The rapid evolution of artificial intelligence (AI) has significantly transformed how businesses operate, enabling automation, data analysis, customer insights, and personalized experiences.
By 2025, AI is expected to be more deeply integrated into various sectors, shaping industries ranging from healthcare to retail, finance, and beyond. However, for businesses considering AI adoption, understanding the costs associated with AI development is crucial for planning, budgeting, and making informed decisions.
1. What Is AI Development and Why Is It Important in 2025?
AI development involves the creation and implementation of algorithms, machine learning models, and data systems that allow machines to perform tasks typically requiring human intelligence. These tasks include language processing, decision-making, problem-solving, and predictive analytics. The goal of AI development is not just to automate processes, but to enhance the ability of businesses to scale, innovate, and deliver superior products and services.
As we approach 2025, AI’s importance continues to grow. Companies like Google are already integrating AI into their core products, from Google Search to Google Assistant, to improve user experiences and streamline business processes. Google’s continuous investment in AI technologies, including its powerful machine learning models, demonstrates how AI can revolutionize business operations and consumer engagement. According to PwC (2020), AI is expected to contribute $15.7 trillion to the global economy by 2030, showing its immense economic potential across sectors.
1.1 .Why Understanding AI Development Costs Matters for Businesses
While the benefits of AI are clear, understanding its development costs is vital for businesses aiming to implement AI technologies. The costs of AI development can vary depending on several factors, including the complexity of the project, the size of the dataset, and the development timeline.
For example, the cost of developing an AI-powered app can range from $50,000 to $500,000, depending on the complexity of the app’s features (Future Processing, 2024). AI-driven applications such as those used in customer service, inventory management, or personalized marketing often require significant investment in both human resources and infrastructure. Tesla, known for its AI-driven self-driving technology, has invested heavily in machine learning to power its autonomous vehicles, with estimated development costs running into the millions. This level of investment is required to manage the massive datasets used to train Tesla’s AI models for real-time driving decisions.
Understanding these costs helps businesses decide whether to develop AI in-house or partner with AI agencies. For example, IBM Watson Health’s investment in AI for healthcare illustrates how major corporations are deploying AI to improve efficiency and outcomes. IBM has committed significant resources to develop AI that assists doctors in diagnosing and treating medical conditions, showing how large-scale AI adoption can lead to new service offerings and business models.
1.2. Overview of AI Development Cost Influences
The cost of AI development is influenced by several factors that businesses must consider before embarking on an AI project. Key factors include:
- Complexity of the AI Model: More complex models, such as those involving deep learning or large-scale machine learning, require more specialized expertise and resources, thus increasing costs.
- Data Requirements: AI models need large volumes of high-quality, labeled data to train effectively. Collecting and processing this data can be time-consuming and expensive.
- Talent Acquisition: Skilled professionals, such as data scientists, machine learning engineers, and AI researchers, are in high demand, and hiring such talent comes at a premium.
- Technology Infrastructure: High-performance computing resources, whether on-premises or cloud-based, are necessary to process the large datasets AI models require. These can be costly, especially when scaling.
- Integration and Maintenance: Integrating AI into existing business systems and continuously maintaining the models to ensure they evolve with changing data also adds to the cost.
By understanding these influences, businesses can better estimate the costs of AI development and make strategic decisions regarding their AI initiatives. Recognizing these variables upfront can help businesses allocate resources more effectively and manage expectations throughout the development process.
Overall, the importance of understanding AI development costs cannot be overstated. With AI poised to be a driving force in business transformation by 2025, companies that are well-prepared for the financial commitments involved will be better positioned to succeed in the rapidly evolving digital landscape.
2. The Basics of AI Development Costs
Understanding the cost structure of AI development is key for businesses considering AI adoption. Several elements contribute to the overall cost, and each has a different impact depending on the size and scope of the project. The costs involved in developing AI are not limited to just the development phase; they extend throughout the project lifecycle, from planning and building to deployment and ongoing maintenance.
2.1. What Goes into the Cost of AI Development?
The cost of AI development can be divided into several critical components, each with its own implications for businesses:
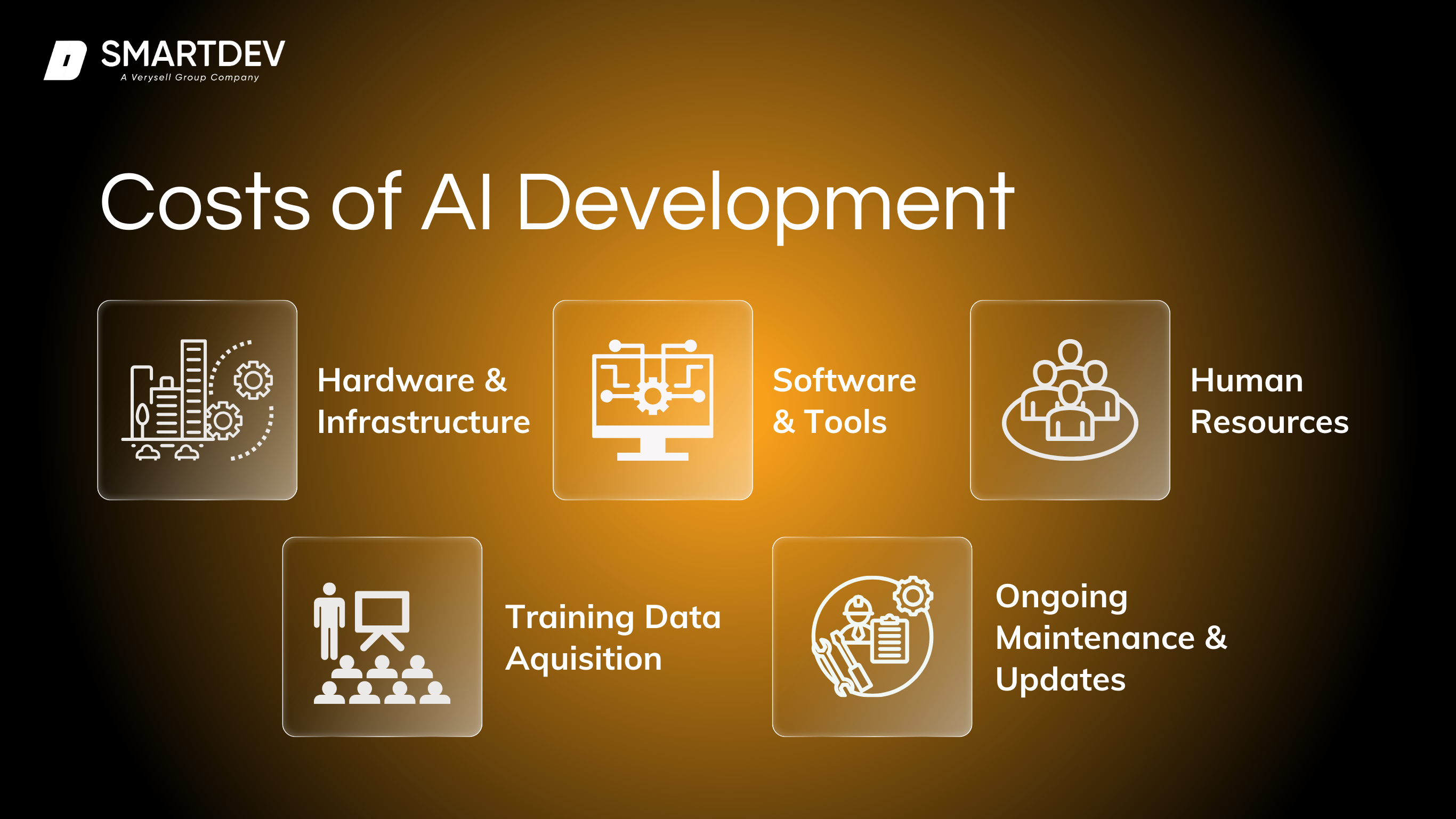
Hardware and Infrastructure
AI systems require significant computational power, especially for deep learning models, which need to process large datasets in real-time. The infrastructure involved in AI development includes hardware such as GPUs, TPUs, and other high-performance processors. Cloud-based services such as Amazon Web Services (AWS), Google Cloud, and Microsoft Azure also offer the necessary computing power to run AI algorithms.
The cost of infrastructure can vary based on the scale of the AI system. For instance, a small-scale AI application might require only basic cloud services, whereas an enterprise-grade AI system could require dedicated servers or specialized hardware.
Software and Tools
AI development also requires specialized software tools and frameworks. Some of the commonly used tools include TensorFlow, PyTorch, and Keras, which are open-source frameworks for building machine learning models. However, businesses might also opt for proprietary software, which can carry significant licensing fees. Additionally, businesses may require data storage solutions and databases like SQL, NoSQL, or cloud storage services.
While open-source tools are often free, the integration, customization, and scaling of AI projects can lead to additional software costs. Companies that build large-scale AI systems will often need customized tools tailored to their unique business needs, increasing the overall expenditure.
Human Resources
One of the largest contributors to the cost of AI development is human resources. AI projects require specialized talent, including AI developers, data scientists, machine learning engineers, and data analysts. According to the World Economic Forum’s Job Report in 2020, data science and AI roles are among the highest-paid in the tech industry, with experienced professionals commanding salaries of $120,000 to $300,000 or more annually.
The size of the team needed depends on the scale of the AI project. Small-scale applications may require only a handful of developers, while enterprise-grade AI solutions could involve large, multi-disciplinary teams. Hiring costs are especially high in competitive markets where talent shortages exist.
Training Data Acquisition
AI models learn by being trained on data, and obtaining high-quality data is both time-consuming and expensive. For businesses developing AI applications, acquiring training data can involve gathering and cleaning large datasets, which may require significant investment in data collection or purchasing datasets from third-party providers.
Additionally, businesses must ensure that the data is properly labeled and structured, a process known as data annotation, which can add further costs. For example, self-driving car companies like Waymo must collect vast amounts of sensor data, including images, videos, and LIDAR data, to train their autonomous driving systems.
Ongoing Maintenance and Updates
AI systems require continuous updates and retraining to adapt to new data and changing conditions. This ongoing maintenance ensures that AI systems remain relevant and effective. Maintenance costs include fine-tuning the models, fixing bugs, updating software, and expanding the system to accommodate new functionalities or integrate with other business systems.
For example, Google continually updates its search algorithms and AI-driven tools to improve user experience and search result accuracy (Kevin, 2024). These updates require an ongoing investment of both time and resources.
2.2. Average Cost Ranges for AI Development Projects
The cost of AI development can vary widely depending on the complexity and scale of the project:
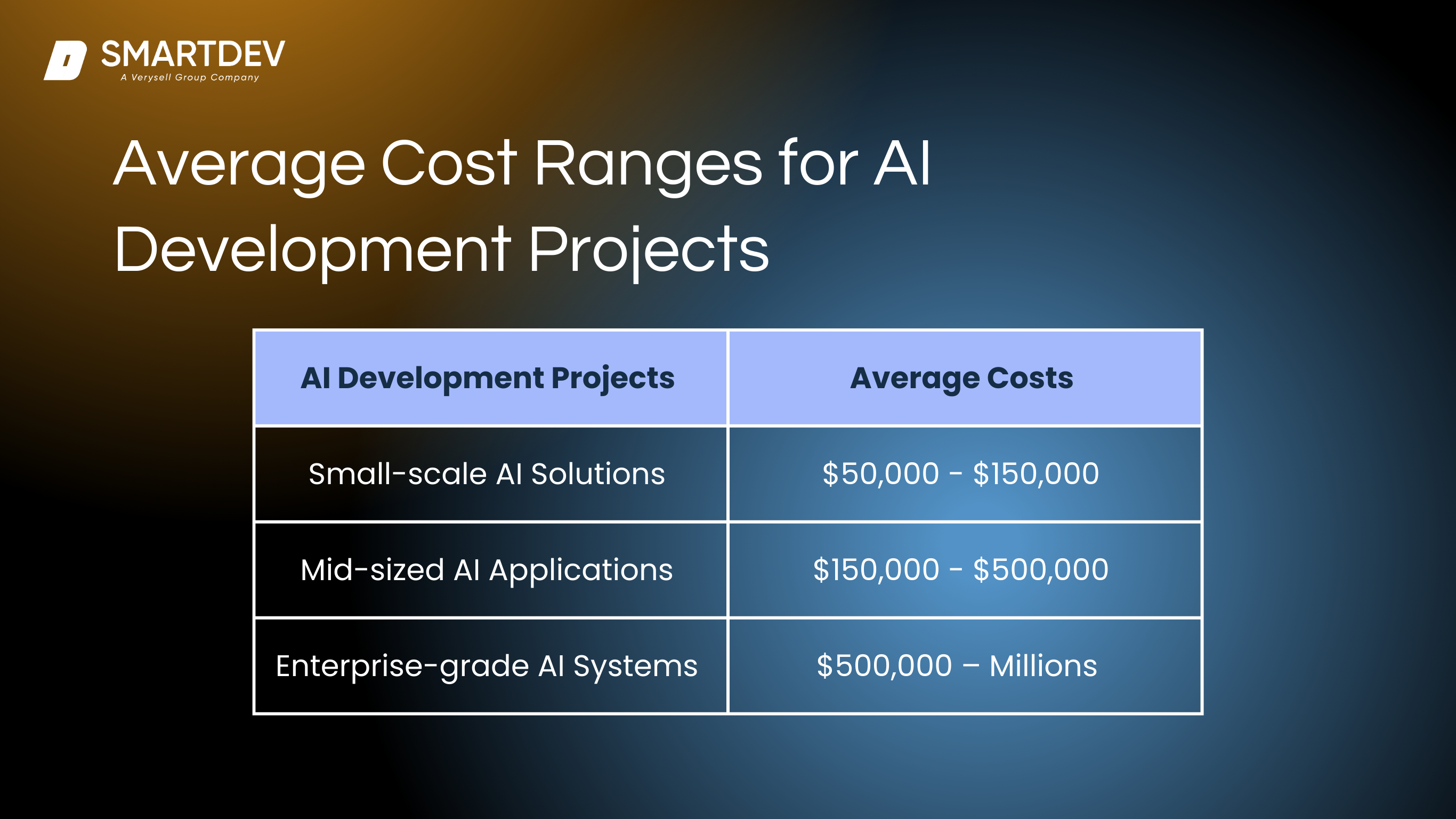
Small-Scale AI Solutions
For businesses that want to develop relatively simple AI applications, such as chatbots, recommendation engines, or image recognition tools, the cost typically ranges from $50,000 to $150,000. These solutions may require basic machine learning algorithms, and the data involved is often smaller in scale compared to larger projects. Many companies will opt to work with third-party development firms or AI agencies, which can provide these services at a more affordable price.
- Example: A small business developing a chatbot for customer support might spend between $50,000 and $100,000 depending on the complexity of the chatbot’s capabilities and integration into existing systems.
Mid-Sized AI Applications
Mid-sized AI applications, which often involve more complex machine learning models or AI integrations into business workflows, tend to cost between $150,000 and $500,000. Examples of these include AI solutions for marketing automation, predictive analytics, or inventory management. At this scale, businesses might need a team of several data scientists and engineers to implement the solution effectively.
- Example: An e-commerce company integrating personalized product recommendations or demand forecasting into its platform could expect to spend around $250,000.
Enterprise-Grade AI Systems
At the enterprise level, AI systems are larger, more sophisticated, and often mission critical. Developing these systems can cost anywhere from $500,000 to several million dollars. These projects typically involve deep learning models, massive data sets, and integration into core business processes, such as AI for autonomous vehicles, large-scale fraud detection, or enterprise resource planning (ERP) systems powered by AI.
For instance, Tesla’s self-driving technology, which requires massive amounts of data and highly specialized machine learning models, is an example of an enterprise-grade AI project that runs into the millions of dollars in development costs (Agam, 2023)
In summary, businesses must carefully consider these costs when planning for AI development. Whether the project is small or large, AI can drive significant value, but it comes with considerable financial commitment. By understanding the core elements of AI development costs—hardware, software, human resources, data acquisition, and ongoing maintenance—companies can more effectively budget and allocate resources to maximize the return on their investment in AI.
3. Key Factors Influencing AI Development Costs
The cost of developing AI solutions is not a one-size-fits-all equation. A variety of factors, including the scope of the project, the type of AI application, and the development team’s composition, all play a significant role in determining the final cost.
3.1. Scope and Complexity of the AI Solution
The scope and complexity of an AI project are among the most critical factors affecting its cost. A project’s scope determines the scale and intricacy of the AI solution, with costs rising proportionally to complexity.
- Simple Predictive Models vs. Complex Neural Networks
Developing a simple predictive model for tasks like sales forecasting might cost significantly less than creating a deep learning-based neural network for applications like image recognition or autonomous driving. Simple models are faster to develop and require fewer resources, while advanced neural networks demand more data, computational power, and expertise. For example, Tesla’s self-driving AI, built on complex neural networks, reflects the high end of AI development costs.
- Custom Development vs. Off-the-Shelf Solutions
Custom AI development allows businesses to tailor solutions to their unique needs but is generally more expensive than off-the-shelf tools. Companies like IBM Watson offer pre-built AI platforms that can be customized, reducing development time and cost compared to entirely bespoke solutions.
3.2. Types of AI Application
The type of AI applications significantly impacts costs, as different applications require varying levels of expertise, computational power, and development time.
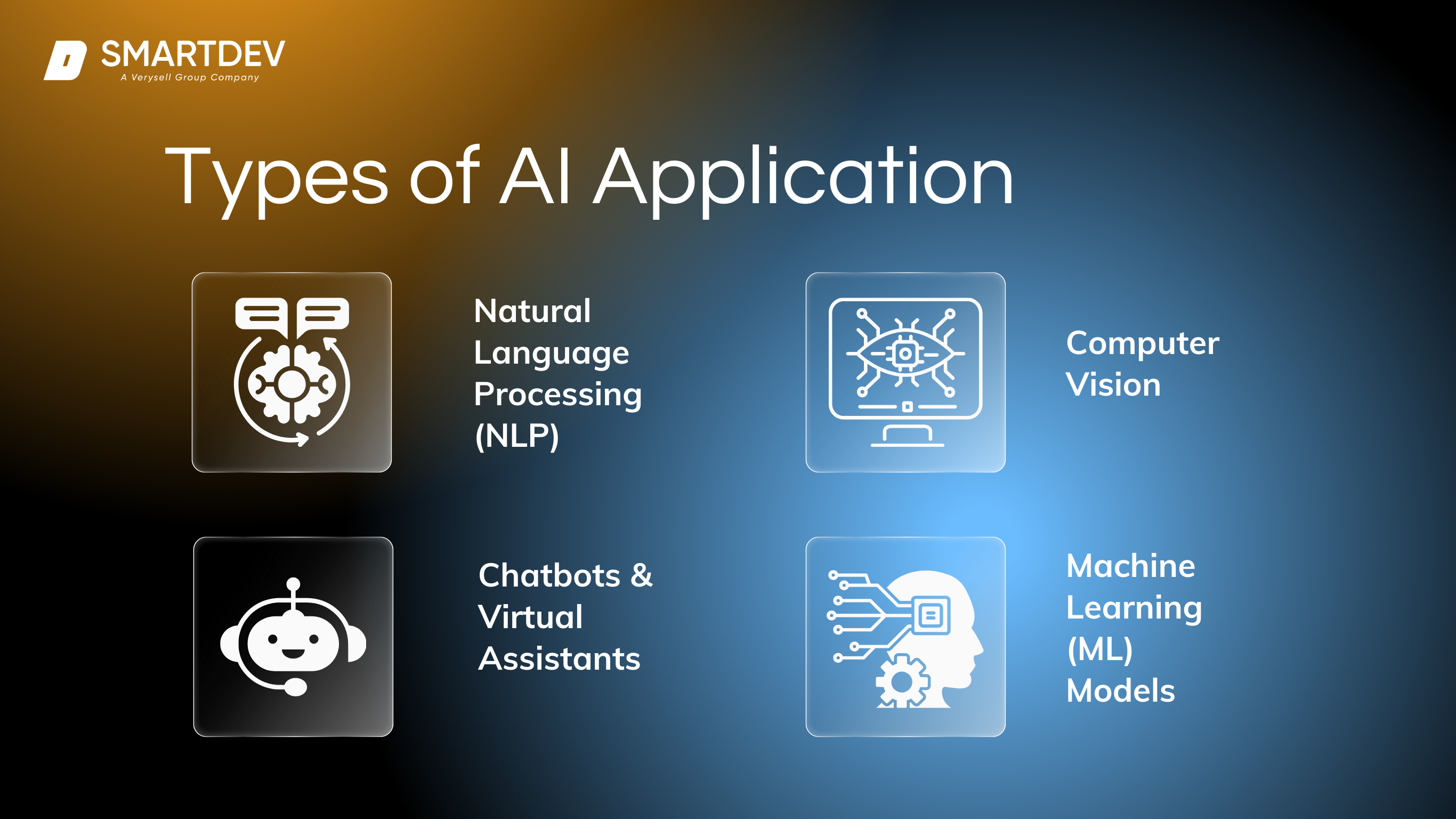
Natural Language Processing (NLP)
NLP applications, such as chatbots or language translation tools, often involve deep learning models and large datasets. A chatbot for customer service might cost between $50,000 and $200,000, depending on complexity.
Computer Vision
AI systems for tasks like facial recognition or object detection require substantial computational resources and high-quality datasets. For example, developing AI for autonomous drones involves significant hardware and software investment.
Machine Learning (ML) Models
General ML models, such as those used for fraud detection or personalized recommendations, vary in cost depending on their scale and complexity. Companies like Amazon, which use ML to power their recommendation engines, invest heavily in data and infrastructure.
Chatbots and Virtual Assistants
Simple chatbots may cost under $50,000, but advanced virtual assistants, such as Google Assistant or Siri, can require budgets exceeding $500,000.
Read more: Chatbots vs. Virtual Assistants: Which AI Solution is Right for Your Business?
3.3. Team Composition and Location
The cost of AI development also depends on the structure and location of the development team.
- In-House vs. Outsourced Development: Building an in-house team provides greater control but comes with higher costs, including salaries, benefits, and infrastructure. Outsourcing to specialized firms can reduce costs and allow businesses to access expert talent on a project basis.
- Cost Differences by Region: Geographic location plays a significant role in determining costs. In regions like the USA, hourly rates for AI developers can exceed $150, while rates in Europe and Asia are often significantly lower. For instance, outsourcing to countries like India or Ukraine can cost between $30 and $70 per hour.
3.4. Tools and Technologies Used
The choice of tools and technologies in AI development significantly impacts overall costs. Each option presents unique trade-offs in terms of upfront costs, development time, and resource requirements.
- Open-Source Frameworks
Open-source frameworks such as TensorFlow, PyTorch, and Keras are popular choices for AI development due to their flexibility, community support, and zero licensing costs. These tools provide robust capabilities for building machine-learning models and are widely adopted by both startups and tech giants.
However, their effective implementation often requires experienced developers and data scientists, which can increase labor costs. Additionally, the time required for customization and integration into existing systems can add to the overall project expenses.
- Proprietary Tools
Proprietary platforms like IBM Watson, Amazon SageMaker, and Microsoft Azure AI come with licensing fees but offer pre-built functionalities designed to streamline the development process. These tools are especially valuable for businesses aiming to reduce time-to-market, as they provide ready-made infrastructure and simplified workflows. While the upfront costs may be higher, the savings in development time and reduced need for technical expertise can offset these expenses, particularly for organizations without extensive in-house AI capabilities.
By carefully evaluating their project needs, businesses can select the tools and technologies that best align with their goals and budget, ensuring an efficient and cost-effective development process.
4. Detailed Breakdown of AI Development Costs
Understanding the AI development cost is essential for businesses aiming to implement artificial intelligence solutions effectively. Below is a detailed analysis of the key stages and their associated costs:
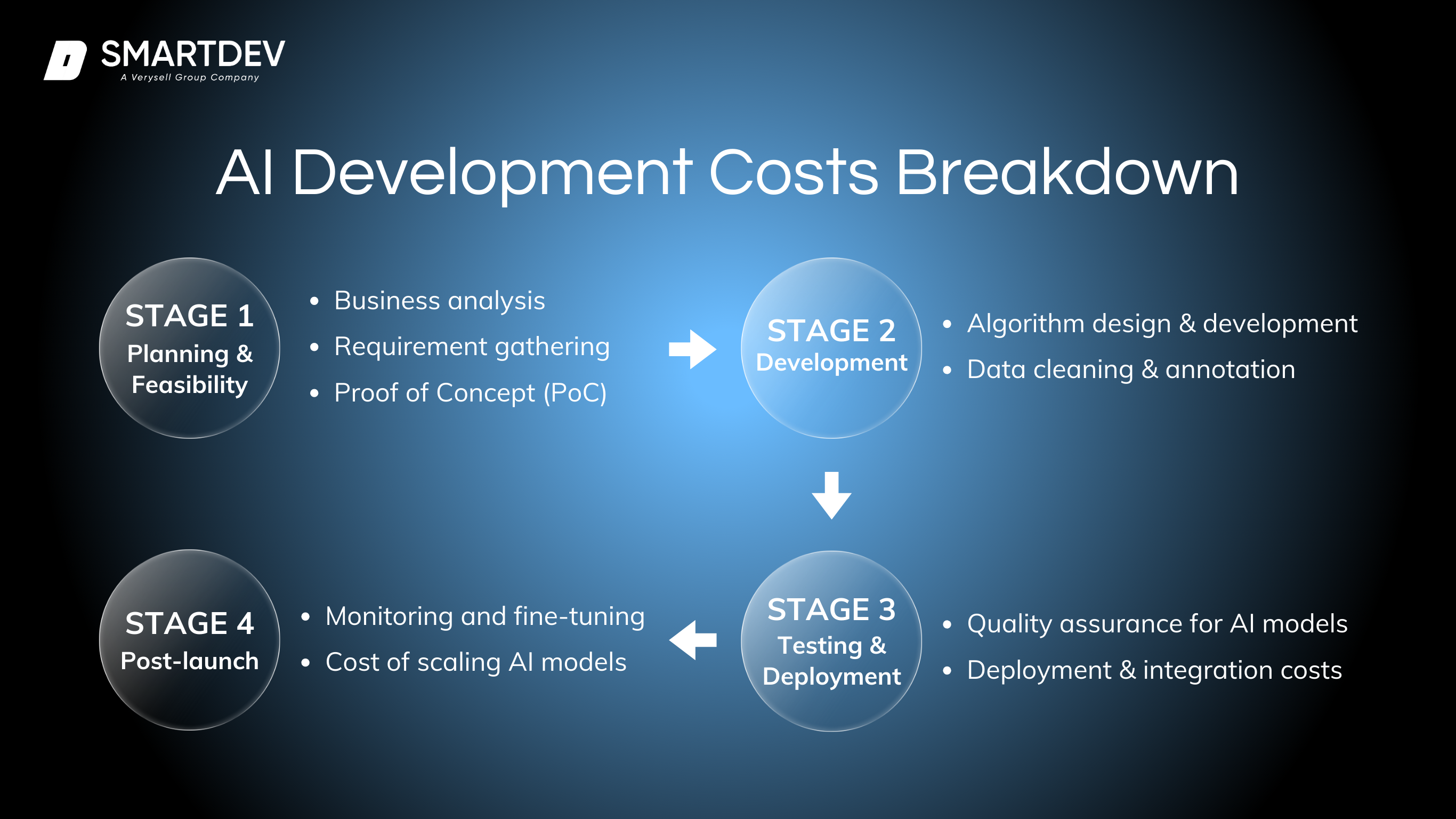
4.1. Planning and Feasibility Stage
Business Analysis: This task focuses on identifying the business problems that AI can solve, defining the project’s objectives, and estimating the expected ROI. It involves consulting with stakeholders to establish clear goals and evaluating the alignment of AI initiatives with business strategies.
- Tasks Involved: Stakeholder meetings, requirement documentation, feasibility studies.
- Costs Consideration: Consulting fees for business analysts and AI experts, tools for requirement gathering like Confluence or Jira.
Proof of Concept (PoC): The PoC validates the technical feasibility and practicality of the AI project before full-scale development. It includes creating prototypes or limited functional models to test the core idea.
- Tasks Involved: Prototype development, technical feasibility testing, performance evaluations.
- Costs Consideration: Salaries for developers and designers, cloud services for testing, and third-party tools for prototyping. PoC costs typically range from $5,000 to $20,000 depending on complexity.
4.2. Development Stage
Algorithm Design and Development: Custom algorithms tailored to specific business needs are created during this phase. These algorithms require significant effort in coding, testing, and optimization to achieve the desired functionality and efficiency.
- Tasks Involved: Algorithm creation, model training, and fine-tuning.
- Costs Required: Salaries for AI engineers and data scientists, licensing fees for AI frameworks like TensorFlow or PyTorch.
Data Cleaning and Annotation: Clean and annotated datasets are vital for the accuracy and reliability of AI models. This process involves removing inconsistencies from raw data and labeling data for supervised learning tasks.
- Tasks Involved: Data preprocessing, annotation of images, text, or other formats, quality checks.
- Costs Required: Hiring annotators or using annotation tools, cloud storage for large datasets. Costs for data annotation can range between $10,000 and $50,000 based on data volume.
4.3. Testing and Deployment Stage
Quality Assurance (QA) for AI Models: Testing ensures the AI system meets performance benchmarks and functions as expected under different scenarios. This includes unit testing, stress testing, and performance optimization.
- Tasks Involved: Component testing, stress tests, debugging, performance evaluations.
- Costs Consideration: Salaries for QA testers skilled in AI, automated testing tools like Apache JMeter or Selenium.
Deployment and Integration Costs: Integrating the AI model into existing IT infrastructures and deploying it for real-world use. This stage also involves setting up monitoring systems to ensure seamless operation.
- Tasks Involved: API development, backend integration, real-time monitoring.
- Costs Consideration: Developer hours, cloud hosting services for scalability, subscription costs for monitoring tools. Deployment costs vary widely but can range from $5,000 to $50,000 for enterprise-grade solutions.
4.4. Post-Launch Stage
Monitoring and Fine-Tuning: After deployment, the AI model needs regular monitoring to detect errors or performance issues. Fine-tuning involves retraining the model with new data to adapt to changing requirements or improve accuracy.
- Tasks Involved: Monitoring system setup, performance tracking, periodic model retraining.
- Costs Consideration: Monitoring tools and dashboards, developer salaries for updates. This step is critical to maintain long-term efficiency and reliability.
Cost of Scaling AI Models: Scaling ensures that the AI system can handle increasing data volumes and user demands. This requires upgrades in computational resources and infrastructure.
- Tasks Involved: Infrastructure expansion, optimization for large-scale operations, hardware upgrades.
- Costs Consideration: Additional cloud services, hardware like GPUs or TPUs, scaling software frameworks. Scaling a customer service chatbot, for example, could add 30–50% to the overall project cost.
5. Real-World Cases of AI Development Costs
The costs associated with developing and implementing artificial intelligence (AI) systems can vary significantly based on factors such as project complexity, industry requirements, and the scale of deployment. Below are real-world examples illustrating these costs:
5.1. Large-Scale AI Model Development
OpenAI’s GPT-3, training the GPT-3 model, which supports 175 billion parameters, incurred an estimated development cost of $12 million. Or, in terms of Future Projections, the CEO of Anthropic predicts that forthcoming AI systems may require approximately $1 billion for development, with subsequent generations potentially reaching $10 billion.
5.2. AI Implementation in Healthcare
General Motors’ Cruise Programs invested nearly a decade and $10 billion in developing its Cruise robotaxi program, which was eventually terminated due to challenges and costs in scaling the business amid rising competition.
5.3. AI in Manufacturing
Implementing AI for process automation in manufacturing can cost between $30,000 and $500,000.
5.4. AI Research and Development
Databricks’ Valuation, a software startup specializing in AI and data analytics, has grown to a valuation of $62 billion, reflecting significant investment in AI development and infrastructure.
It’s important to note that these figures are approximate and can vary based on specific project requirements, the expertise of the development team, data acquisition needs, and ongoing maintenance expenses. Additionally, as AI technology continues to evolve, the associated development costs are expected to change accordingly.
6. Common Cost Pitfalls and How to Avoid Them
Implementing AI solutions can be transformative, but businesses often face hidden pitfalls that inflate the AI development cost. By identifying and addressing these challenges early, you can control budgets and ensure efficient project delivery.
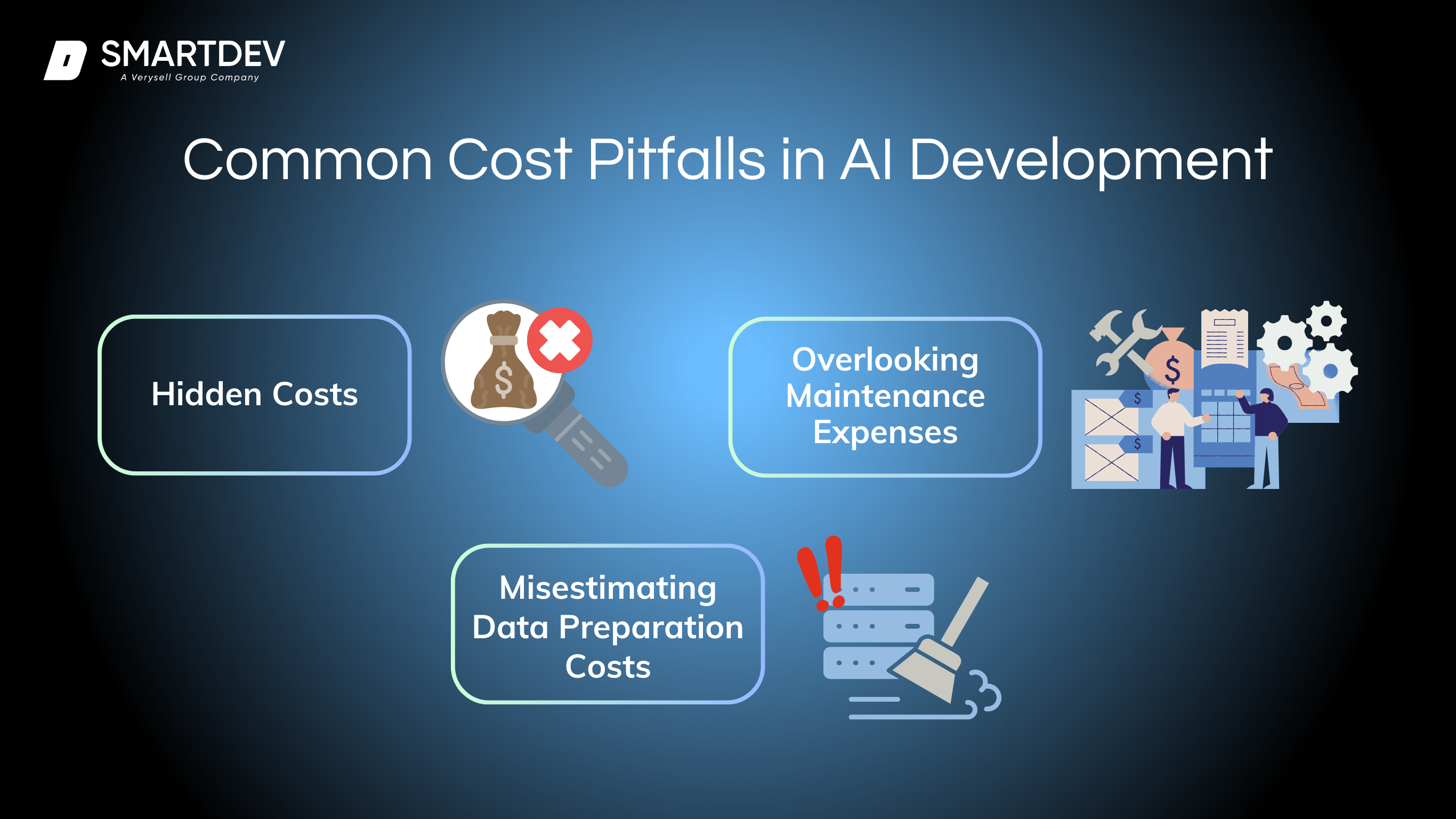
6.1. Hidden Costs in AI Development
The Pitfall: Many companies underestimate the total AI cost because they focus solely on model development. Hidden costs such as infrastructure setup, integration with existing systems, and training for employees are often overlooked.
- How to Avoid: Conduct a comprehensive cost assessment that includes hardware, software licenses, cloud services, and employee upskilling. Factoring in these hidden elements ensures a realistic budget for the entire project.
6.2. Overlooking Maintenance Expenses
The Pitfall: Businesses often fail to plan for post-deployment costs, such as model retraining, bug fixes, and system updates. These ongoing tasks can cause AI app development costs to escalate over time.
- How to Avoid: Include maintenance as a critical line item in your budget. Set clear expectations for long-term monitoring, updates, and resource allocation to keep your AI solution relevant and effective.
6.3. Misestimating Data Preparation Costs
The Pitfall: High-quality data is essential for effective AI, but collecting, cleaning, and labeling data can significantly impact the cost of AI development. Misestimating these efforts leads to project delays and budget overruns.
- How to Avoid: Assess data availability and quality early in the project. Allocate resources for data preprocessing and consider leveraging tools or external services to streamline the process.
By proactively managing these common pitfalls—hidden costs, overlooked maintenance, and underestimated data preparation—businesses can keep AI development costs under control. Careful planning, thorough assessments, and a forward-looking strategy will ensure your AI project stays on budget and delivers maximum value.
7. Your AI Budget: Balancing Cost Savings and Quality
The landscape of AI development is frequently perceived as a costly and intricate journey, particularly for businesses eager to leverage the latest technological advancements. This perception can deter many organizations from pursuing innovative AI solutions. However, there is a silver lining: various effective strategies can significantly enhance your AI development budget.
By embracing industry best practices and making judicious use of available resources, companies can not only trim expenses but also ensure the delivery of top-notch outcomes. In this discussion, we will delve into several pivotal strategies that facilitate cost-effective AI development, empowering organizations to harness the transformative power of artificial intelligence without breaking the bank.
Rationale Behind Cost-Saving Contributions
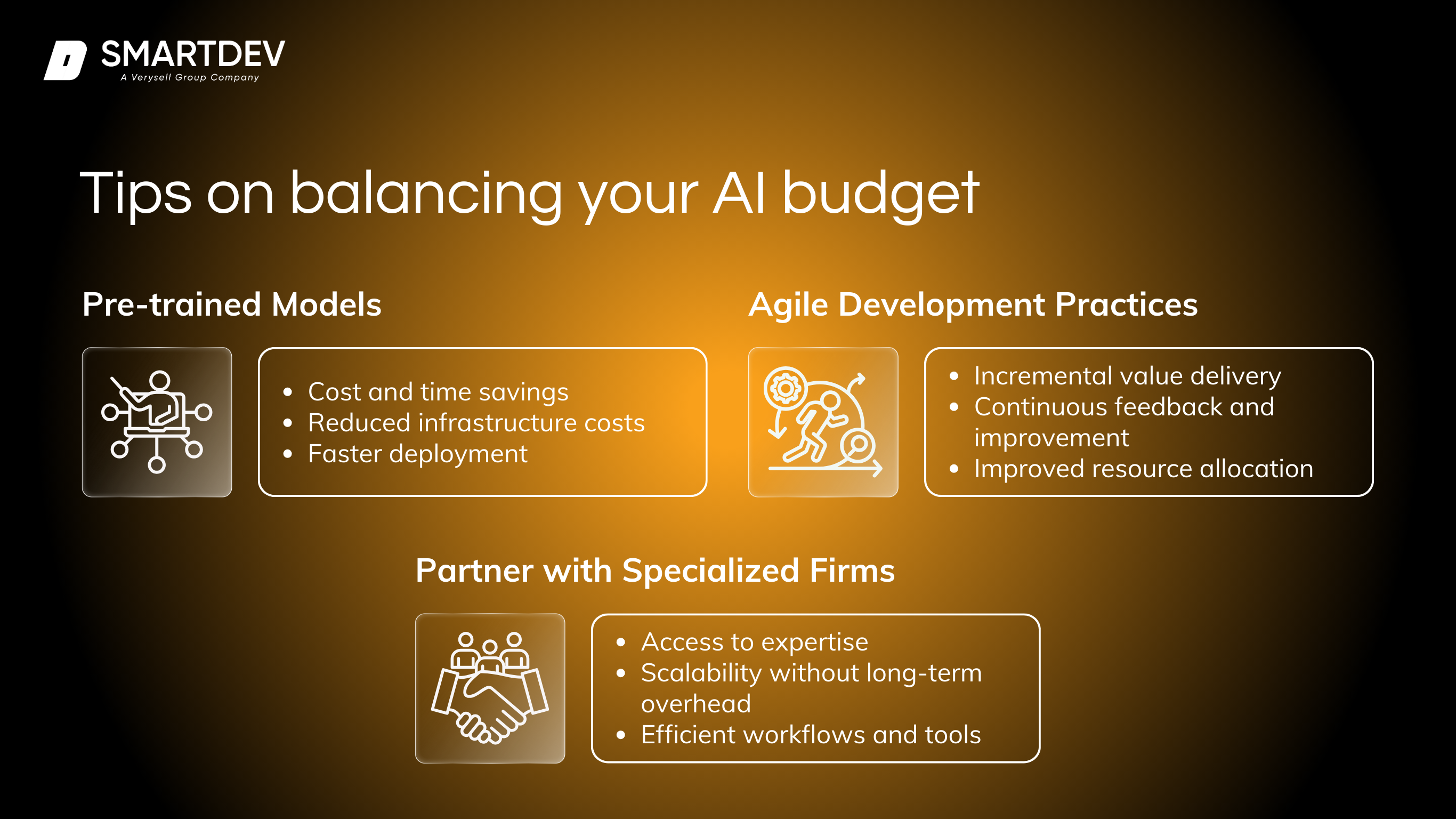
7.1. Leveraging Pre-Trained Models
Pre-trained models, widely available in leading AI libraries such as TensorFlow and PyTorch, offer substantial financial benefits by drastically cutting down the time and resources needed to create customized AI solutions from the ground up. These sophisticated models have been trained on vast and diverse datasets, which equips them with a robust understanding of various tasks, making them an ideal starting point for any AI initiative.
How It Helps Optimize Budget
- Cost and Time Savings
Developing machine learning models from scratch is a resource-intensive process that demands significant computational power, access to large datasets, and a considerable amount of time and expertise.
By utilizing pre-trained models, organizations can circumvent these hurdles. Instead of starting from zero, they can simply adapt and fine-tune these models for their specific needs and applications, resulting in a more streamlined and efficient development process.
- Reduced Infrastructure Costs
Leveraging pre-trained models significantly reduces the reliance on costly, high-performance servers or potent GPUs, which are typically necessary for the resource-intensive process of training machine learning models from scratch.
This alleviation of infrastructure demands not only leads to substantial cost savings but also enables organizations to redirect their financial and operational resources toward other vital initiatives and projects. By minimizing the need for expensive hardware, companies can invest more in innovation, talent acquisition, and improving their core business functions.
- Faster Deployment
By utilizing a robust pre-trained model as a foundational component, businesses can significantly speed up the implementation of their artificial intelligence solutions. This accelerated process allows companies to launch their products and services into the market with greater urgency, thereby strengthening their competitive advantage in an increasingly dynamic landscape.
Furthermore, this rapid deployment translates into an enhanced return on investment, enabling organizations to start experiencing the advantages of their AI initiatives much earlier in the process. As a result, they can not only maximize the benefits derived from their investments but also adapt more swiftly to evolving market demands.
7.2. Adopting Agile Development Practices
Agile development practices align perfectly with the unique requirements of AI projects, where the ability to adapt and refine is crucial. By dividing larger projects into smaller, manageable sprints, teams can focus on delivering incremental value at each stage. This approach allows for regular testing and evaluation of outcomes, fostering continuous feedback and improvement. As a result, teams can respond swiftly to new insights and changing conditions, ultimately minimizing unnecessary costs and enhancing the overall effectiveness of the project.
How It Helps Optimize Budget
- Incremental Value Delivery
Agile methodology emphasizes the importance of delivering functional outputs through short, focused cycles known as sprints. This iterative approach allows organizations to concentrate their efforts on high-priority features that promise the most significant impact. By doing so, they can effectively minimize resource expenditure on components that do not contribute substantially to project success.
Throughout these sprints, teams engage in continuous refinement of their focus, ensuring that each increment of work built during the cycle aligns with and advances the overall project goals. Through regular reassessment and adaptation, Agile fosters an environment where each contribution is not only meaningful but also enhances the trajectory of the project, leading to greater efficiency and innovation.
- Continuous Feedback and Improvement
The Agile process incorporates regular testing and solicits feedback from stakeholders throughout the development cycle. This ongoing dialogue ensures that the AI model or product remains in alignment with the business objectives, significantly reducing the likelihood of expensive rework later in the project. By fostering an environment of open communication, teams can swiftly identify and address issues, resulting in a more robust final product.
- Improved Resource Allocation
The iterative nature of Agile methodology enables teams to remain agile and responsive in the face of unforeseen challenges that can emerge throughout a project’s timeline. This inherent flexibility equips teams with the ability to strategically reallocate their resources as necessary, ensuring they can prioritize tasks effectively to meet critical deadlines and adhere to budgetary constraints.
By continually adapting to changing circumstances, organizations can not only optimize their operational efficiency but also significantly enhance the overall quality of their deliverables. This responsiveness fosters an environment where innovation can thrive, allowing teams to refine their processes and produce outcomes that truly meet the needs of their stakeholders.
7.3. Partnering with Specialized Development Firms
Collaborating with specialized AI development firms provides organizations with a unique opportunity to tap into a wealth of expertise, advanced tools, and streamlined workflows that would otherwise necessitate significant financial and resource investments if done in-house.
These firms possess a deep understanding of the intricacies involved in delivering complex AI solutions and have honed their processes to achieve remarkable efficiency. This not only helps to lower overall development costs but also ensures that the quality of the final product meets or exceeds industry standards. Through such partnerships, organizations can leverage cutting-edge technology and best practices without the burden of extensive internal development.
How It Helps Optimize Budget
- Access to Expertise
Specialized firms are staffed with seasoned professionals who possess a deep understanding of AI development, machine learning, and data science. This wealth of expertise not only shortens the learning curve for your projects but also ensures that development cycles are faster and more efficient, leading to quicker implementation of innovative solutions.
- Scalability Without Long-Term Overhead
Collaborating with specialized firms grants you the flexibility to adjust your team size according to the demands of each project, rather than committing to long-term hiring of full-time AI engineers. This approach allows you to scale your resources up or down as needed, effectively minimizing operational costs while still meeting project deadlines and objectives.
- Efficient Workflows and Tools
Development firms come equipped with established workflows, as well as a suite of pre-built components and tools that are designed to streamline the development process. By leveraging these resources, you can significantly accelerate project timelines and reduce costs, all while maintaining high standards of quality in the final product.
7.4. Maximizing ROI from Your AI Investment
Achieving a high return on investment (ROI) from AI requires more than simply reducing costs; it demands a strategic alignment of AI initiatives with core business objectives. A successful AI strategy begins with a clear understanding of how each project contributes to overall business goals. By tying AI initiatives to specific key performance indicators (KPIs), such as improved customer experience, streamlined operations, or enhanced decision-making, businesses can ensure that resources are allocated to projects that deliver measurable impact. Collaboration across departments is crucial to identify areas where AI can address pain points or unlock new opportunities.
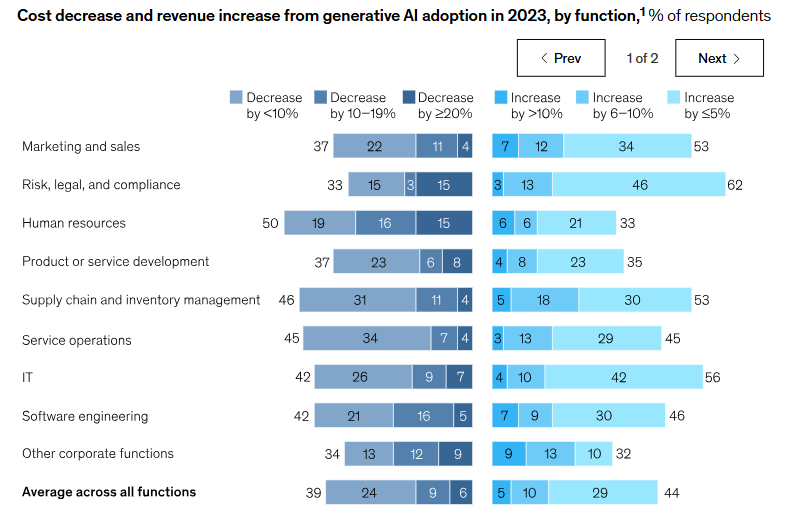
Source: McKinsey & Company
Low-hanging fruit includes any areas that AI can make a big difference with little upfront investment. Chatbots for customer service and call centers are a good example. Other quick wins may involve:
- Process automation
- Content generation
- Predictive analytics
- Data analysis
- Supply chain
- Employee experiences
- Cybersecurity
Achieving a high return on investment (ROI) from AI can be challenging, especially in the early phases. Many organizations expect to see ROI within one to three years; however, studies indicate that about 90% of AI projects fail to meet their ROI targets. To overcome these challenges, businesses should adopt a comprehensive strategy that includes integrating data analytics, AI, automation, and cloud technologies, as well as implementing end-to-end governance and fostering a culture aligned with ethical considerations
8. Industry Insights on AI Development Costs
8.1. Current Trend in AI. Pricing Models
In today’s rapidly evolving technology environment, the cost of developing and deploying AI models varies widely. Factors like model complexity, data requirements, and computerresources play a significant role in shaping the overall expense. Here are a few key trends shaping AI development pricing:
Pre-trained Models vs. Custom Models
- Pre-trained models, such as GPT or BERT, are increasingly being leveraged as a cost-effective option. These models can be fine-tuned for specific use cases, significantly reducing development time and expenses.
- On the other hand, custom AI models, tailored from scratch, require extensive resources for data collection, training, and validation, which can drive up costs.
Cloud-Based AI Services
- Major players like AWS, Azure, and Google Cloud offer AI services on a pay-as-you-go basis. This allows businesses to scale their projects without the need for hefty upfront investments in infrastructure.
Open Source and Collaborative AI
- The open-source movement has introduced tools and frameworks like TensorFlow and PyTorch, making AI development more accessible. However, expertise is still required to maximize their potential, which can impact the overall cost.
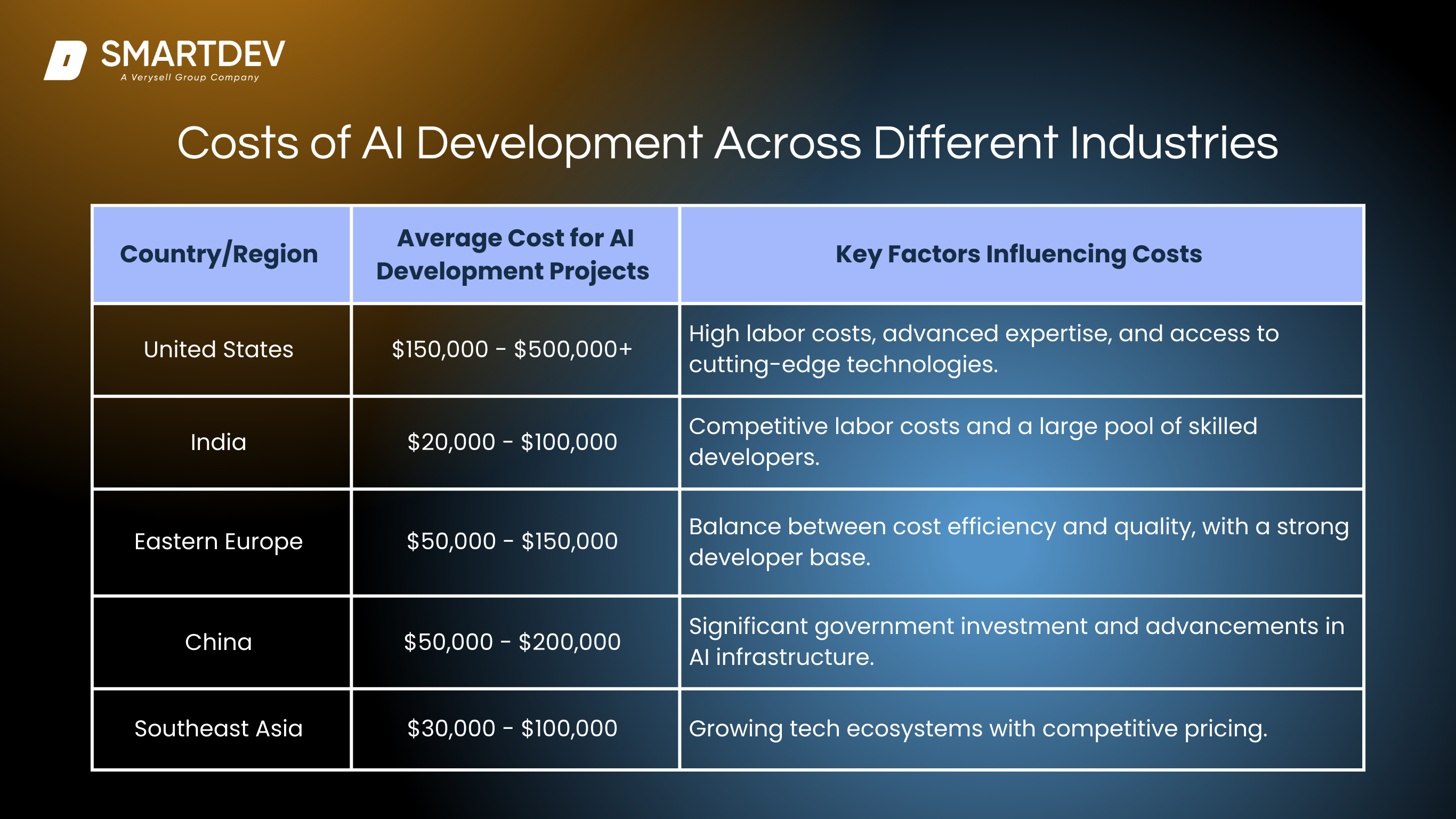
8.2. Impact of Technological Advancements on Costs
Advancements in technology have been a double-edged sword for AI development costs. On one hand, breakthroughs in hardware, such as GPUs and TPUs, have significantly reduced the time and expense involved in training models. Similarly, automation tools and synthetic data generation have streamlined workflows, making development faster and more cost-efficient.
However, the flip side is that these advancements also lead to the creation of more sophisticated models. For example, large language models (LLMs) like GPT-4 come with immense computational and data requirements, driving up costs. Additionally, maintaining and fine-tuning such models can be resource-intensive over time.
8.3. Outlook: How AI Costs Are Expected to Change
Looking ahead, AI development costs are expected to follow a mixed trajectory:
- Decreasing Costs for Entry-Level AI Projects: As tools and platforms become more democratized, entry-level AI projects will likely see lower barriers to entry. Pre-trained models and no-code AI platforms will enable smaller businesses to adopt AI cost-effectively, with initial budgets ranging from $5,000 to $50,000.
- High Costs for Cutting-Edge Innovation: Companies aiming to push the boundaries of what AI can achieve will continue to face high costs. For example, developing multimodal systems or generative AI applications may require budgets exceeding $1 million due to intensive R&D, computational power, and data acquisition needs.
- Greater Predictability Through Subscription Models: AI services are shifting toward subscription-based models, providing businesses with predictable monthly costs for accessing robust AI tools and infrastructure. Subscription fees can range from $1,000 to $10,000 per month, depending on the scope and scale of services required.
9. FAQs on AI Development Costs
Q1: What are the main factors that make AI development so expensive?
AI development involves highly complex algorithms, huge amounts of high-quality data, and powerful computational resources. Think about training a marathon runner—it takes time, effort, and resources. Similarly, AI needs robust training with top-notch tools, and that’s why it’s costly. The expertise of developers and data scientists further adds to the price, especially in high-wage regions. On top of this, hardware like GPUs and cloud services rack up the costs.
Q2: Are there any hidden costs in AI development that businesses should be aware of?
YES. Beyond the obvious costs of development, there are “surprise” expenses. For example, you might spend more than planned on cleaning and organizing your data or buying licenses for specialized tools. Integration with existing systems can also sneak into the budget, and let’s not forget the ongoing costs—monitoring, debugging, and retraining models to keep them sharp. These hidden elements can catch businesses off guard if they’re not prepared.
Q3: How much does it cost to maintain and update an AI solution after deployment?
Maintenance is like giving your car regular tune-ups. After deployment, AI solutions need attention to keep performing well. Retraining the model with new data, fixing bugs, and scaling infrastructure can cost about 15-20% of the original project cost every year. So, if your AI project was $100,000, expect to spend around $15,000 to $20,000 annually for upkeep.
Q4: What Is the Cost Difference Between AI and Traditional Software Development?
AI is a different ballgame compared to traditional software development. While standard software might cost between $50,000 and $200,000, AI can start at $100,000 and go well beyond $1 million. The reason? AI involves more advanced computational needs, ongoing training, and specialized skills. Traditional software is more like building a house, while AI is like creating a self-sustaining smart city—more resources and effort are required.
Q5: Can small businesses afford to implement AI solutions?
Of course! AI is becoming more accessible for small businesses thanks to pre-trained models, no-code platforms, and cloud-based services. These options significantly reduce upfront costs. Small-scale projects can start as low as $5,000 to $20,000, making AI implementation achievable for businesses with limited budgets. With the right planning and tools, even smaller players can harness the power of AI to stay competitive.
Conclusion
For organizations looking to embark on their AI journey, understanding these cost dynamics is crucial. Investing in AI doesn’t always mean breaking the bank—with careful planning and leveraging the right tools, businesses can achieve impactful results without overspending.
At SmartDev, we’re here to guide you through this journey, offering insights and solutions tailored to your unique needs. By staying informed and choosing the right development strategies, you can harness the power of AI while keeping your costs under control.
References
- PwC’s Global Artificial Intelligence Study: Exploiting the AI Revolution | PricewaterhouseCoopers
- How Tesla Uses and Improves Its AI for Autonomous Driving | AI Wire
- The Hidden Costs Of Implementing AI In Enterprise | Forbes
- The state of AI in early 2024: Gen AI adoption spikes and starts to generate value | McKinsey & Company
- Understanding Google’s Algorithm Updates | Kevin McLauchlin | LinkedIn
- Cost Comparison of In-House vs. Outsourced AI Development Team | Superstaff