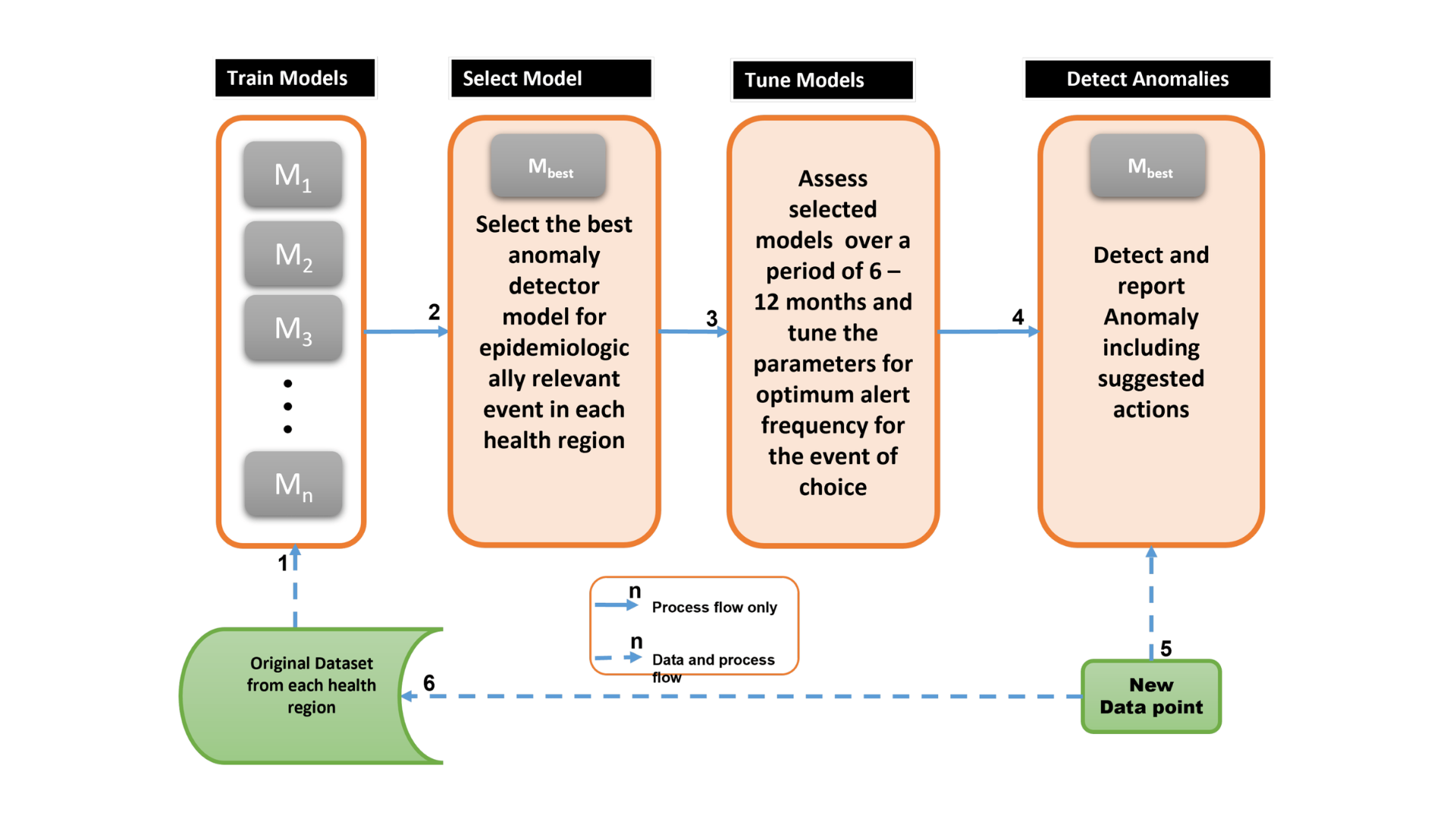
Quelle: Anomalieerkennung in Daten zur Überwachung endemischer Krankheiten mithilfe maschineller Lerntechniken – MDPI
In healthcare, AI-powered anomaly detection can identify unusual medical patterns in patient data, such as abnormal test results or rare diseases. These systems can help medical professionals quickly detect conditions like cancer or cardiac events before they become critical.
A study in PubMed emphasizes the importance of anomaly detection in medical imaging and diagnostics, helping to identify anomalies that might be overlooked by human clinicians, ultimately improving patient outcomes.
Finanzen: Verhinderung betrügerischer Transaktionen
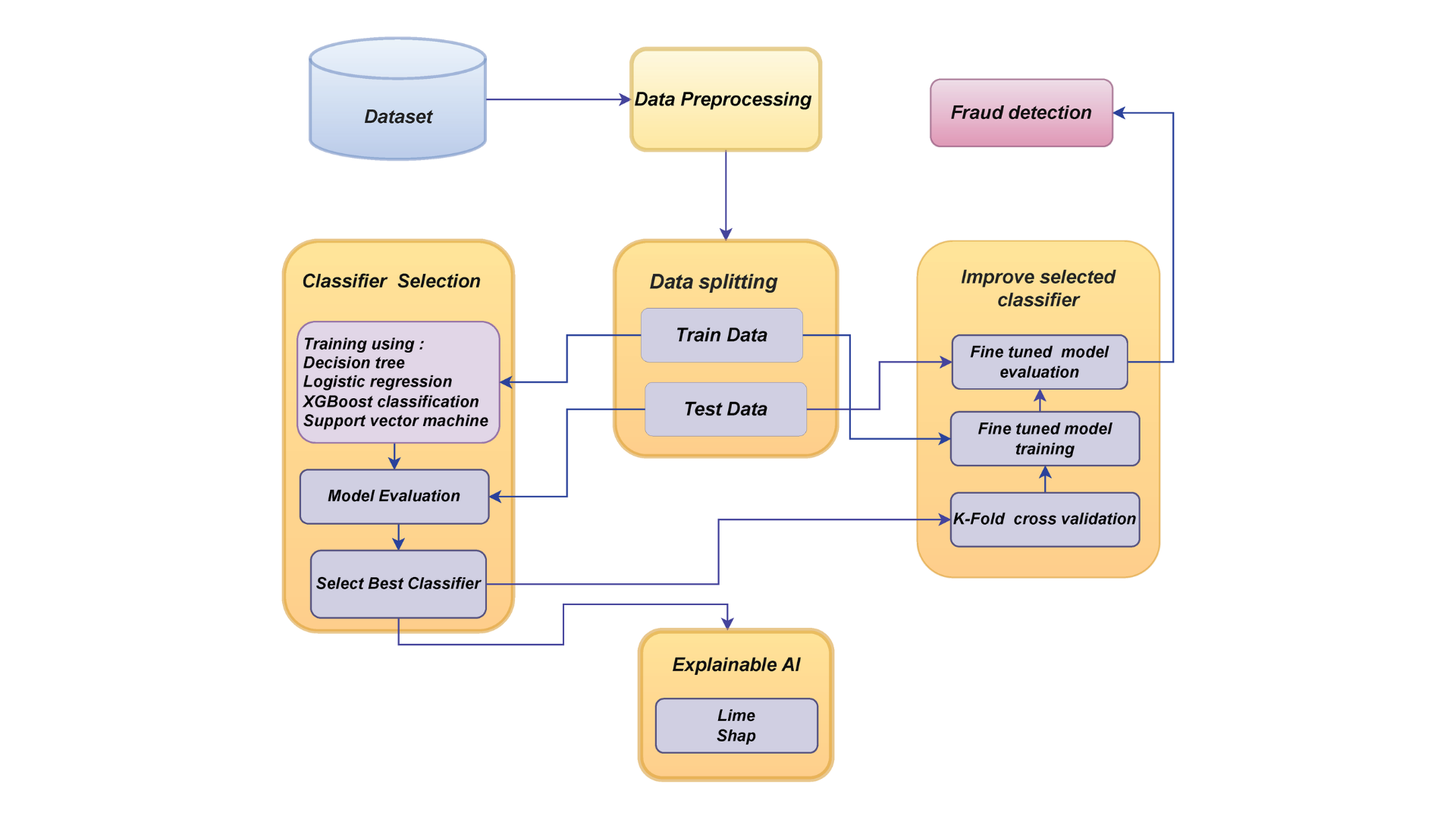
Quelle: Aufdeckung von Bankbetrug: Die Macht des maschinellen Lernens und der erklärbaren KI (XAI) bei unausgewogenen Daten entfesseln – MDPI
AI is increasingly used in finance to detect fraudulent activities, such as unauthorized transactions, money laundering, or credit card fraud. Machine learning models are trained to recognize the typical behaviors of users and flag any outliers as potential fraud.
Research in arXiv demonstrates how AI anomaly detection improves fraud detection by adapting to evolving transaction patterns, reducing the risk of financial losses and increasing security in banking systems.
Fertigung: Vorausschauende Wartung und Fehlererkennung
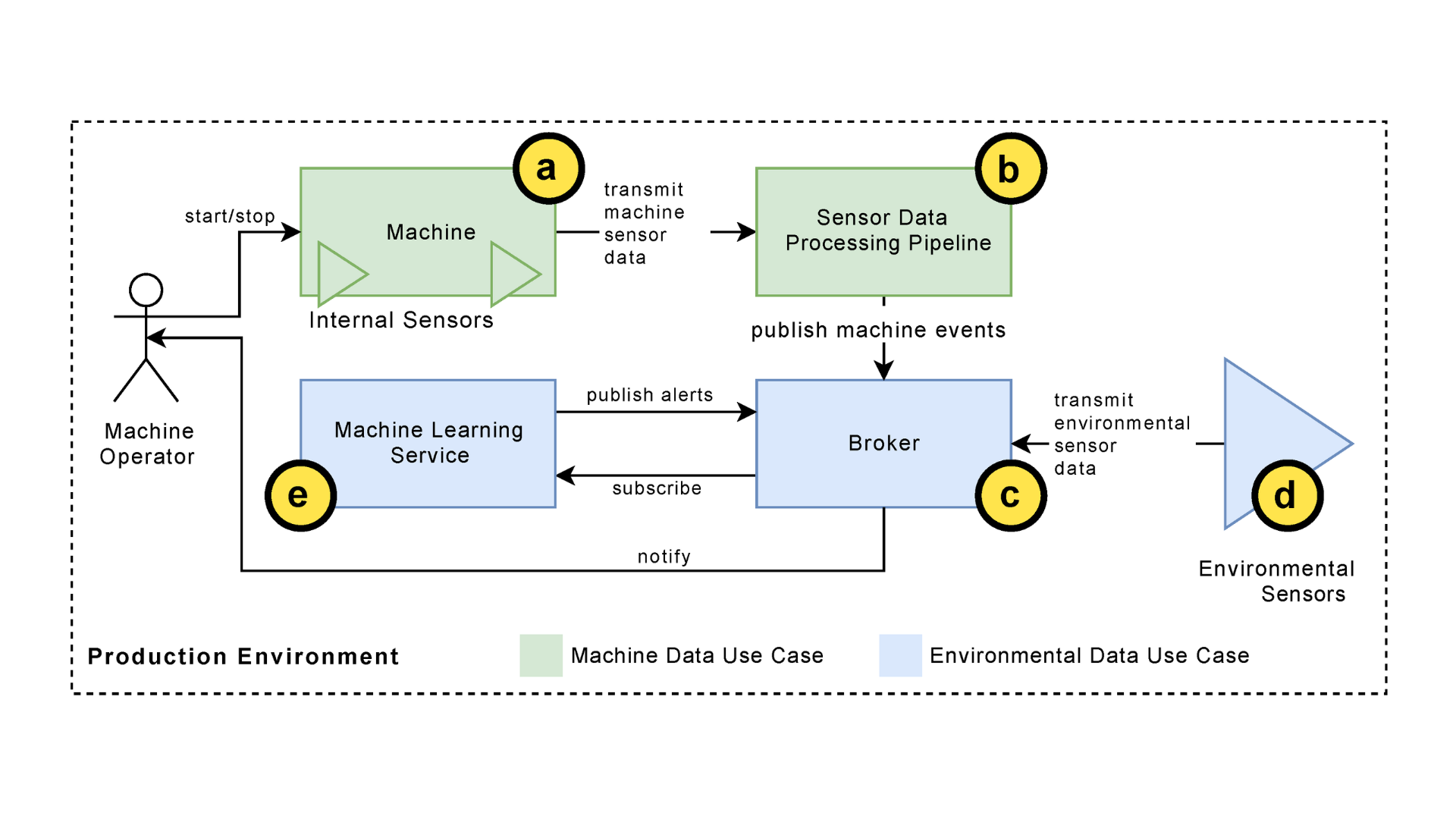
Quelle: Anomalieerkennung für Fertigungssysteme auf Basis von Sensordaten – Einblicke in zwei anspruchsvolle reale Produktionsumgebungen – MDPI
In manufacturing, anomaly detection helps predict equipment failures before they occur by analyzing data from sensors and machinery. AI models can detect patterns that indicate potential failures, allowing for timely maintenance and reducing downtime.
According to research from SpringerLink, predictive maintenance powered by AI anomaly detection improves operational efficiency, extends the lifespan of industrial equipment, and reduces unplanned downtime, enhancing production efficiency.
Netzwerksicherheit: Überwachung von Systemunregelmäßigkeiten
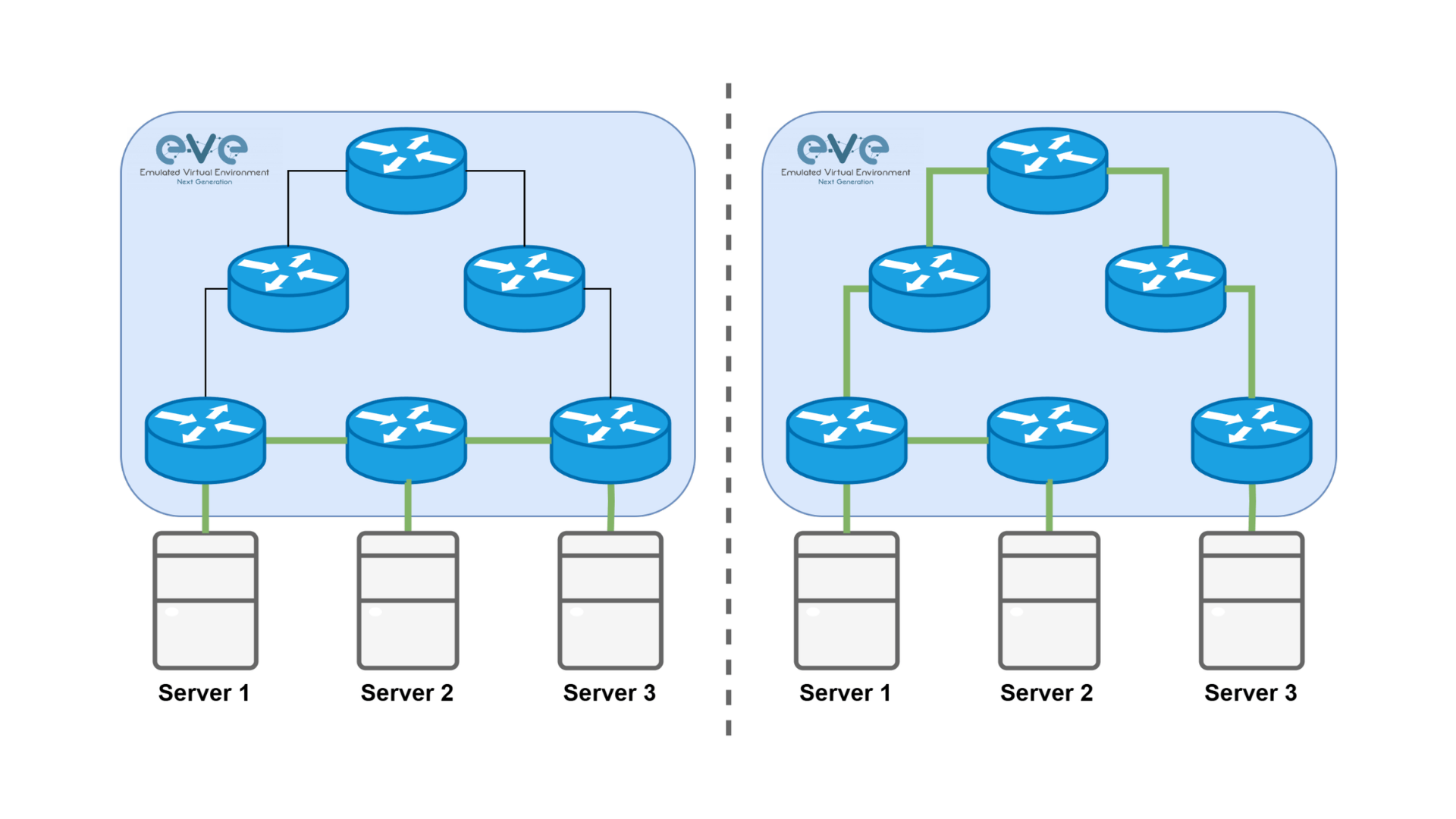
Quelle: Auf maschinellem Lernen basierende Erkennung von Netzwerkanomalien: Design, Implementierung und Evaluierung – MDPI
AI-powered anomaly detection plays a crucial role in network security by monitoring system performance and detecting irregularities in network traffic, server logs, or communication protocols. These systems can quickly identify threats such as DDoS attacks or network breaches.
A study from IEEE Xplore highlights how AI-based models in network security are improving the detection of previously undetected anomalies, enhancing the security of critical infrastructure and providing real-time responses to potential threats.
Aufbau eines KI-Anomalieerkennungssystems
Building an AI anomaly detection system requires careful planning and the right selection of techniques, data sources, and algorithms. By leveraging AI anomaly detection, organizations can create systems that effectively identify outliers and abnormal patterns across different use cases, from fraud detection to predictive maintenance.
This section covers the key steps involved in building a successful AI-powered anomaly detection system.
Ziele und Anwendungsfälle definieren
The first step in building an AI anomaly detection system is defining clear objectives and use cases. Understanding the specific problem, such as fraud detection, predictive maintenance, or network security, is essential for selecting the appropriate techniques and models.
According to arXiv, a well-defined use case helps align the system’s capabilities with business goals and ensures the model can accurately detect relevant anomalies in the given context, improving the overall effectiveness of AI for anomaly detection.
Auswahl der richtigen Datenquellen
Choosing the right data sources is crucial for training a successful anomaly detection model. The data should be relevant to the identified use case and include sufficient examples of both normal and anomalous behavior.
A study from SpringerLink highlights the importance of incorporating diverse data types, such as time-series data, sensor data, and transactional records, to capture the full range of anomalies that may arise in the system. The success of AI anomaly detection depends on the quality and variety of data used in training the model.
Datenvorverarbeitung und Feature Engineering
Data preprocessing and feature engineering are critical steps in building an anomaly detection system. This stage involves cleaning and transforming raw data into a format that can be easily analyzed by the model.
Techniques such as normalization, missing data imputation, and feature selection are essential to improve model performance. As noted by arXiv, effective preprocessing ensures that the model learns the most relevant patterns, reducing noise and improving the accuracy of AI anomaly detection by focusing on critical features.
Auswahl der besten Algorithmen
Selecting the right algorithm depends on the data type, the complexity of the problem, and the available computational resources. Common algorithms include statistical methods, machine learning models (such as Isolation Forest and One-Class SVM), and deep learning approaches like autoencoders.
A study from arXiv suggests that hybrid models combining multiple algorithms often provide better results by leveraging the strengths of different techniques, providing more robust and scalable AI anomaly detection systems.
Modelltraining, Validierung und Bereitstellung
Once the model is selected, the next step is training it on labeled or unlabeled data. It is essential to use proper validation techniques, such as cross-validation, to assess the model’s performance and prevent overfitting. After training, the model can be deployed for real-time anomaly detection.
According to SpringerLink, continuous monitoring and periodic retraining of the model ensure that it adapts to new data and maintains high detection accuracy over time, making it a key component of AI-powered anomaly detection systems in real-world applications.
Tools und Plattformen zur KI-Anomalieerkennung
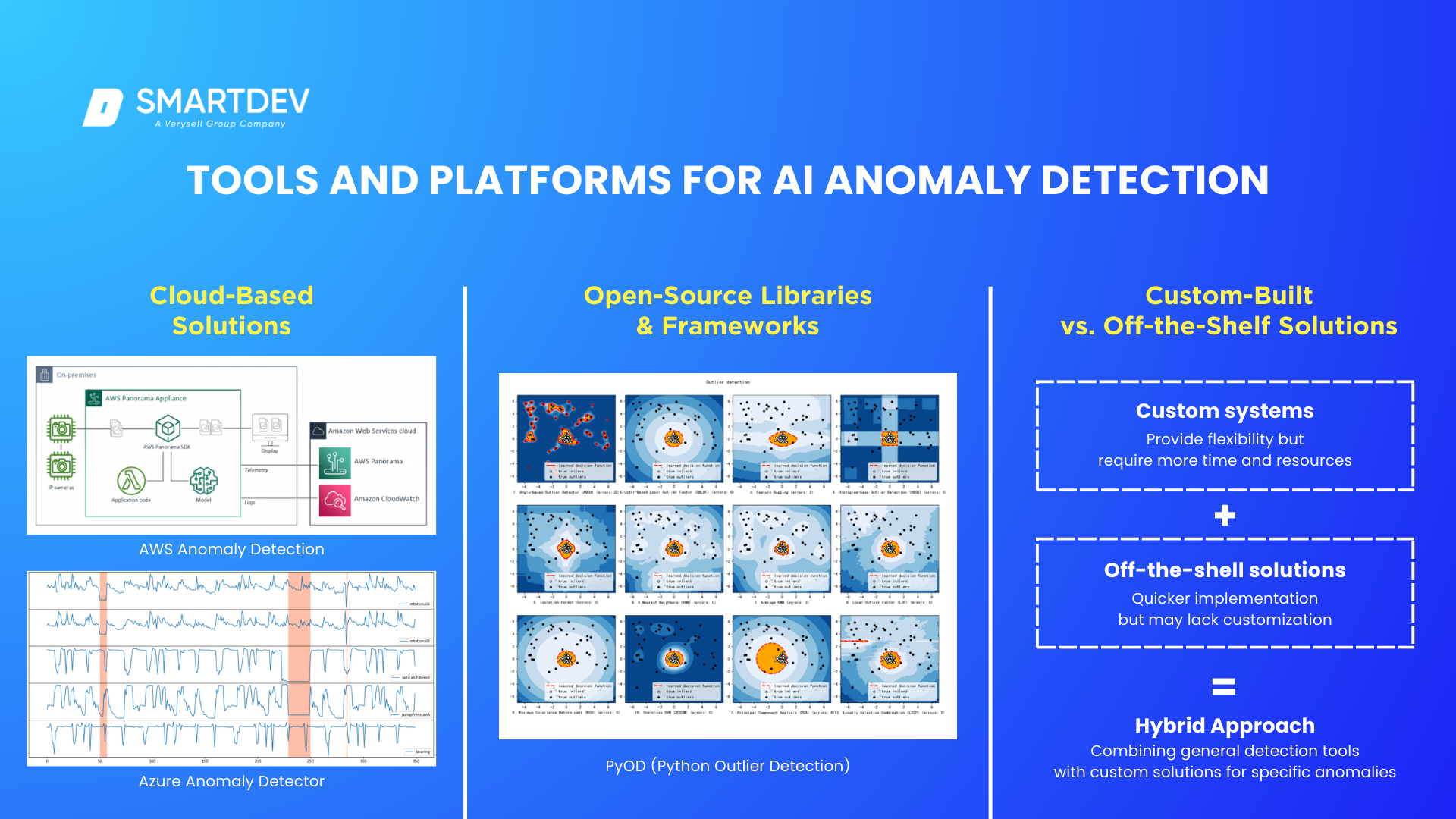
Quelle: Microsoft, AWS, PyOD
AI anomaly detection is made even more powerful with the use of specialized tools and platforms. Whether using cloud-based solutions for scalability, open-source libraries for customization, or opting for custom-built systems, businesses have many options to create tailored anomaly detection solutions.
This section explores the leading tools and platforms for AI-powered anomaly detection.
Cloud-basierte Lösungen
Cloudbasierte Lösungen bieten skalierbare und flexible Funktionen zur Anomalieerkennung bei minimalem Einrichtungs- und Wartungsaufwand. Große Cloud-Anbieter bieten spezialisierte Tools zur Anomalieerkennung für verschiedene Branchen an.
AWS-AnomalieerkennungAWS bietet einen robusten KI-Dienst zur Anomalieerkennung, der sich in andere AWS-Tools integrieren lässt und es Unternehmen ermöglicht, große Datensätze zu analysieren und Anomalien in Echtzeit zu erkennen. Laut AWS-Dokumentation wird dieser Dienst häufig zur Überwachung von Maschinendaten und zur Erkennung von Anomalien im Netzwerkverkehr, bei Transaktionen und IoT-Daten eingesetzt.
Azure-AnomalieerkennungMicrosoft Azure bietet einen KI-basierten Anomalieerkennungsdienst zur Erkennung von Unregelmäßigkeiten in Zeitreihendaten. Der Dienst ist vollständig verwaltet und erfordert nur minimale Einrichtung. Wie in Microsoft Azure beschrieben, wird dieser Dienst häufig für vorausschauende Wartung, IoT-Datenüberwachung und Finanzprognosen verwendet.
Google Cloud AI-Lösungen: Google Cloud bietet KI-Tools wie Cloud AI und AutoML zur Anomalieerkennung in verschiedenen Datentypen, einschließlich Zeitreihen- und Sensordaten. Laut Google CloudDiese Tools werden zur Anomalieerkennung in Bereichen wie Sicherheit, Gesundheitswesen und Einzelhandelsanalyse verwendet.
Open-Source-Bibliotheken und Frameworks
Für diejenigen, die eine stärkere Anpassung bevorzugen oder Anomalieerkennungssysteme von Grund auf neu erstellen möchten, bieten mehrere Open-Source-Bibliotheken leistungsstarke Tools zur Anomalieerkennung.
TensorFlow-AnomalieerkennungTensorFlow ist ein weit verbreitetes Open-Source-Framework für maschinelles Lernen, das fortschrittliche Tools für den Aufbau von KI-Anomalieerkennungssystemen bietet. Die Anomalieerkennungsfunktionen von TensorFlow können große Datensätze und komplexe Modelle wie Autoencoder und tiefe neuronale Netzwerke verarbeiten. Forschungen in arXiv belegen die Effektivität von TensorFlow-basierten Modellen bei der Erkennung komplexer Anomalien in unstrukturierten Daten.
PyOD (Python-Ausreißererkennung): PyOD ist eine Open-Source-Python-Bibliothek, die eine breite Palette von Algorithmen zur Anomalieerkennung bietet, von klassischen statistischen Methoden bis hin zu Techniken des maschinellen Lernens. Wie in der PyOD-Dokumentation hervorgehoben, ist sie eine beliebte Bibliothek zum Aufbau skalierbarer KI-Systeme zur Anomalieerkennung, insbesondere bei der Arbeit mit hochdimensionalen Datensätzen.
Scikit-Learn zur Anomalieerkennung: Scikit-Learn ist eine weitere beliebte Python-Bibliothek, die verschiedene Machine-Learning-Algorithmen zur Anomalieerkennung bereitstellt, darunter One-Class SVM und Isolation Forest. Laut Scikit-Learn-Dokumentation, diese Bibliothek wird häufig für einfachere Aufgaben zur Anomalieerkennung verwendet, bei denen Benutzerfreundlichkeit und Interpretierbarkeit im Vordergrund stehen.
Maßgefertigte Lösungen vs. Standardlösungen
When deciding between custom-built systems and off-the-shelf solutions, organizations must consider the specific needs of their anomaly detection use case.
Custom-built systems offer greater flexibility, allowing for tailored models and integrations, but they require more time, expertise, and resources.
On the other hand, off-the-shelf solutions offer quicker implementation and are generally easier to maintain. However, they may lack the customization needed for more complex or unique use cases.
According to arXiv, many companies opt for hybrid approaches, using off-the-shelf tools for general detection and custom solutions for domain-specific anomalies.
Bewertung der Leistung von KI-Modellen zur Anomalieerkennung

Quelle: Eine Studie zu Leistungsmetriken für die Anomalieerkennung basierend auf Betriebsdaten industrieller Steuerungssysteme – MDPI
Evaluating the performance of AI anomaly detection models is essential to ensure they are effective in real-world applications. By using the right performance metrics, organizations can assess the accuracy and efficiency of their models.
This section explores key metrics, trade-offs, and common pitfalls to consider when evaluating AI anomaly detection systems.
Wichtige Kennzahlen zur Messung der Genauigkeit
Die Bewertung der Leistung von Anomalieerkennungsmodellen ist entscheidend, um ihre Wirksamkeit in realen Anwendungen sicherzustellen. Zur Bewertung von Genauigkeit und Leistung werden häufig verschiedene Metriken verwendet.
Präzision, Recall, F1-Score
Precision, recall, and F1-score are essential metrics for measuring the accuracy of anomaly detection models.
- Precision refers to the proportion of true positive predictions out of all positive predictions made by the model, while recall indicates the proportion of actual anomalies correctly identified.
- F1-score is the harmonic mean of precision and recall, providing a balanced measure of model performance.
According to arXiv, these metrics are especially useful in imbalanced datasets where anomalies are rare, which is common in AI for anomaly detection tasks.
ROC-Kurve und AUC
The Receiver Operating Characteristic (ROC) curve is a graphical representation of a model’s ability to distinguish between classes (normal vs. anomaly). The Area Under the Curve (AUC) is a scalar value that represents the model’s overall ability to discriminate.
A higher AUC indicates better model performance. Research from IEEE Xplore highlights the importance of AUC in comparing different models and choosing the most efficient one for anomaly detection AI tasks, particularly when working with diverse and large-scale datasets.
Kompromisse zwischen falsch positiven und falsch negativen Ergebnissen
In anomaly detection, there is often a trade-off between false positives and false negatives. A false positive occurs when a normal data point is incorrectly classified as an anomaly, while a false negative happens when an anomaly is missed.
The ideal model minimizes both, but depending on the application, one might be more acceptable than the other. As noted in SpringerLink, understanding the specific use case helps in deciding which metric to prioritize, especially in sensitive applications like fraud detection and healthcare, where minimizing false negatives can be critical.
Häufige Fehler und wie man sie vermeidet
Bei der Bewertung von Modellen zur Anomalieerkennung gibt es mehrere häufige Fallstricke, die zu irreführenden Ergebnissen oder einer suboptimalen Leistung führen können.
Überanpassung
Overfitting occurs when a model learns the noise in the training data rather than general patterns, which can lead to poor performance on unseen data.
To avoid overfitting, it is crucial to use techniques like cross-validation and regularization, as suggested by arXiv. Implementing these techniques ensures that AI anomaly detection models generalize well to new, unseen data and do not become too specialized to the training data.
Datenungleichgewicht ignorieren
In anomaly detection, anomalies are often much rarer than normal data, leading to data imbalance. Ignoring this imbalance can result in a model that predicts mostly normal data, leading to low recall.
Techniques such as oversampling, undersampling, or using specialized metrics like balanced accuracy can mitigate this issue, as explained in SpringerLink. Using these techniques ensures that the model remains sensitive to detecting rare anomalies, which is critical for AI anomaly detection in fields like fraud prevention and cybersecurity.
Unzureichende Bewertung
Die Bewertung eines Modells anhand nur einer Metrik kann zu unvollständigen Schlussfolgerungen führen. Um ein umfassendes Verständnis der Modellleistung zu erhalten, ist die Verwendung einer Kombination von Metriken wichtig. Wie in arXiv erwähnt, stellt der Einsatz mehrerer Bewertungsmetriken sicher, dass das Modell in verschiedenen Szenarien effektiv funktioniert und reduziert das Risiko, kritische Anomalien in komplexen Datensätzen zu übersehen.
Zukünftige Trends bei der KI-Anomalieerkennung
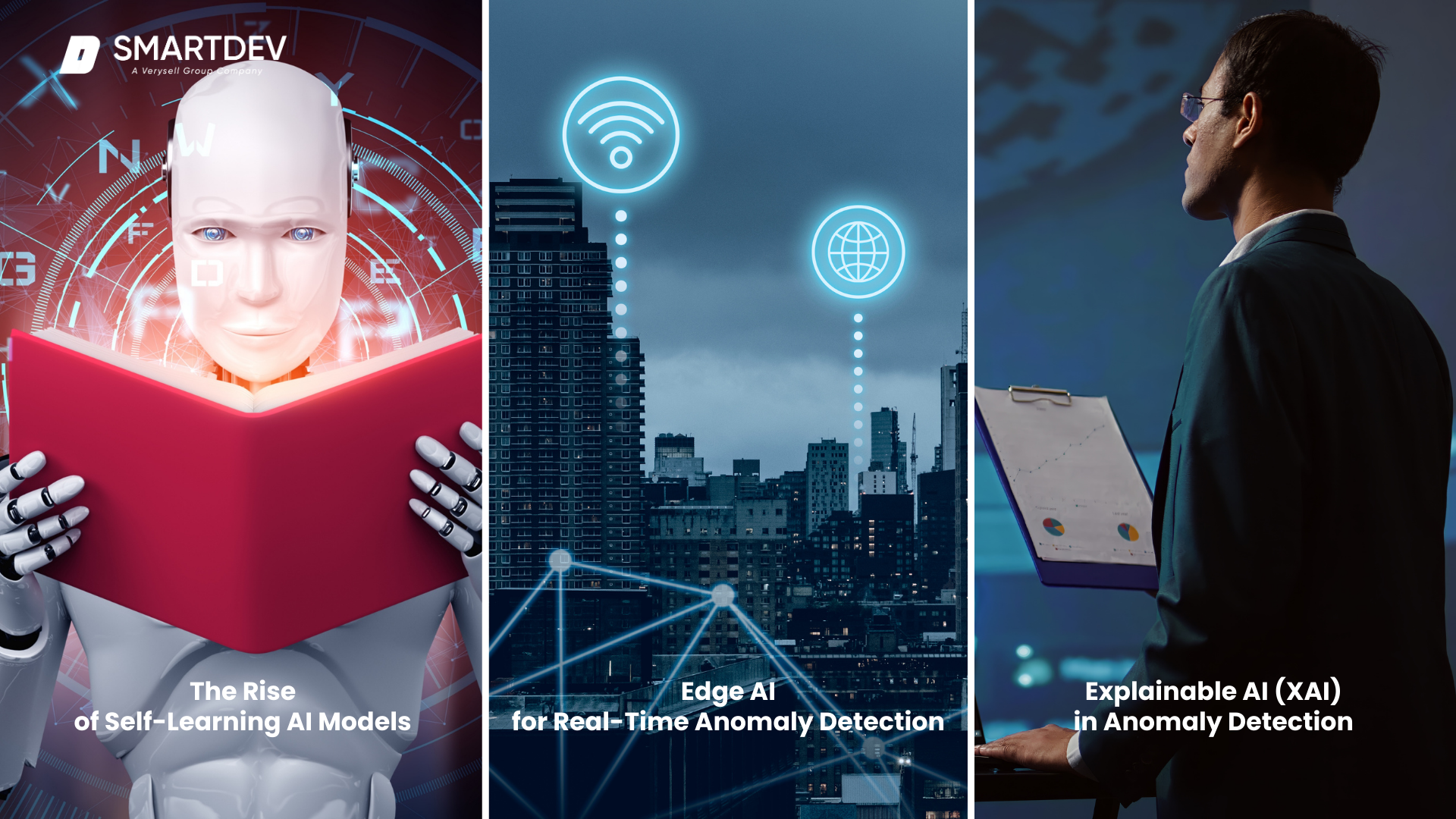
Was hält die Zukunft für KI bei der Anomalieerkennung bereit?
As AI-powered anomaly detection evolves, emerging trends such as self-learning models, edge AI, and explainable AI (XAI) are reshaping how organizations detect anomalies in real-time. The integration of AI-driven automation is also revolutionizing industries, allowing for faster and more accurate anomaly detection with reduced human intervention.
This section highlights the future trends in AI anomaly detection and how they will impact various sectors.
Der Aufstieg selbstlernender KI-Modelle
Self-learning AI models, also known as unsupervised or semi-supervised learning systems, are gaining traction in anomaly detection. These models are capable of learning from unlabelled data, making them adaptable to evolving patterns without requiring constant human intervention.
Research from arXiv indicates that self-learning models are essential for industries where labeling large amounts of data is impractical, such as in fraud detection or cybersecurity. The rise of self-learning AI anomaly detection marks a shift towards more autonomous and flexible systems that can adapt in real-time to new data.
Edge-KI zur Echtzeit-Anomalieerkennung
Edge AI refers to the deployment of AI anomaly detection models directly on devices or sensors, rather than relying on cloud-based solutions. This allows for faster, real-time anomaly detection without the latency associated with sending data to centralized servers. In sectors like manufacturing, healthcare, and autonomous vehicles, edge AI is becoming increasingly important for detecting anomalies as soon as they occur.
According to IEEE Xplore, edge AI improves efficiency and reduces the risk of security breaches by analyzing data locally and minimizing data transmission.
Erklärbare KI (XAI) in der Anomalieerkennung
Explainable AI (XAI) is a growing trend in anomaly detection, focusing on making AI models transparent and interpretable to users. As AI models become more complex, the need for understanding their decision-making process becomes critical, especially in high-stakes fields like healthcare and finance.
XAI techniques help users understand why a particular anomaly was detected, increasing trust and facilitating better decision-making. A study from arXiv discusses how XAI is improving anomaly detection by providing explanations for model outputs, which is especially useful when models are used to make critical decisions in applications like fraud detection and patient monitoring.
KI-gesteuerte Automatisierung und ihre geschäftlichen Auswirkungen
AI-driven automation in anomaly detection is expected to transform business operations by reducing human intervention and allowing for faster, more accurate detection of issues. Automating anomaly detection processes can lead to cost savings, enhanced operational efficiency, and faster response times.
For instance, in cybersecurity, AI anomaly detection can autonomously detect and mitigate threats in real-time, reducing the reliance on security personnel.
According to McKinsey & Company, AI-driven automation has the potential to revolutionize industries by enhancing predictive capabilities and enabling proactive management of anomalies, rather than just reactive measures.
Fazit und nächste Schritte
AI-driven anomaly detection is rapidly transforming how organizations identify and manage irregularities across various industries. By leveraging AI anomaly detection and advanced machine learning techniques, businesses can enhance decision-making and improve operational efficiency.
As AI continues to evolve, the integration of self-learning models, edge computing, and explainable AI will further enhance anomaly detection capabilities.
This section outlines the key takeaways, steps for implementing AI anomaly detection, and additional resources for further learning.
Die wichtigsten Erkenntnisse
AI-driven anomaly detection is a powerful tool for identifying irregularities and outliers across various industries, from cybersecurity to healthcare and finance.
By leveraging machine learning, deep learning, and hybrid models, organizations can detect complex anomalies in real-time and improve decision-making. As AI continues to evolve, self-learning models, edge computing, and explainable AI are expected to play an even more significant role in enhancing the accuracy and transparency of anomaly detection systems.
Implementierung der KI-Anomalieerkennung in Ihrem Unternehmen
Implementing AI anomaly detection can significantly enhance your business operations by identifying potential issues early, reducing risks, and improving efficiency. SmartDev specializes in providing AI solutions tailored to your specific needs, from custom-built anomaly detection systems to leveraging cloud-based platforms for scalability.
If you’re ready to adopt AI-driven anomaly detection to gain actionable insights and improve operational resilience, contact SmartDev today for a consultation.
Zusätzliche Ressourcen für weiteres Lernen
Für diejenigen, die sich eingehender mit der Erkennung von KI-Anomalien befassen möchten, stehen verschiedene Ressourcen zur Verfügung, um Ihr Verständnis zu vertiefen:
- Bücher: „Hands-On Anomaly Detection with Python“ von MAA Awan ist eine großartige Ressource für praktische Anleitungen zum Erstellen von Anomalieerkennungsmodellen mit Python.
- Forschungsarbeiten: Erkunden Sie die neuesten Fortschritte bei der Anomalieerkennung anhand wissenschaftlicher Artikel auf arXiv.
- Online-Kurse: Plattformen wie Coursera und edX bieten Kurse zu maschinellem Lernen und Anomalieerkennung an, um Ihnen den Einstieg in die KI zu erleichtern.
Indem Sie tiefer in diese Ressourcen eintauchen, können Sie Ihr Wissen erweitern und über die neuesten Trends und Techniken zur KI-Anomalienerkennung auf dem Laufenden bleiben.
—
Verweise
- Zhao, Z. (2017). Ein Beispiel für eine Punktanomalie. ResearchGate. https://www.researchgate.net/figure/An-example-of-a-point-anomaly_fig1_270274504
- Zhao, Z. (2017). Ein Beispiel für eine kontextuelle Anomalie. ResearchGate. https://www.researchgate.net/figure/An-example-of-a-contextual-anomaly_fig2_270274504
- Chakraborty, S. (26. Mai 2020). Anomalieerkennung mit Z-Score: Pflücken Sie die niedrig hängenden Früchte. Medium. https://medium.com/swlh/anomaly-detection-with-z-score-pick-the-low-hanging-fruits-ccd5ccccaee9
- GeeksforGeeks. (nd). Was ist Isolation Forest? GeeksforGeeks. https://www.geeksforgeeks.org/what-is-isolation-forest/
- Garima, M. (7. Juni 2021). Ein-Klassen-SVM (OC-SVM). Medium. https://medium.com/@mail.garima7/one-class-svm-oc-svm-9ade87da6b10
- NVIDIA. (nd). K-Mittel. NVIDIA. https://www.nvidia.com/en-us/glossary/k-means/