In a sector traditionally plagued by high costs, lengthy timelines, and limited success rates, drug discovery has been taking both immense and baby steps in securing the human future with a new cure for the new age.
24 years into the new millennium, pharmaceutical companies are reaching the breakthrough we all long for, with the biggest help from Artificial Intelligence (AI). AI is tearing down old barriers, accelerating breakthroughs, and redefining what’s possible in medicine.
Take the case of DeepMind’s AlphaFold, the system accurately predicted the 3D structures of over 200 million proteins, covering nearly every known protein in science. This achievement enables researchers to understand complex biological processes at an unprecedented level, shaving years off drug discovery timelines and opening doors to targeted treatments for diseases previously deemed untreatable.
1. The history of the last decade
Over the past decade, artificial intelligence (AI) has significantly transformed drug discovery, leading to the emergence of numerous AI-driven companies and innovative methodologies.
2012–2014: Early adoption and technological advancements
The deep learning revolution began around 2012, marked by the development of AlexNet, which excelled in image recognition tasks. This period also saw the introduction of Generative Adversarial Networks (GANs) in 2014, enhancing AI’s generative capabilities. These advancements spurred the foundation of AI-focused drug discovery companies such as Atomwise, Exscientia, and Insilico Medicine, which applied deep learning and GANs to molecular design and target identification.
2015–2019: Expansion and diversification
During this time, the AI-driven drug discovery landscape expanded with the establishment of companies like Insitro, Relay Therapeutics, and Valo Health. These firms utilized AI to address various aspects of drug development, including disease modeling and small molecule design. Notably, Schrödinger, founded in 1990, integrated AI into its existing computational platforms, enhancing its drug discovery capabilities.
2020–2024: Maturation and clinical integration
The recent years have witnessed AI’s maturation in drug discovery, with several companies advancing their AI-designed drug candidates into clinical trials. For instance, Insilico Medicine’s AI-discovered drug for idiopathic pulmonary fibrosis (scarred lungs disease) entered Phase I clinical trials, demonstrating AI’s potential to expedite the drug development process. Additionally, collaborations between pharmaceutical companies and AI firms have increased, aiming to leverage AI for more efficient and cost-effective drug discovery. Overall, the past decade has seen AI evolve from a novel concept to a pivotal component in drug discovery, enhancing efficiency, reducing costs, and accelerating the development of new therapeutics.
2. Current market landscape and investment trends in AI drug discovery
2.1. Current industry growth
The adoption of AI in drug discovery is accelerating, as the market is currently 1.86 billion USD and is expected to reach 6.89 billion USD by 2029, at a CAGR of 29.9%. This is fueled by pharmaceutical demand for efficient, data-driven approaches. As AI adoption scales, companies are gaining a competitive edge with faster, more cost-effective drug development.
2.2. Investment in AI pharma startups
Investor interest in AI-driven drug discovery is surging immensely. Venture capital and private equity funding for AI pharma startups have grown exponentially, supporting innovations that accelerate drug development and improve outcomes. This influx of capital is driving rapid advancements and encouraging more companies to integrate AI solutions into their R&D pipelines.
The most notable case is that a startup developing robots foundational software, Physical Intelligence, has raised $400 million in early-stage funding from Amazon’s Jeff Bezos, OpenAI, venture capital firms Thrive Capital and Lux Capital.
2.3. The players leading the big game
Key players in the AI-driven drug discovery market include tech giants like Google and Microsoft, alongside specialized biotech firms such as BenevolentAI and Exscientia. These companies are at the forefront, leveraging AI to optimize molecular modeling, target identification, and clinical trial efficiency, setting new industry standards in precision and speed. The AI adoption ecosystem can be categorized into 3 groups: the direct AI vendors, the enablers and the end users as shown in the picture below. This growing market landscape underscores AI’s transformative impact on pharmaceuticals, with strong investor backing and increasing adoption propelling the industry forward.
3. The process of designing drugs with AI
AI is revolutionizing drug discovery by enhancing efficiency and precision across various stages of the development process. The integration of AI into drug design involves several key steps:
Candidate identification and validation: AI algorithms analyze extensive biological data to identify and validate potential drug targets, such as proteins or genes associated with specific diseases. This data-driven approach enables researchers to pinpoint novel targets that may not be evident through traditional methods.
Testing optimization: Once targets are identified, AI models assist in generating and optimizing chemical compounds that can interact effectively with these targets. Machine learning techniques predict the binding affinity and activity of these compounds, facilitating the selection of promising candidates for further development.
Predictive modeling for ADMET properties: AI tools predict the Absorption, Distribution, Metabolism, Excretion, and Toxicity (ADMET) profiles of potential drug candidates. By forecasting these pharmacokinetic and safety parameters, AI helps in identifying compounds with favorable profiles, reducing the likelihood of late-stage failures.
Clinical trial design and patient stratification: AI aids in designing efficient clinical trials by analyzing patient data to identify suitable participants and predict their responses to treatments. This stratification enhances trial outcomes and accelerates the approval process by ensuring that therapies are tested on the most appropriate patient groups. By integrating AI into these stages, the drug discovery process becomes more streamlined, cost-effective, and capable of delivering innovative therapies to patients more rapidly.
4. Case studies: AI most successful applications in drug discovery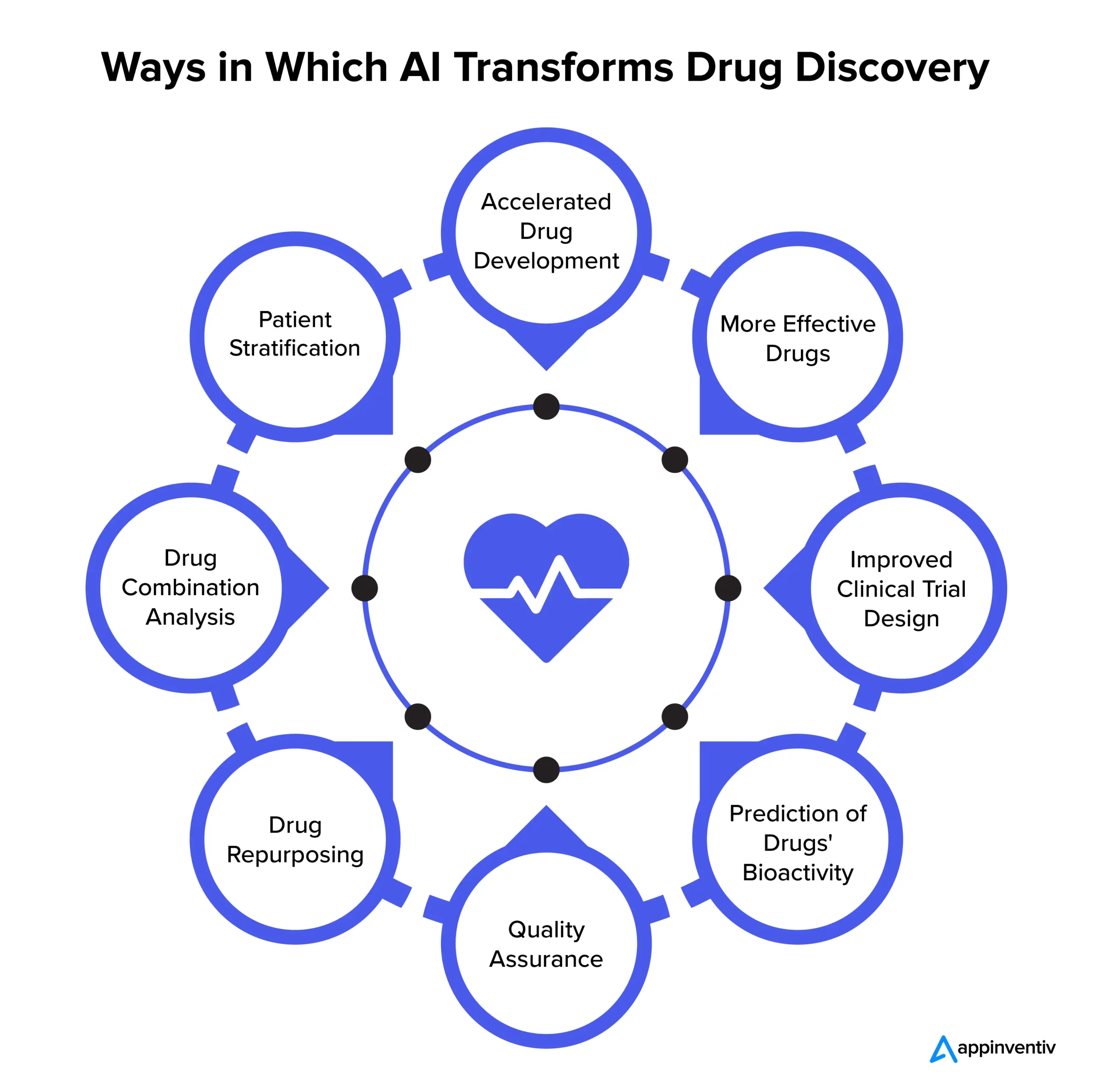
4.1. AlphaFold by DeepMind – 3D structure
AlphaFold has transformed protein structure prediction, enabling researchers to determine 3D protein structures from amino acid sequences with remarkable accuracy. This advancement accelerates drug design by providing insights into protein folding, crucial for understanding disease mechanisms and developing targeted therapies.Image source
4.2. IBM Watson – Small molecule detection
IBM Watson leverages AI to analyze vast datasets, assisting in identifying potential drug targets and discovering novel molecules. Its natural language processing capabilities enable researchers to extract insights from scientific literature, patents, and clinical trial data, streamlining the drug development process.Image source
4.3. BenevolentAI – Disease analysis using ML
BenevolentAI utilizes machine learning to predict disease mechanisms and identify potential drug candidates. By integrating biomedical data, it uncovers hidden relationships between genes, diseases, and drugs, facilitating the discovery of new therapeutic targets and accelerating the development of effective treatments.
4.4. Insilico Medicine – Medical simulations
Insilico Medicine offers end-to-end AI-powered platforms for drug discovery, encompassing molecular design and clinical trial simulations. Its AI algorithms generate novel molecular structures and predict their pharmacological properties, optimizing the drug development pipeline and reducing time to market.
4.5. Exscientia – drug & candidates compatibility identification
Exscientia is an AI-first drug design company that combines automation with human expertise to optimize compound selection. Its AI-driven platforms enable rapid identification of high-quality drug candidates, enhancing efficiency and success rates in drug discovery projects.
4.6. Atomwise – Disease monitor database
Atomwise pioneers virtual screening using AI to analyze extensive libraries of compounds against specific disease targets. Its deep learning models predict the binding affinity of small molecules to protein targets, facilitating the identification of promising drug candidates and expediting the lead optimization process.
4.7. Schrödinger’s Platform – Molecule modeling
Schrödinger integrates physics-based simulations with AI to support molecular modeling and drug candidate evaluation. Its computational platform enables accurate prediction of molecular properties and behaviors, aiding in the design of potent and selective drugs with improved safety profiles.
Explore more case studies & deep thinking about healthcare
5. The main setback: AI risks and limitations
5.1. Affected data quality and quantity
AI models are only as good as the data they’re trained on. In drug discovery, if data is incomplete or unrepresentative, AI algorithms can produce biased or inaccurate results. For instance, a dataset lacking diversity may lead to drugs that are less effective or unsafe for certain populations. This makes high-quality, comprehensive data essential to avoid errors and ensure fairness in AI-driven drug development.
5.2. Model interpretability and transparency
AI models, particularly deep learning algorithms, often function as “black boxes,” meaning their decision-making processes can be difficult for researchers to interpret. In drug discovery, this lack of transparency is a concern since it’s crucial to understand why an AI model recommends a certain molecule or therapeutic approach. Without interpretability, it can be challenging to verify and trust AI-generated results, which limits AI’s full potential in scientific and regulatory settings.
5.3. High initial costs and technical expertise
Implementing AI in drug discovery requires significant upfront investments in technology, software, and specialized talent. Additionally, AI systems require high computational power and infrastructure, which can be costly and may hinder adoption, particularly among smaller biotech firms. These expenses, while often offset by longer-term gains, can be a major barrier to entry.
5.4. Data privacy & Regulatory compliance
The regulatory landscape for AI in drug discovery is still evolving, and many regulatory bodies are cautious about approving AI-generated results without rigorous evidence. The need for AI-specific standards and validation processes can slow the deployment of AI-developed drugs, creating a gap between technological advancements and real-world applications. Regulatory uncertainty remains a challenge as policymakers work to keep pace with rapid AI innovations in the pharmaceutical industry.
5.5. Serious ethical considerations
The patient’s privacy and data security should be the top priority in applying AI in the drug discovery and testing phase. The researchers, pharmaceutical and technology companies need to collaborate and ensure that AI systems protect sensitive patient data and comply with regulations like GDPR and HIPAA. In addition, it is a must for transparent AI models to foster trust among healthcare professionals and patients. In some cases, AI may have biases that need to be addressed to avoid disparities in drug effectiveness across different demographic groups.
5.6. Lack of standardization
One notable challenge is the increasing need for standardized data formats, collection methodologies, and analysis techniques. This interrupts and makes streamlining the process of data and studies comparison more difficult and time-consuming. Consequently, there is visible hindrance in ggenerating consistent and reliable AI predictions and models.
6. Emerging trends in seeking AI power in drug discovery
6.1. Personalized Medicine and Genomics
AI is paving the way for personalized medicine by enabling drugs to be tailored to an individual’s genetic profile. Through analysis of vast genomic datasets, AI can predict how patients with specific genetic markers might respond to a drug, improving efficacy and reducing side effects. This approach holds promise for conditions with high variability in patient response, such as cancer and autoimmune diseases.
6.2. AI-Enhanced CRISPR and Gene Editing
AI is expected to revolutionize gene editing by enhancing the precision and speed of CRISPR technology. AI algorithms can analyze vast genetic information to guide CRISPR’s targeting, making gene therapies more accurate and effective. This combination opens up new avenues for personalized treatments, especially for rare genetic diseases and conditions where conventional treatments fall short.
6.3. Biomarker Discovery
AI’s analytical power is transforming biomarker discovery, allowing researchers to identify molecular markers that predict how a patient will respond to a treatment. By sifting through clinical and biological data, AI can reveal correlations that might otherwise be missed, helping to predict drug efficacy and tailor treatments to individuals. This is particularly valuable in oncology, where biomarkers play a crucial role in therapy selection.
6.4. AI in Real-World Evidence and Post-Market Surveillance
After a drug reaches the market, AI can track its performance in real-world settings by analyzing patient data, adverse events, and treatment outcomes. This post-market surveillance enables pharmaceutical companies to refine treatments based on real-world evidence, ensuring continued efficacy and safety. AI’s ability to aggregate and analyze this data can also help identify unexpected side effects more rapidly, supporting proactive patient care.
6.5. Natural Language Processing (NLP) in Research
NLP is becoming an essential tool for drug discovery as it enables researchers to analyze large volumes of scientific literature, patents, and clinical trial data for insights. By processing text-based data, NLP can identify trends, uncover new potential drug targets, and track developments in specific therapeutic areas. This helps researchers stay on top of the latest discoveries and identify areas for further investigation, fueling continuous innovation in drug development.
7. Measuring and achieve success with SmartDev
Understanding the immense potential of AI in developing new drugs and securing the future of advanced healthcare, pharmaceutical companies should collaborate with big tech companies to achieve this future together.
SmartDev is a leading IT outsourcing company that provides tailored AI and Machine Learning solutions, providing a powerful lending hand to transform your vision into reality. With our expertise in AI-powered solutions and healthcare applications, we can help you streamline drug discovery, enhance patient care, and drive innovation in personalized medicine. Together, let’s build scalable, secure, and effective AI tools that make a tangible impact on health outcomes.
Contact SmartDev today to start leveraging the power of AI in healthcare—because advancing health technology requires the right team behind you. Let’s innovate together for a healthier, data-driven future.