1. Introduction to AI in Operational Efficiency
a. What is Operational Efficiency?
In today’s hyper-competitive business landscape, operational efficiency has evolved from being merely desirable to becoming an existential imperative. Organizations across all sectors face unprecedented pressure to do more with less, deliver faster, and maintain quality standards while navigating economic uncertainties. Artificial Intelligence (AI) has emerged as a transformative force in this quest, redefining what’s possible in business operations.
b. How AI Transforms Operational Processes
Operational efficiency represents the delicate balance between resource consumption and value creation. It encompasses not just cost reduction, but the optimization of time, energy, capital, and human potential. Modern definitions extend beyond traditional metrics to include sustainability, scalability, and adaptability. Organizations with superior operational efficiency can respond more agilely to market shifts, innovate continuously, and maintain resilience during disruptions.
c. The Evolution of AI in Business Operations
The journey of AI in business operations can be traced through several distinct phases:
- Rule-Based Automation (2000s-2010s): Early AI applications focused on automating structured, repetitive tasks using explicit programming rules.
- Machine Learning Era (2010s-present): The explosion of data and computational power enabled algorithms to learn from examples rather than being explicitly programmed.
- Cognitive AI (2020s): Advanced systems now understand natural language, recognize images, and make context-aware decisions.
- Autonomous Operations (Emerging): The next frontier involves AI systems that can operate with minimal human intervention across entire business functions.
2. The Core Benefits of AI for Operational Efficiency
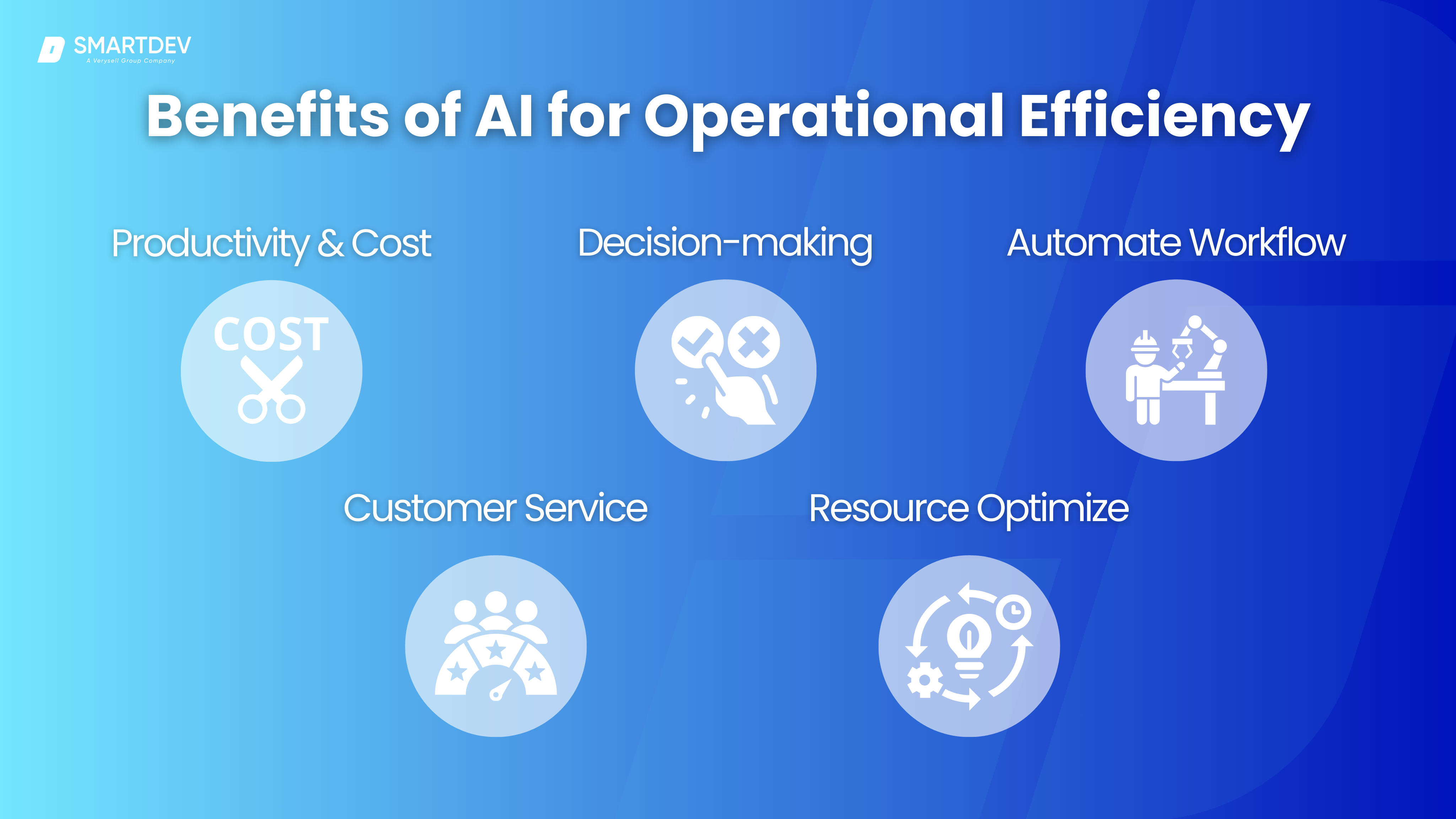
a. Increased Productivity and Cost Reduction
AI-driven automation doesn’t just replace manual labor—it elevates human potential. By handling routine tasks with machine precision, AI frees employees to focus on creative problem-solving and strategic initiatives. The productivity gains extend beyond direct labor savings; they create compounding effects through faster decision cycles and reduced operational friction.
Example: In manufacturing, AI-powered quality control systems can inspect products at production-line speeds, identifying defects with 99.9% accuracy compared to human inspectors’ typical 80-90% accuracy. This not only reduces waste but also prevents costly recalls and protects brand reputation.
b. Enhanced Decision-Making with AI-driven Insights
The true power of AI emerges when it transforms raw data into actionable intelligence. Modern organizations generate vast amounts of operational data that remain untapped—AI serves as the key to unlock this potential.
Example: A leading retail chain implemented AI-powered demand forecasting during the pandemic. While traditional forecasting methods failed due to unprecedented market shifts, the AI system adapted in real-time, analyzing 150+ variables including weather patterns, social media trends, and regional infection rates. This enabled the retailer to maintain 95% service levels despite supply chain disruptions, outperforming competitors by 23% in customer satisfaction metrics.
c. Automation of Repetitive Tasks & Workflow Optimization
Beyond simple task automation, AI excels at workflow orchestration—analyzing entire processes to identify bottlenecks, suggest improvements, and dynamically reroute work based on current conditions.
Implementation Tip: Successful workflow optimization requires mapping not just the official processes but the “shadow workflows” that employees naturally develop to compensate for system limitations. AI can discover these hidden patterns and incorporate them into optimized designs.
d. Improved Customer Service and Experience
AI enables organizations to deliver personalized, 24/7 customer experiences at scale. Modern consumers expect immediate responses, customized solutions, and seamless omnichannel interactions—all capabilities that AI enables.
Organizations implementing AI in customer service report 35-55% reduction in average handle time, 25-40% decrease in operational costs, and 20-35% improvement in customer satisfaction scores (Gartner, 2023).
e. Resource Management and Waste Reduction
AI brings unprecedented precision to resource allocation, whether managing energy consumption, raw materials, or human capital. Predictive analytics enables organizations to anticipate needs before they become critical, avoiding both shortages and over-provisioning.
3. Key AI Technologies Driving Operational Efficiency
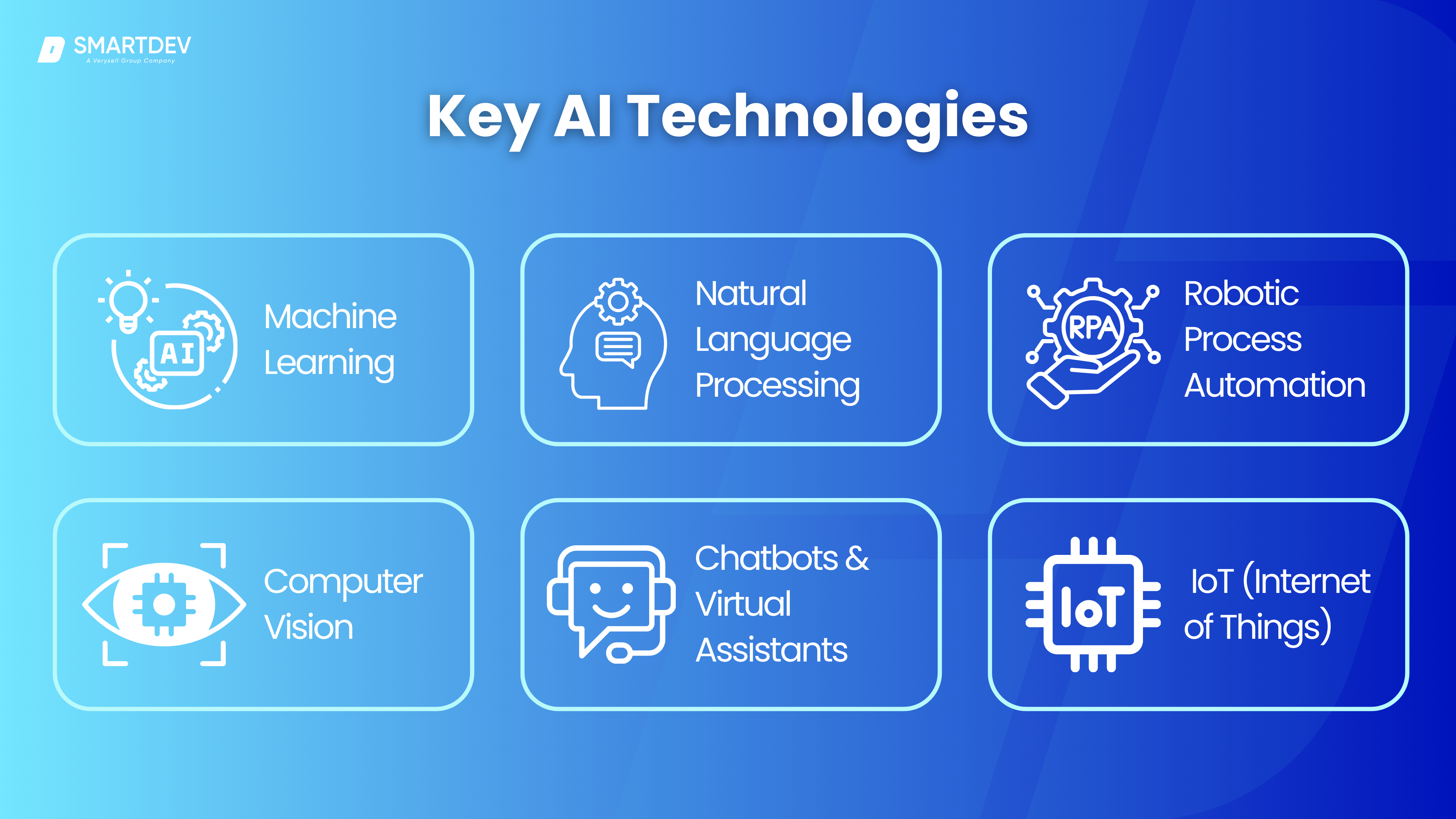
a. Machine Learning (ML) for Predictive Analytics
Machine learning forms the foundation of most AI applications in operations. Supervised learning models predict future outcomes based on historical data, while unsupervised learning discovers hidden patterns without explicit guidance.
Deep learning, a subset of ML using neural networks with multiple layers, has revolutionized image and speech recognition. In manufacturing quality control, deep learning systems can identify microscopic defects invisible to the human eye, improving yield rates by 5-15% (MIT Sloan Management Review, 2023).
b. Natural Language Processing (NLP) for Process Automation
NLP enables machines to understand, interpret, and generate human language, creating opportunities for automation in customer service, document processing, and knowledge management.
While NLP systems have made remarkable progress, they still struggle with context, sarcasm, and cultural nuances. Successful implementations often combine NLP with human-in-the-loop validation systems during the initial deployment phase.
c. Robotic Process Automation (RPA) for Repetitive Tasks
RPA software “bots” automate rule-based digital tasks across applications, from data entry to report generation. When combined with AI capabilities, RPA becomes Intelligent Process Automation (IPA), capable of handling unstructured data and making context-aware decisions.
Organizations typically achieve 200-500% ROI within the first year of RPA implementation, with payback periods averaging 6-12 months (Forrester, 2022).
d. Computer Vision for Quality Control and Monitoring
Computer vision systems analyze visual data from cameras, drones, and sensors to monitor processes, inspect products, and enhance security. In agriculture, computer vision enables precision farming techniques that increase crop yields by 10-20% while reducing water usage by 25-40% (John Deere, 2023).
e. AI-Powered Chatbots and Virtual Assistants
Modern chatbots go beyond simple question-answering to guide users through complex processes, resolve disputes, and even negotiate contracts. Some healthcare providers now use AI chatbots that can conduct preliminary medical diagnoses, triage patients, and schedule appropriate care, reducing emergency department visits by 15-25% for non-urgent cases.
f. AI in IoT (Internet of Things) for Smart Operations
The combination of AI with IoT creates intelligent systems that continuously monitor, analyze, and optimize physical operations. Successful AIoT implementations require robust edge computing capabilities, secure data transmission protocols, and real-time analytics platforms. Organizations should prioritize use cases with clear data flows and measurable outcomes when beginning their AIoT journey.
4. AI Use Cases Across Industries for Operational Efficiency
a. Manufacturing
The manufacturing sector has embraced AI to transform traditional production processes into intelligent operations. Predictive maintenance systems analyze sensor data from machinery to anticipate equipment failures before they cause downtime, reducing unplanned stoppages by 30-50%. AI-driven supply chain optimization allows manufacturers to respond dynamically to market fluctuations, supplier disruptions, and logistical challenges by analyzing multiple variables simultaneously.
Smart robotics and automation have evolved beyond simple repetition, with collaborative robots (cobots) working alongside human operators, learning from their actions, and adapting to optimize production workflows, increasing output while maintaining quality standards.
b. Retail & E-commerce
AI has revolutionized retail operations by enabling data-driven decision making. AI-driven demand forecasting analyzes historical sales data, market trends, and even social media sentiment to predict customer with remarkable accuracy, allowing retailers to maintain optimal inventory levels.
Personalized shopping experiences powered by AI analyze customer behavior across multiple touchpoints, delivering tailored recommendations and engaging chatbot interactions that increase conversion rates and customer loyalty.
Fraud detection systems monitor transactions in real-time, identifying suspicious patterns while AI-powered dynamic pricing strategies adjust prices based on demand, competition, and other market factors to maximize profitability.
c. Healthcare
Healthcare organizations are implementing AI to improve patient outcomes and operational efficiency. AI in medical diagnostics analyzes imaging studies and patient data to identify anomalies that might be missed by human clinicians, reducing diagnostic errors by 30-40%.
Automated patient scheduling systems optimize appointment slots based on historical data and resource availability, reducing wait times and improving facility utilization. AI-powered drug discovery accelerates the development process by identifying potential compounds and predicting trial outcomes, cutting research timelines and costs significantly while bringing treatments to market faster.
d. Finance & Banking
The financial sector has been an early adopter of AI for operational efficiency. AI-powered fraud detection systems analyze millions of transactions daily, identifying patterns indicative of fraudulent activity with greater accuracy than traditional rule-based systems.
Algorithmic trading platforms execute complex strategies at speeds impossible for human traders, optimizing portfolio performance based on real-time market data. Intelligent customer support systems provide 24/7 assistance through AI chatbots that resolve routine inquiries instantly, escalating complex issues to human agents when necessary while maintaining consistent service quality.
e. Logistics & Supply Chain
Logistics companies leverage AI to optimize their complex operations. AI for route optimization analyzes traffic patterns, weather conditions, and delivery windows to create the most efficient delivery paths, reducing fuel consumption and operational costs.
Demand forecasting in logistics helps companies anticipate shipping volumes and adjust resources accordingly, while inventory management systems track products across the supply chain, ensuring optimal stock levels at all points. AI in warehouse automation employs robotic systems that pick, pack, and ship products with minimal human intervention, increasing throughput and reducing errors.
f. IT & Cybersecurity
IT departments and cybersecurity firms depend on AI to manage growing complexity and threats. AI for threat detection analyzes network traffic and system behavior to identify potential security incidents before they cause damage. Automated incident response systems contain threats rapidly by isolating affected systems and initiating remediation protocols.
AI-driven data management optimizes storage, backup, and retrieval processes while cloud optimization tools analyze usage patterns to recommend the most cost-effective resource configurations.
g. Energy & Utilities
The energy sector implements AI to improve sustainability and efficiency. Smart grid optimization balances energy supply and demand in real-time, reducing waste and preventing outages. AI-powered predictive maintenance in power plants analyzes equipment performance data to schedule repairs before failures occur, extending asset life and reducing maintenance costs.
AI in renewable energy efficiency optimizes the performance of solar and wind installations, predicting generation capacity based on weather patterns and adjusting systems to maximize output while integrating with traditional energy sources seamlessly.
5. Challenges of Implementing AI for Operational Efficiency
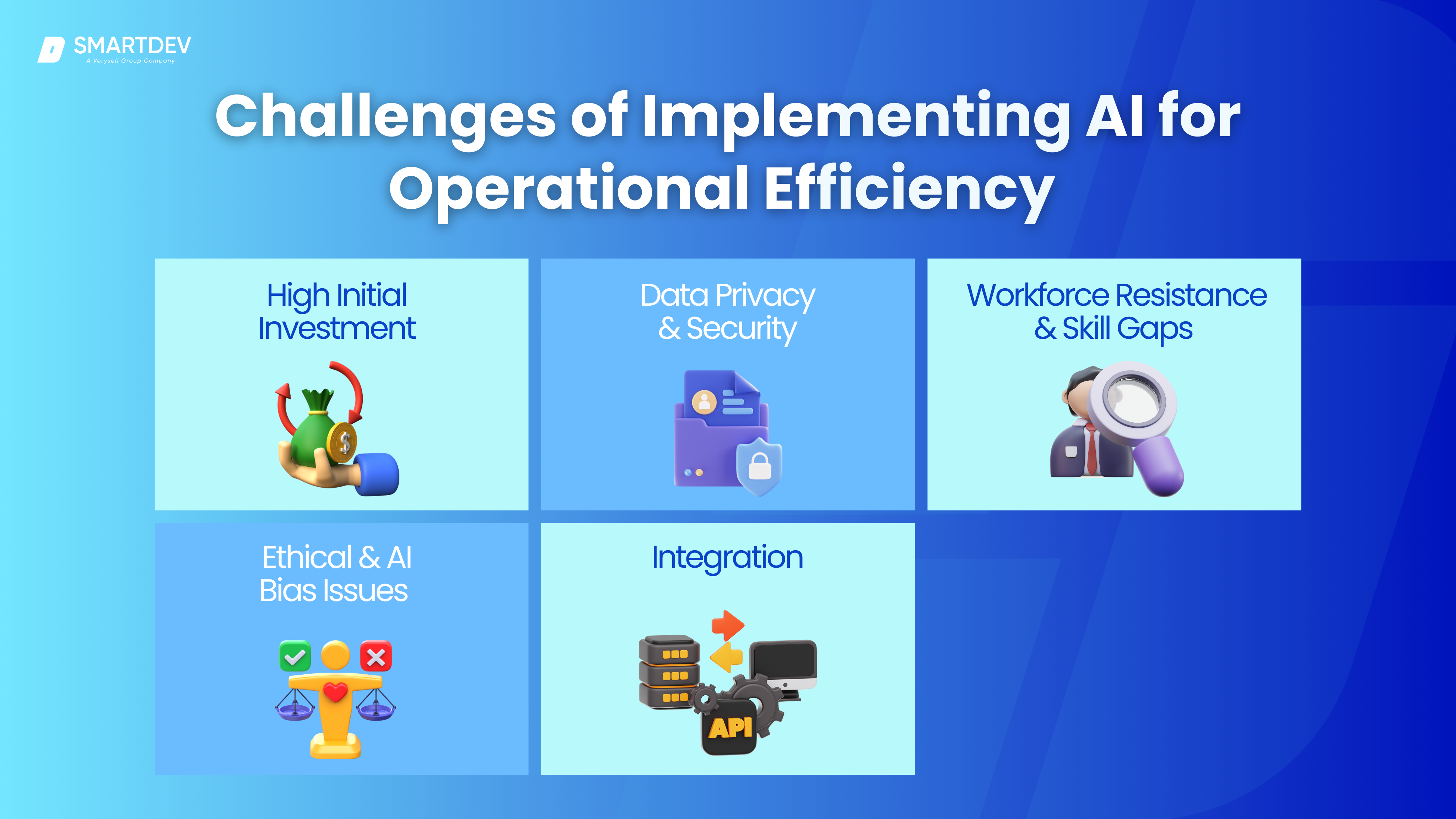
a. High Initial Investment & Implementation Costs
While cloud-based AI services have reduced barriers to entry, comprehensive AI implementations still require significant investment. Organizations must consider not just software costs but also data preparation, integration, training, and change management expenses.
Cost Mitigation Strategy: Many organizations begin with “AI as a Service” models, leveraging pre-trained models and pay-as-you-go pricing to minimize upfront investment. This approach allows companies to demonstrate value before committing to larger investments.
b. Data Privacy & Security Concerns
AI systems require access to sensitive operational data, creating potential vulnerabilities. Organizations must implement robust security frameworks that address data in transit, at rest, and in use.
Best Practice: Adopt a “privacy by design” approach, incorporating data protection measures from the earliest stages of AI development. This includes data anonymization techniques, encryption, and strict access controls.
c. Workforce Resistance & Skill Gaps
Employees may resist AI adoption due to fear of job displacement or lack of understanding. Additionally, a shortage of AI skills creates implementation challenges.
Change Management Framework: Successful AI implementations follow a three-phase approach: awareness building, skill development, and incentive realignment. Organizations should identify “AI champions” within business units to lead adoption efforts.
d. Ethical Considerations & AI Bias Issues
AI algorithms can perpetuate biases present in training data, leading to unfair outcomes. Organizations must establish ethical guidelines and regularly audit AI systems to ensure fairness and transparency.
Implementation Tip: Create cross-functional AI ethics committees that include representatives from legal, HR, operations, and IT to oversee AI development and deployment.
e. Integration with Legacy Systems
Many businesses operate with outdated systems that lack compatibility with modern AI technologies. This creates integration challenges that can delay implementations.
Modernization Approach: Organizations should prioritize creating API layers that allow legacy systems to communicate with modern AI platforms without requiring full system replacement. This “middle-out” strategy balances cost considerations with functionality needs.
6. AI-Powered Strategies for Boosting Operational Efficiency
a. Intelligent Process Automation (IPA)
IPA combines the rule-based automation of RPA with the cognitive capabilities of AI, creating systems that can handle complex, knowledge-based tasks. Unlike traditional automation, IPA systems can adapt to changing conditions and improve over time through machine learning.
Organizations should begin with high-volume, repetitive processes that have clear decision criteria. As capabilities mature, expand to more complex workflows requiring contextual understanding and judgment.
b. Digital Twin Technology for Business Operations
Digital twins create virtual replicas of physical processes, enabling simulation and testing of operational changes without disrupting live systems. This technology allows organizations to experiment with process improvements, predict outcomes, and optimize before implementation.
Some manufacturers now maintain digital twins for individual machines throughout their lifecycle, using them to train service technicians, predict maintenance needs, and even design next-generation equipment.
c. AI in Business Intelligence (BI) for Data-Driven Decisions
AI-enhanced BI tools provide actionable insights through advanced analytics and visualization. These systems can automatically generate reports, identify trends, and even suggest strategic actions based on predictive models.
The most effective AI-BI implementations focus on specific business questions rather than attempting to analyze all data at once. This “question-first” approach ensures that analytics efforts deliver immediate business value.
d. Human-AI Collaboration: Augmenting Workforce Productivity
The most powerful AI implementations don’t replace humans but augment their capabilities. By handling routine tasks and providing decision support, AI enables employees to work at higher levels of complexity and creativity.
Effective human-AI collaboration requires clear role definition, transparent decision-making processes, and continuous feedback loops where humans can refine AI outputs.
e. AI-powered Predictive & Prescriptive Analytics
While predictive analytics forecasts future outcomes, prescriptive analytics recommends optimal actions. Together, they enable proactive decision-making and strategic planning across the organization.
Some organizations now implement “explanation-aware” AI systems that not only predict outcomes but also provide understandable rationales for their recommendations, increasing adoption rates among business users.
7. AI in Operational Efficiency: Case Studies & Real-World Examples
In this section, we delve into real-world examples where leading companies have harnessed Artificial Intelligence (AI) to enhance operational efficiency. These case studies illustrate the transformative power of AI across various industries.
Tesla’s AI-driven Manufacturing Process
Tesla has integrated AI into its manufacturing operations to optimize production and improve efficiency. A cornerstone of this integration is the development of the Dojo supercomputer, designed to enhance the training of AI models for autonomous driving and other applications. The Dojo system features the D1 chip, which contains 50 billion transistors and is manufactured using 7-nanometer technology. This chip architecture allows Tesla to process vast amounts of data more efficiently, leading to improvements in both vehicle performance and manufacturing processes.
Key Points:
- Custom AI Hardware: Development of the D1 chip tailored for high-performance AI computations.
- Enhanced Data Processing: Ability to process large datasets efficiently, aiding in real-time decision-making.
- Scalability: Modular design facilitating expansion to meet growing computational demands.
Amazon’s AI-powered Supply Chain & Logistics
Amazon has extensively implemented AI to streamline its supply chain and logistics, leading to significant cost reductions and efficiency gains. The company has deployed over 750,000 mobile robots and numerous robotic arms across its fulfillment centers. These robots perform tasks such as heavy lifting and package sorting, which has resulted in a 25% reduction in order fulfillment costs. Additionally, Amazon’s AI-driven inventory management system accurately predicts product demand, optimizing stock levels and reducing waste.
Key Points:
- Robotic Automation: Extensive use of robots to handle repetitive tasks, enhancing efficiency.
- Predictive Analytics: AI models forecasting product demand to optimize inventory and reduce waste.
- Cost Reduction: Achieved significant savings in fulfillment operations through automation.
AI in Financial Fraud Prevention at JPMorgan Chase
JPMorgan Chase has integrated AI into its operations to enhance payment efficiency and reduce fraud. The bank employs AI-powered models for payment validation screening, which has led to a 15-20% reduction in account validation rejection rates. These models analyze vast amounts of transaction data in real-time to identify anomalies and potential fraudulent activities, thereby improving customer experience and operational efficiency.
Key Points:
- Real-Time Analysis: AI systems capable of analyzing transactions instantly to detect fraud.
- Adaptive Learning: Continuous improvement of fraud detection accuracy through machine learning.
- Enhanced Security: Strengthened measures to protect customer assets and maintain trust.
Google’s AI-driven Data Center Energy Efficiency
Google has utilized AI to enhance the energy efficiency of its data centers. By implementing AI systems to monitor and adjust cooling mechanisms, Google has achieved significant reductions in energy consumption. These AI-driven adjustments have led to more sustainable operations and cost savings, aligning with the company’s environmental goals.
Key Points:
- Dynamic Optimization: AI systems that continuously adjust cooling settings for optimal performance.
- Energy Savings: Substantial reduction in energy usage, contributing to sustainability efforts.
- Scalability: AI solutions that can be applied across multiple data centers globally.
AI-powered Healthcare Operations at Mayo Clinic
Mayo Clinic has established an “AI Factory” to integrate artificial intelligence into its healthcare services. This initiative focuses on enhancing medical diagnostics, predictive patient care, and efficient resource allocation. By leveraging AI, Mayo Clinic aims to improve patient outcomes and operational efficiency.
Key Points:
- Diagnostic Enhancement: AI tools assisting in accurate and swift medical diagnoses.
- Predictive Care: Utilizing AI to anticipate patient needs and personalize treatment plans.
- Operational Efficiency: Streamlining hospital operations through data-driven decision-making.
These case studies exemplify the diverse applications of AI in enhancing operational efficiency across various sectors. By adopting AI technologies, these organizations have achieved significant improvements in productivity, cost reduction, and service quality.
8. How to Implement AI for Operational Efficiency in Your Business
Successfully implementing AI to enhance operational efficiency requires a strategic approach that aligns technology with business objectives. Here’s a comprehensive guide to help organizations navigate this transformation:
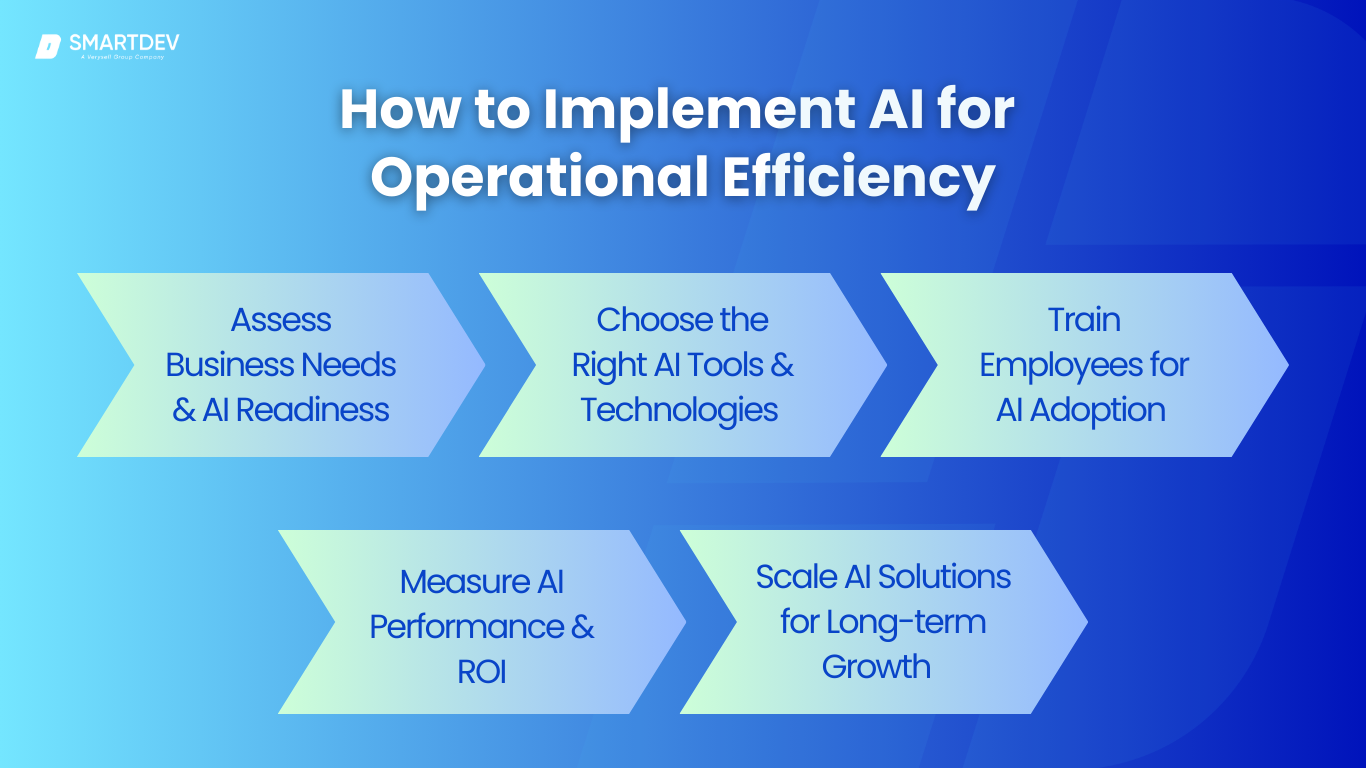
a. Assessing Business Needs & AI Readiness
Begin by conducting a thorough assessment of your organization’s operational landscape. Identify pain points by mapping your business processes to pinpoint areas with high costs, frequent errors, bottlenecks, or slow cycle times—these are prime candidates for AI intervention. Evaluate your data infrastructure by inventorying your data assets and assessing their quality, accessibility, and governance. Remember, AI requires clean, relevant data; without it, even the most sophisticated models will underperform.
Finally, assess your organizational culture to determine your team’s readiness for AI adoption. Identify champions within your organization who can help drive the transformation and address resistance.
b. Choosing the Right AI Tools & Technologies
Select solutions that address your specific needs by matching tools to use cases. For example, predictive maintenance requires computer vision and IoT sensors, while customer service automation needs NLP-powered chatbots. Evaluate vendor capabilities beyond marketing claims to assess actual performance, integration capabilities, and vendor support. Consider implementation complexity by balancing between off-the-shelf solutions and custom development based on your technical capacity and budget.
Subsequently, AI depends on robust data foundations. Start with a data inventory and quality assessment, documenting all data sources and evaluating their relevance, accuracy, and completeness. Establish data governance with policies for data collection, storage, security, and usage. Build data pipelines that create efficient pathways for data to flow from sources to AI models, ensuring timely availability.
c. Training Employees for AI Adoption
Successful AI implementation requires organizational adaptation. Address workforce concerns by proactively communicating how AI will augment rather than replace roles. Involve employees in the selection and design process. Build AI literacy with training tailored to different roles—technical teams need development skills while business users require understanding of AI capabilities and limitations. Create implementation roadmaps with phased plans and clear milestones, starting with quick wins to demonstrate value.
d. Measuring AI Performance & ROI
Approach implementation methodically by selecting pilot projects with clear metrics for success, high impact potential, and manageable scope. Establish KPIs to define measurable outcomes such as processing time reduction, accuracy improvement, or cost savings. After successful pilots, scale strategically by expanding to related processes while documenting best practices.
e. Scaling AI Solutions for Long-term Growth
AI implementation is not a one-time project. Monitor performance by tracking AI systems against KPIs and adjust models as business conditions change. Update models regularly with new data to maintain accuracy and relevance. Continuously scan operations for additional areas where AI can create value.
9. Future of AI in Operational Efficiency
a. Proliferation of Autonomous AI Agents
Autonomous AI agents are expected to become integral components of business operations, handling tasks ranging from customer service to complex decision-making processes. These agents will operate with increasing independence, learning and adapting to new information without constant human intervention. By 2035, AI agents are projected to increase overall business profitability by approximately 38%, highlighting their substantial impact on operational efficiency.
b. Mainstream Adoption of Generative AI
Generative AI, capable of creating new content such as text, images, and music, is set to revolutionize creative processes within businesses. By 2026, it is anticipated that 75% of businesses will utilize generative AI to produce synthetic customer data, a significant increase from less than 5% in 2023. This technology will enable personalized customer interactions and innovative product development, thereby enhancing operational efficiency.
c. Integration of AI with Blockchain Technology
The convergence of AI and blockchain is expected to transform transaction management by offering unprecedented security, efficiency, and transparency. This integration will streamline operations, bolster stakeholder trust, and unlock new business opportunities, thereby enhancing operational efficiency.
d. Enhanced Human-AI Collaboration
The future will witness a deeper collaboration between humans and AI, with AI systems augmenting human capabilities rather than replacing them. This synergy will lead to more efficient workflows, improved decision-making, and enhanced productivity across various industries.
e. Emergence of Physical AI Agents
Physical AI agents, which combine cognitive intelligence with real-world actions, are anticipated to revolutionize industries such as manufacturing, healthcare, and logistics. These agents will interact directly with physical environments, performing tasks with precision and adaptability, thus reducing human error and enhancing operational efficiency.
10. Conclusion
AI represents a transformative force in operational efficiency, offering businesses unprecedented opportunities to enhance productivity, reduce costs, and deliver superior customer experiences. The organizations that thrive in this AI-augmented future will be those that view AI not as a replacement for human intelligence but as a powerful extension of it.
Actionable Steps for Businesses to Leverage AI
- Identify High-Impact Use Cases: Focus on areas with clear operational inefficiencies and measurable ROI potential. Look for processes with high volume, clear decision criteria, and significant downstream impact.
- Invest in Data Infrastructure: Ensure robust data collection, storage, and governance frameworks. Remember that AI is only as good as the data that feeds it—garbage in, garbage out
- Collaborate with AI Experts: Partner with technology providers and consultancies to accelerate implementation. Few organizations possess all the necessary expertise in-house.
- Foster a Culture of Innovation: Encourage experimentation and learning to drive continuous improvement. Create innovation labs or AI centers of excellence to coordinate efforts across business units.
Final Thoughts & Future Considerations
As AI continues to evolve, businesses must adopt a proactive stance to remain competitive. By embracing ethical practices, investing in talent, and staying attuned to technological advancements, organizations can harness AI’s full potential to achieve sustainable operational excellence in an increasingly dynamic global landscape. The companies that will lead tomorrow are those that begin building their AI capabilities today, not just as a technology implementation but as a fundamental transformation of how they create value.
References
- The Future of AI Agents: Transformative Trends and Predictions 2025-2030 | Alex Velinov
- Top AI Development Trends for 2025-2030 | Techstack
- Top 10 AI Trends to Watch in 2025 for Businesses | Rapidops
- Physical AI Agents: Integrating Cognitive Intelligence with Real-World Action | Cornell University
- Five Trends in AI and Data Science for 2025 | MIT Sloan Review
- Amazon bets savings from automation can help fuel AI spending boom | Financial Times
- AI for operational efficiency: Use cases, benefits, implementation, technologies and development | LeewayHertz
- AI for Operational Efficiency: Simplify Business Operations | CallHippo
- AI in Everyday Use Success Stories & Industry-specific AI Case Studies | Rapid Innovation