Why AI is Revolutionizing Financial Fraud Detection
In today’s fast-paced, digitally driven world, financial fraud has become a growing threat, affecting individuals and businesses alike. From phishing attacks to sophisticated identity theft schemes, fraudsters are constantly evolving their tactics, leaving many traditional security measures struggling to keep up. It’s a stark reminder of just how vulnerable we all can be in this interconnected age.
But here’s the good news: technology, in this case, Artificial Intelligence (AI), is revolutionizing the way we fight back. AI isn’t just a buzzword; it’s a game-changer in fraud prevention. With its ability to analyze massive amounts of data, detect patterns, and predict risks in real time, AI offers a level of accuracy and speed that was unimaginable just a few years ago. It’s not about replacing human expertise but empowering it – giving financial institutions, businesses, and even individuals a fighting chance to stay one step ahead of fraudsters.
At SmartDev, we’re passionate about exploring how cutting-edge technology can solve real-world problems. As an ITO company based in Vietnam, we’ve witnessed firsthand how AI-driven solutions are reshaping the financial security landscape. In this blog, we’re excited to share insights into how AI is transforming fraud detection, why it matters, and what it means for the future of financial security. Let’s dive in together!
- Financial fraud: A growing threat that exploits digital advancements, demanding innovative solutions beyond traditional methods.
- AI’s role: AI transforms fraud detection with real-time monitoring, adaptability, and precision, addressing the growing complexity of financial fraud.
- Advantages: AI improves fraud prevention by reducing false positives, enhancing accuracy, and building customer trust while ensuring scalability.
- Implementation Essentials: Key steps include integrating data, training AI models, and continuously updating systems to stay ahead of emerging threats.
1. The Scope of Financial Fraud
Financial fraud has been a growing concern worldwide, impacting not only individuals and businesses but also the whole economy. To understand its impact, it’s essential to recognize the common types of financial fraud and understand the current situations of financial frauds to consider the legal consequences for perpetrators.
The 2024 AFP Payments Fraud and Control Survey revealed that 80% of organizations experienced attempted or actual payment fraud in 2023, a 15-percentage point increase from the previous year. They stated:
“This number reflects a 15-percentage point increase in payments fraud over 2022 and the highest rate reported since 2018. With heightened awareness and increased preventative controls and processes in place, the increase is not a result of a passive mindset among organizations but rather relentless attempts by fraudsters.”
Additionally, the Association of Certified Fraud Examiners (ACFE) – the world’s largest anti-fraud organization – reported that organizations lost a total of $3.1 billion to fraud, with a median loss of $117,000 per case. The reports estimated that businesses lose about 5% of their revenue to fraud annually as of 2024. Importantly, the numbers also revealed an interesting fact that a typical fraud case lasted about 12 months before it was detected, which is insane! For that amount of time, it is impossible for the victims to get their assets back.
a. Common Types of Financial Fraud: A Closer Look
Identity Theft: it occurs when fraudsters steal personal information, such as Social Security numbers, credit card details, or login credentials, to impersonate someone else and commit fraud.
- How it Happens: Data breaches, phishing scams, or theft of physical documents.
- Impacts: Victims may face unauthorized transactions, damaged credit scores, and years of recovery efforts.
- Example: During the COVID-19 pandemic, there was a significant surge in fraudulent unemployment claims filed using stolen personal information. Many individuals discovered they were victims upon receiving unexpected mail from government agencies regarding unemployment benefits they had not applied for.
Phishing and Social Engineering: These scams trick victims into revealing sensitive information, often through fake emails, messages, or phone calls.
- How it Happens: Fraudsters pose as legitimate organizations (banks, government agencies, or well-known companies).
- Impacts: Can lead to compromised accounts, unauthorized transactions, or identity theft.
- Example: The “Nigerian Prince” scam, also known as the “419 scam”, is a classic example where fraudsters pose as foreign royalty in distress, soliciting financial assistance with the promise of substantial repayment. This scam has evolved over time, adapting to new communication technologies to reach potential victims.
Payment Fraud: involves the unauthorized use of payment systems, such as credit cards or digital wallets, to steal funds.
- Types:
-
- Credit Card Fraud: Using stolen card information for purchases.
-
- Wire Fraud: Deceiving victims into transferring funds.
-
- Account Takeover: Gaining control of a victim’s account to initiate fraudulent payments.
- Example: In 2023, a significant increase in check fraud was observed, with criminals stealing checks from mailboxes and altering them to withdraw large sums from victims’ accounts. This resurgence in check fraud highlights vulnerabilities in traditional payment methods.
Investment Scams: fraudsters lure victims with promises of high returns in non-existent or dubious investment schemes.
- How it Happens: Pyramid schemes, fake cryptocurrency platforms, or “get-rich-quick” programs.
- Impacts: Victims often lose significant amounts of money, with little chance of recovery.
- Example: The “Pig Butchering” scam involves fraudsters cultivating online relationships to convince victims to invest in fraudulent cryptocurrency platforms, leading to substantial financial losses. This scam has become increasingly prevalent, with billions of dollars stolen globally.
Insurance and Loan Fraud: This type of fraud involves the misuse of insurance policies or loan applications for financial gain.
- How it Happens:
-
- Insurance Fraud: Filing false claims or exaggerating damages.
-
- Loan Fraud: Using fake documents to secure loans.
- Impacts: Drives up premiums for honest policyholders and leads to stricter lending processes.
- Example: A fraudster placed fake job advertisements to steal applicants’ personal information, which was then used to create fraudulent bank accounts and secure loans, resulting in significant financial gains for the perpetrator.
Money Laundering: involves disguising the origins of illegally obtained money, making it appear legitimate.
- How it Happens: Layering funds through multiple accounts, shell companies, or real estate purchases.
- Impacts: Fuels organized crime and undermine economic stability.
- Example: The “Black Axe” syndicate has been implicated in complex money laundering schemes, using unsuspecting individuals, including the elderly, as drug mules to transport illicit funds internationally.
Cyber Fraud: includes crimes conducted through digital platforms, targeting sensitive information or financial systems.
- How it Happens: Malware, ransomware attacks, and hacking.
- Impacts: Disrupts businesses, compromises personal data, and leads to financial losses.
- Example: In 2017, the Equifax data breach exposed the personal information of over 147 million people, leading to widespread fraud attempts.
b. The Bernard Madoff Case: A Billion-Dollar Ponzi Scheme
As some of you may know, one of the most famous cases of financial fraud in recent history is the Bernard Madoff Ponzi Scheme. This case exemplifies how fraudulent activities can grow to a massive scale, exploiting trust and regulatory gaps.
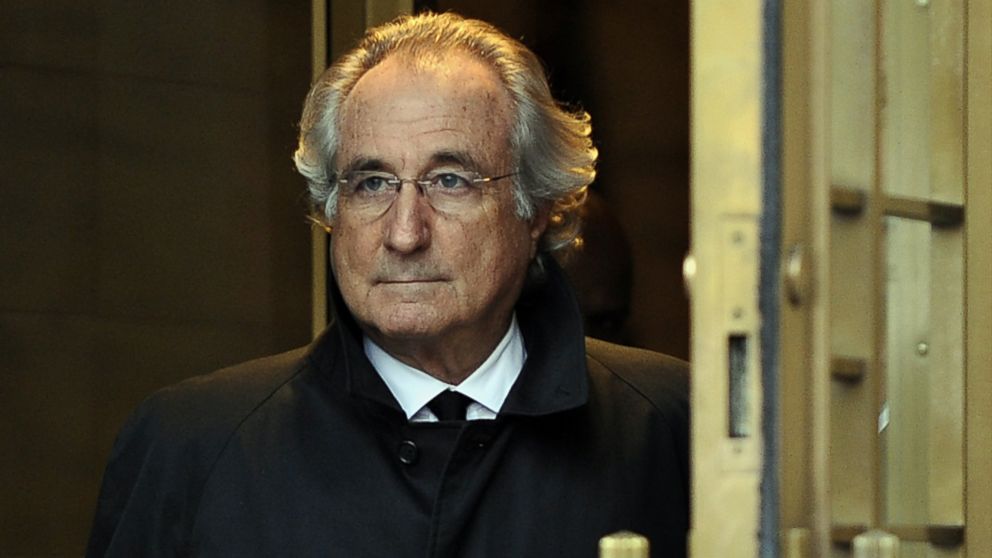
Source: ABC News
Bernard “Bernie” Madoff, a former NASDAQ chairman and respected financier, orchestrated the largest Ponzi scheme in history, defrauding investors of an estimated $65 billion. His scheme spanned decades and preyed on individuals, charities, and institutions worldwide.
How It Worked
Madoff promised consistent, high returns on investments through his wealth management firm. Instead of generating legitimate profits, he used funds from new investors to pay returns to earlier ones. This cycle created an illusion of success, attracting more investors and allowing the scheme to expand.
Key Moments
- The Unraveling: In 2008, during the global financial crisis, Madoff’s clients began requesting withdrawals, which he couldn’t fulfill due to insufficient funds. This led to his arrest in December 2008.
- The Fallout: Thousands of investors, including high-profile individuals and institutions, suffered significant losses. Charities and retirement funds were wiped out, highlighting the devastating personal and organizational impacts.
Consequences
- Legal Actions: In 2009, Madoff was sentenced to 150 years in prison, the maximum penalty for his crimes.
- Restitution Efforts: Court-appointed trustees worked to recover funds for victims, clawing back billions from investors who had benefited from the scheme.
Lessons Learned
This case underscores the importance of due diligence, transparency, and robust regulatory oversight in financial systems. It also illustrates how trust can be exploited, making it a stark warning for both individuals and institutions.
The Bernie Madoff case remains a pivotal example of financial fraud’s scale and impact, serving as a crucial reminder of the need for vigilance and advanced detection mechanisms – such as AI-driven solutions – to prevent such schemes in the future.
c. Consequences of Financial Fraud
The consequences of financial fraud are not only severe – but they are also life-altering. Perpetrators face staggering fines that can bankrupt them and leave their families in financial ruin. Prison sentences for major fraud cases can stretch into decades, effectively destroying any chance of rebuilding their lives outside of a cell. In addition to these penalties, courts often mandate restitution orders, forcing fraudsters to repay victims—a near-impossible task given the sums involved.
And it doesn’t end there: the permanent stain of a criminal record closes doors to employment, tarnishes reputations, and isolates offenders from society. For those who think they can get away with it, the reality is far grimmer than they imagine, as legal systems worldwide are tightening their grip, leaving no room for leniency. Financial fraud isn’t just a crime; it’s a one-way ticket to a ruined life.
2. Investigating Financial Fraud with AI: Processes and Technology
Traditional methods of investigating financial fraud have served us well for decades, but they are no longer sufficient in today’s fast-paced, data-driven world. Consequently, enter Artificial Intelligence (AI), a revolutionary force that’s transforming how we detect and prevent financial fraud.
a. Traditional Methods of Financial Fraud Investigation
Before the rise of AI, financial fraud investigations relied on traditional techniques that were the backbone of financial security. However, as fraudsters became more creative and the volume of transactions surged, these methods started to show significant limitations.
Manual Audits
Manual audits were the cornerstone of fraud detection. Teams of investigators would pore over financial records line by line, searching for irregularities or inconsistencies. While thorough, this approach was painstakingly slow and highly dependent on the experience and intuition of the auditors. It often failed to catch subtle anomalies, especially in datasets that were too large for humans to analyze comprehensively.
Transaction Tracking
Transaction tracking was another key strategy. Financial institutions monitored account activities for unusual behaviors, such as sudden large withdrawals or multiple small transactions designed to avoid detection thresholds. However, these systems were only as effective as the rules they followed. Static thresholds and predefined triggers meant fraudsters could easily adapt their tactics to evade detection, exploiting the rigidity of these systems.
Red Flag Identification
Red flag identification also played a critical role. Certain activities, such as transactions from high-risk locations or excessive account logins, were flagged for further scrutiny. Yet, these triggers were often reactive rather than proactive. They relied on historical fraud patterns, leaving gaps in detecting emerging schemes or sophisticated tactics that fell outside predefined rules.
Reactive Investigations
A major drawback of these traditional methods was their reactive nature. Fraud investigations often began only after an incident was reported or significant financial losses were already incurred. By then, the damage was done – money was lost, reputations were tarnished, and recovery became a long and arduous process.
Human-Centric Processes
Finally, the human-centric nature of these processes added another layer of complexity. Skilled investigators played a vital role in piecing together evidence, but they were limited by the time and resources available to them. As the volume of financial data grew exponentially, manual efforts struggled to keep up. This gap created opportunities for fraudsters to exploit the delays and inefficiencies.
While these methods laid a strong foundation for combating fraud, their limitations became glaringly obvious in the face of modern challenges. The need for a faster, more adaptable, and data-driven solution paved the way for the adoption of AI, a technology that has redefined how financial fraud is detected and prevented.
b. How AI Enhances Fraud Detection and Investigation
Traditional fraud detection methods rely heavily on fixed rules and manual processes. For example, transactions over a specific dollar amount might trigger a red flag, or auditors would comb through records to uncover discrepancies. The problem? These methods often catch fraud only after it has occurred. Worse, they are slow, error-prone, and rigid—fraudsters can easily adapt their tactics to bypass these systems.
AI takes a completely different approach. Instead of relying on static rules, it uses machine learning algorithms that continuously analyze patterns, learn from past data, and adapt to emerging threats. It doesn’t just follow instructions; it evolves, becoming smarter over time.
Keyways AI Transforms Fraud Detection
- Real-Time Insights, Real-Time Action
AI can analyze enormous volumes of data in real time, something no team of humans or traditional system could achieve. It evaluates thousands of transactions per second, detecting irregularities as they happen. This speed enables financial institutions to block suspicious activities before any damage is done—whether it’s a fraudulent transfer, unauthorized login, or an unusual purchase pattern.
- Finding Hidden Patterns
Fraud isn’t always obvious. Sometimes, the clues are scattered across multiple data points—a slightly unusual login time, a transaction just under a threshold, or a pattern of purchases in unfamiliar locations. AI excels at piecing these subtle clues together, revealing suspicious behaviors that would be invisible to the human eye or traditional systems.
- Learning and Evolving
Fraud tactics change constantly, but AI adapts. Machine learning models analyze new data and evolve without waiting for manual updates. For instance, if fraudsters start using new techniques, AI can detect and adapt to these changes much faster than traditional systems, which often require weeks or months to update.
- Precision Over Paranoia
One major problem with traditional fraud systems is the high rate of false positives—legitimate transactions flagged as suspicious. This not only wastes time but also frustrates customers. AI reduces false alarms by being more precise. It evaluates each case in context, using multiple data points to determine whether an activity is genuinely suspicious.
- Empowering Investigators
AI isn’t here to replace human expertise—it’s here to enhance it. By handling the heavy lifting, such as analyzing data and flagging high-risk activities, AI frees investigators to focus on strategic decision-making. Fraud analysts can use AI-generated insights to dive deeper into the most critical cases, speeding up investigations and improving outcomes.
AI Fraud Detection Models
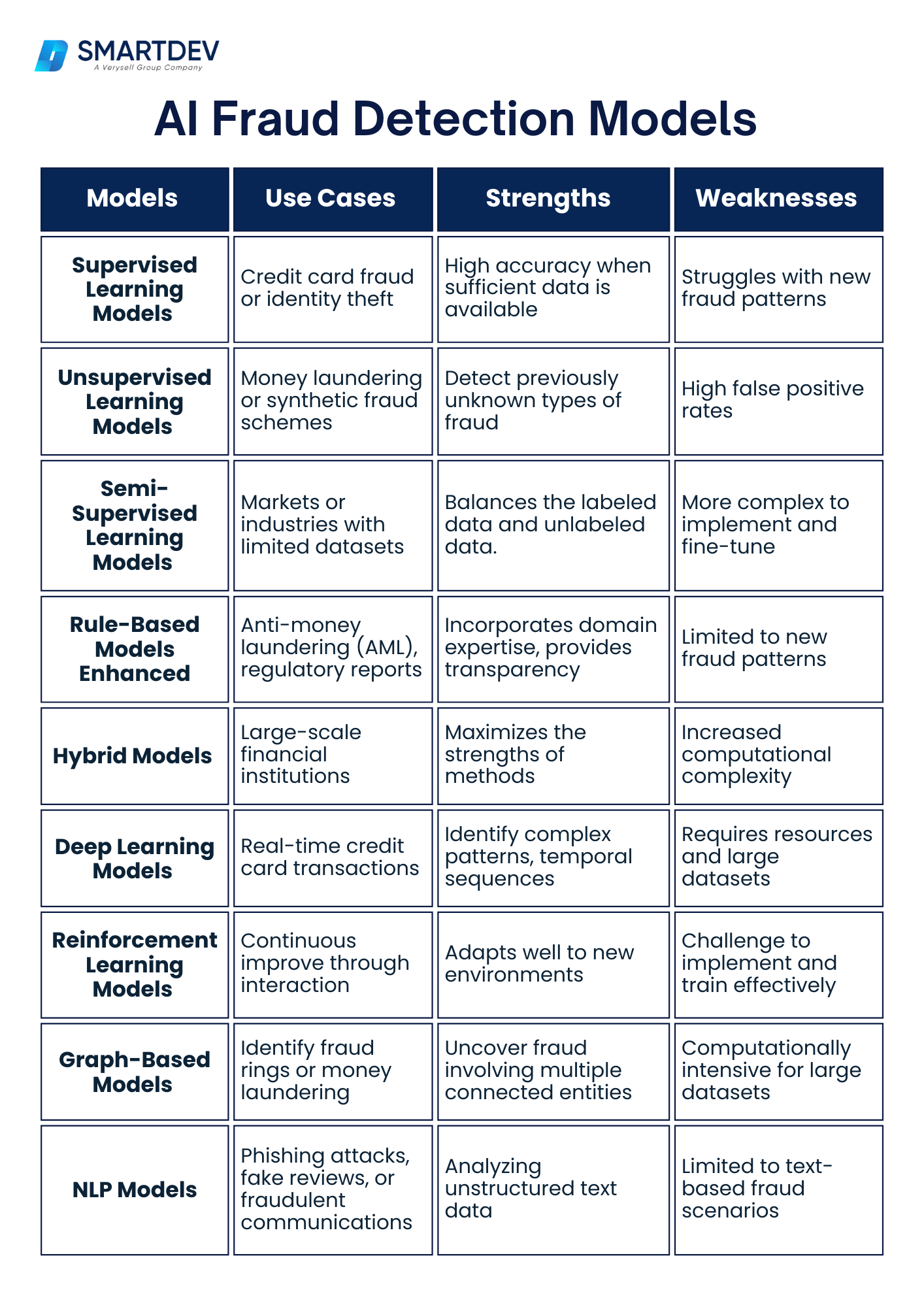
The choice of AI model depends on the type of fraud, available data, and specific needs of the financial institution. Many organizations use hybrid approaches, combining several models to create a robust fraud detection system. The power of AI lies in its adaptability, precision, and ability to scale, making it an essential tool in the fight against financial fraud.
c. How AI Improves the Financial Sector as a Whole
Beyond just catching fraud, AI improves the entire financial ecosystem:
- Stronger Security: By stopping fraud faster and more effectively, AI builds trust between institutions and their customers.
- Cost Efficiency: Investigating fraud is expensive, but AI reduces costs by automating time-consuming tasks and improving accuracy.
- Seamless Customer Experience: AI minimizes disruptions by reducing false positives, ensuring legitimate transactions go through smoothly.
- Scalability: As financial institutions grow and data volumes increase, AI scales effortlessly, ensuring fraud detection systems remain robust.
d. Step-by-Step Guide to Investigating Financial Fraud Using AI
Investigating financial fraud using AI might seem complex at first, but it’s a structured and straightforward process once you break it down. Here’s a clear guide to help you understand how an AI-driven investigation unfolds:
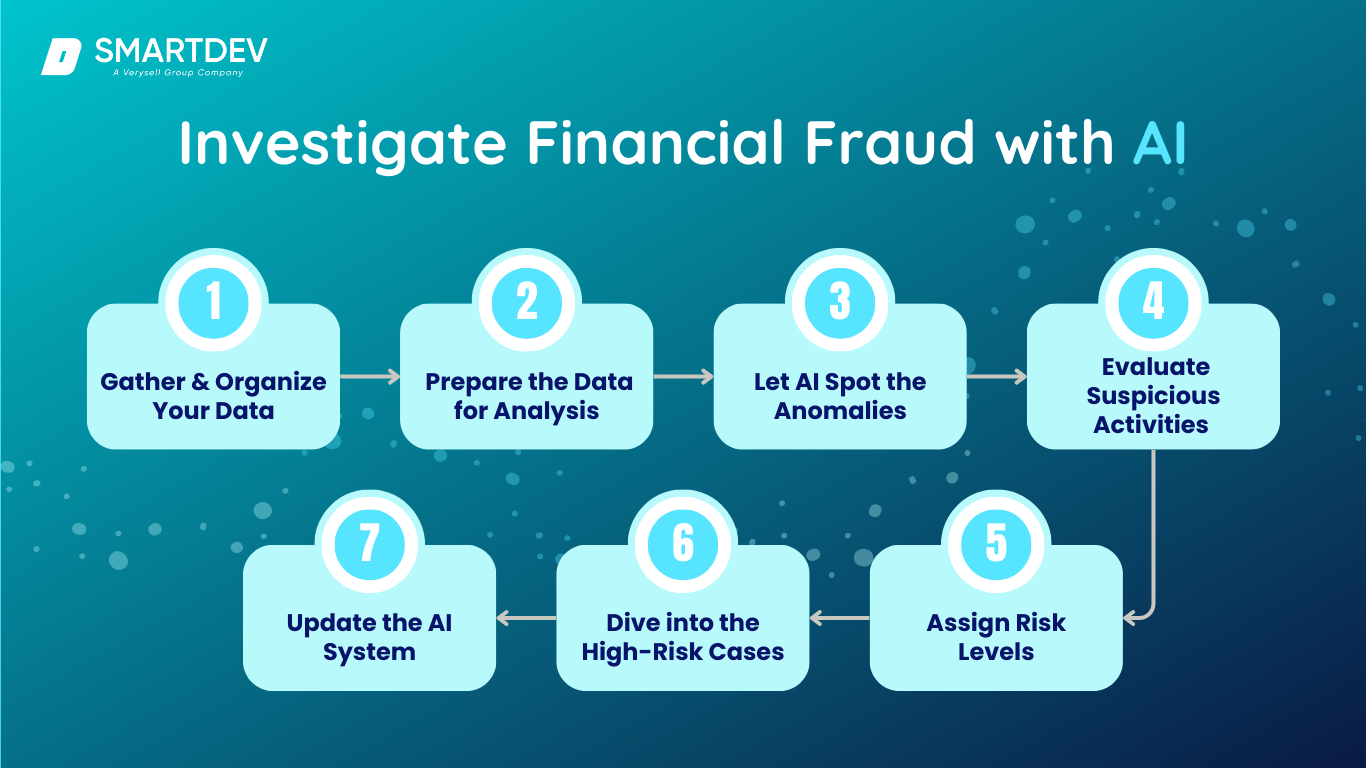
Step 1: Gather and Organize Your Data
Start by collecting all the relevant data. This includes transaction records, account activities, login histories, and even external data sources like blacklists of fraudulent accounts. All this information is fed into the AI system for analysis. Think of it as giving the AI all the puzzle pieces it needs to start identifying where things might not fit.
Step 2: Prepare the Data for Analysis
Before AI can work its magic, the data needs to be cleaned. This step ensures there are no duplicate entries, missing values, or inconsistencies. The system automates this process, ensuring the data is reliable and ready for analysis. This step is like tidying up a workspace before starting an important project—it sets the stage for success.
Step 3: Let AI Spot the Anomalies
The AI system will analyze the data to detect anything that stands out as unusual or suspicious. This could be a sudden spike in transactions, logins from unexpected locations, or behavior that doesn’t match a customer’s usual patterns. At this stage, the AI acts like a detective scanning for clues.
Step 4: Evaluate the Suspicious Activities
Once the anomalies are flagged, the AI system evaluates them in greater detail. It cross-references them with historical data and looks for patterns that might indicate fraudulent behavior. For example, if a flagged transaction is part of a series of small withdrawals across multiple accounts, it might indicate a coordinated scheme.
Step 5: Assign Risk Levels
AI assigns a risk score to each flagged activity. This score helps prioritize which cases are most likely to be fraudulent and need immediate attention. High-risk activities are marked for deeper investigation. This is like highlighting the most urgent tasks on your to-do list
Step 6: Dive Deeper into the High-Risk Cases
Now it’s time for investigators to step in. Using the insights provided by the AI, they focus on the most critical cases, reviewing details to determine if fraud has occurred. If confirmed, necessary actions—such as freezing accounts or reporting to authorities—can be taken. This is where human expertise combines with AI intelligence to make informed decisions.
Step 7: Update the AI System
The final step is feedback. The outcomes of investigations—whether a flagged activity was fraudulent or not—are fed back into the AI system. This helps it learn and refine its detection abilities over time. Think of it as teaching the system so it gets even better at spotting fraud in the future.
The process might sound technical, but it’s designed to be intuitive. With AI handling the heavy data analysis and risk evaluation, your role focuses on decision-making and action. Whether it’s your first time investigating fraud or you’re a seasoned analyst, AI simplifies the process, making it faster and more efficient.
3. AI-Powered Tools for Fraud Detection
a. Overview of popular AI tools in fraud detection
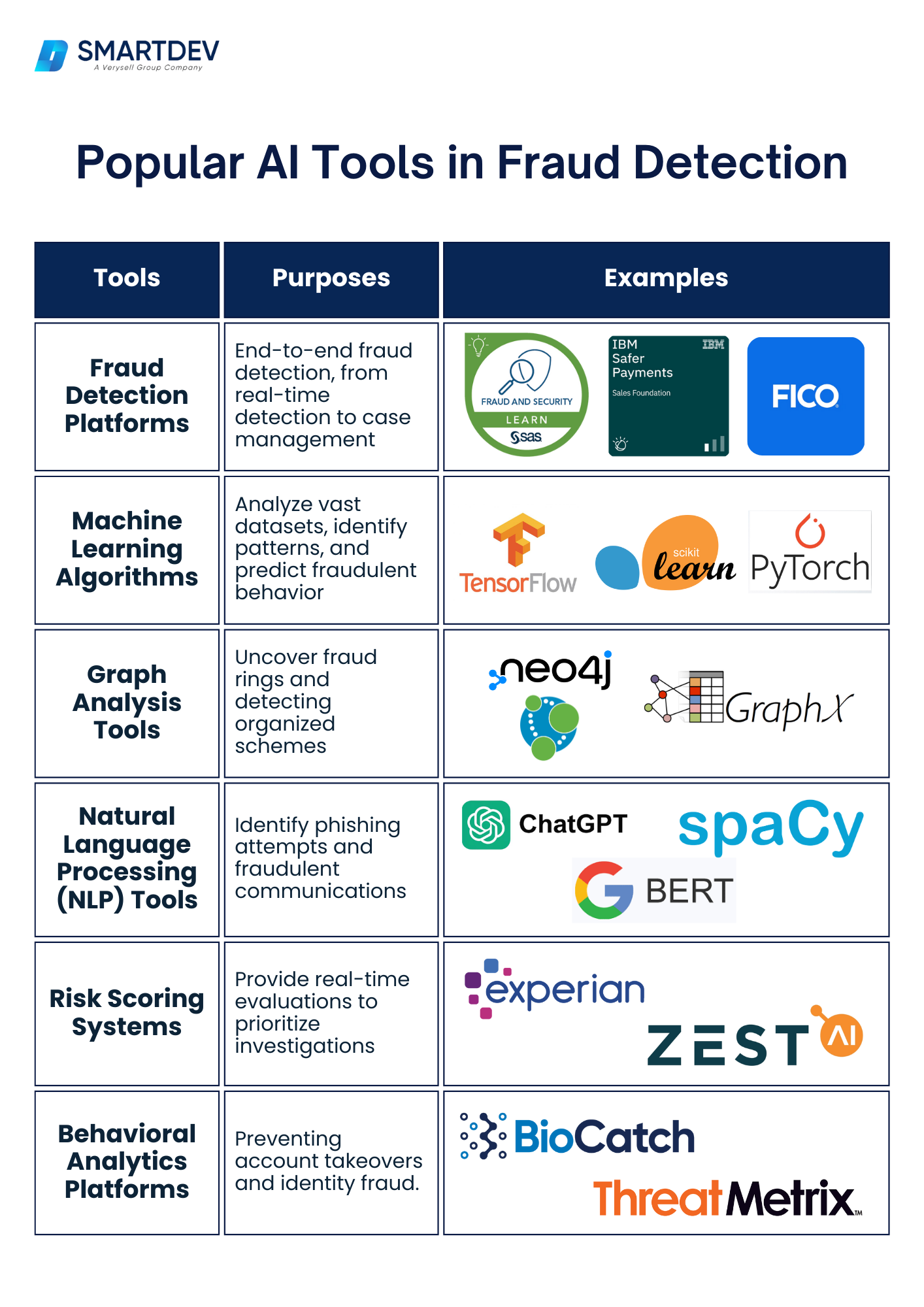
b. Use Cases of AI Tools in Financial Fraud Detection
Fraud is a persistent challenge in the financial sector, and while AI is making strides in combating it, there are three critical areas that demand greater attention to truly turn the tide: payment processing, credit scoring, and transaction monitoring. These domains are not only frequent targets for fraudsters but also foundational to financial security and customer trust.
Payment processing
AI has revolutionized payment processing by enabling real-time fraud detection and prevention. For instance, machine learning models in platforms like FICO Falcon Platform and SAS Fraud Management monitor transactions as they happen, identifying anomalies such as purchases from unexpected locations, unusual spending patterns, or transactions at odd hours. Behavioral analytics tools like BioCatch further enhance this process by analyzing user behavior, such as typing speed or interaction style, to detect fraudulent activities like account takeovers or card cloning.
Credit scoring
Traditional credit scoring methods often exclude individuals without extensive credit histories, leaving gaps in assessing true creditworthiness. AI-powered tools like Zest AI and Experian Decision Analytics address this by analyzing alternative data, such as utility bill payments, rental history, and even behavioral data from social media or online interactions. These insights help financial institutions make fairer and more inclusive lending decisions.
In addition to assessing credit risk, AI tools combat fraud in the credit application process. By leveraging natural language processing tools like spaCy or graph analysis platforms such as Neo4j, AI can identify inconsistencies in application details, flag fake identities or forged documentation. This dynamic approach not only minimizes default rates but also protects lenders from sophisticated application fraud schemes.
Transaction monitoring
AI-powered transaction monitoring systems are indispensable for detecting suspicious activities across accounts and ensuring compliance with regulatory standards. Tools like IBM Safer Payments and SAS Fraud Management specialize in identifying high-risk patterns, such as transactions involving multiple accounts or countries known for money laundering. These tools are complemented by graph analysis platforms like Neo4j, which trace connections between accounts to uncover fraud networks.
For anti-money laundering (AML) purposes, AI models continuously analyze transaction data to detect behaviors like frequent small deposits followed by large withdrawals—classic indicators of layering in money laundering. Behavioral analytics platforms like ThreatMetrix also play a crucial role by monitoring changes in login habits, device usage, or IP addresses, flagging unauthorized account access, or suspicious transfers.
4. Advantages of AI in Financial Fraud Detection
Artificial Intelligence (AI) has become a game-changer in combating these threats, offering numerous advantages that traditional methods simply cannot match. Here are some of the most impactful benefits of AI in financial fraud detection:
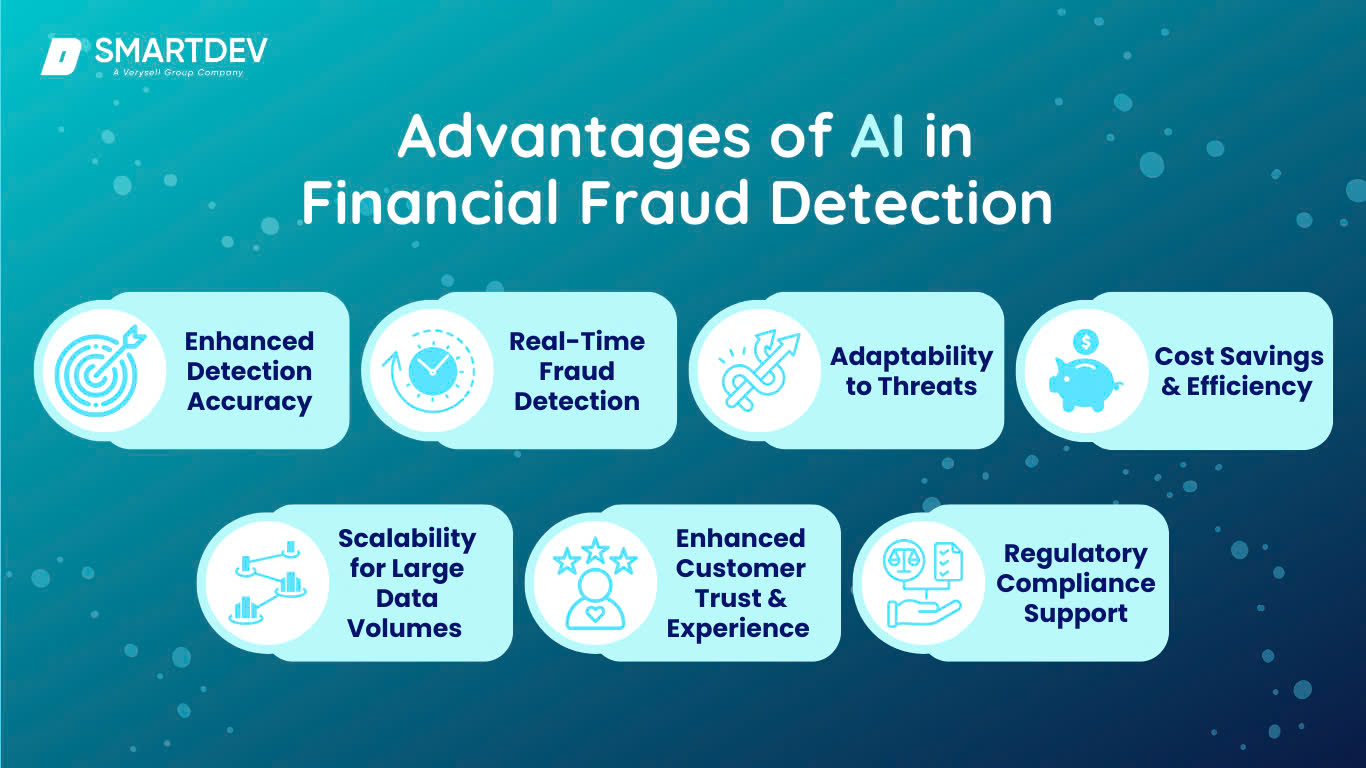
Enhanced Detection Accuracy
AI-driven fraud detection systems can analyze vast amounts of real-time data, enabling them to detect fraudulent activities more accurately and efficiently. By leveraging advanced algorithms and machine learning, these systems can identify patterns and anomalies that traditional methods might miss. This leads to fewer false positives and more accurate fraud detection, resulting in cost savings for financial institutions.
Real-Time Fraud Detection
AI-driven fraud detection systems operate in real-time, allowing financial institutions to detect and respond to fraudulent activities as they happen. This real-time detection enables swift action, minimizing the impact of fraud and reducing financial losses. By detecting fraud in its early stages, financial institutions can prevent further damage and protect their customers’ assets.
Adaptability to Emerging Threats
Fraudsters are constantly evolving their tactics to bypass traditional fraud detection methods. AI-driven fraud detection systems are designed to adapt and learn from new data, enabling them to keep up with emerging fraud trends. By continuously updating their algorithms and analyzing new patterns, these systems can stay one step ahead of fraudsters, providing enhanced security for financial institutions and their customers.
Cost Savings and Improved Efficiency
By automating the fraud detection process, AI-driven systems can significantly reduce manual effort and free up resources for other critical tasks. This results in cost savings and improved efficiency for financial institutions. Additionally, the reduction in false positives allows fraud investigators to focus on genuine cases, streamlining the investigation process and saving valuable time.
Scalability for Large Data Volumes
As the volume of financial transactions grows, AI systems scale effortlessly to handle this increased data load. Tools like TensorFlow and Scikit-learn enable financial institutions to process millions of transactions daily without compromising on speed or accuracy. This scalability is essential for global institutions managing operations across multiple markets.
Enhanced Customer Trust and Experience
AI’s ability to reduce false alarms and swiftly resolve fraud cases improves the customer experience. Customers can trust that their transactions are secure without unnecessary disruptions or delays. Behavioral analytics tools like BioCatch even add a layer of personalized protection by monitoring each user’s unique patterns to flag unauthorized activity.
Regulatory Compliance Support
AI tools assist financial institutions in meeting stringent regulatory requirements, such as anti-money laundering (AML) and know-your-customer (KYC) standards. Platforms like IBM Safer Payments integrate compliance frameworks, automatically flagging suspicious activities for further review and reducing the risk of penalties or reputational damage.
5. Challenges and Limitations
While AI has revolutionized fraud detection and prevention, it is not without its challenges and limitations. Let’s delve into some of the key challenges and limitations associated with AI-driven fraud detection:
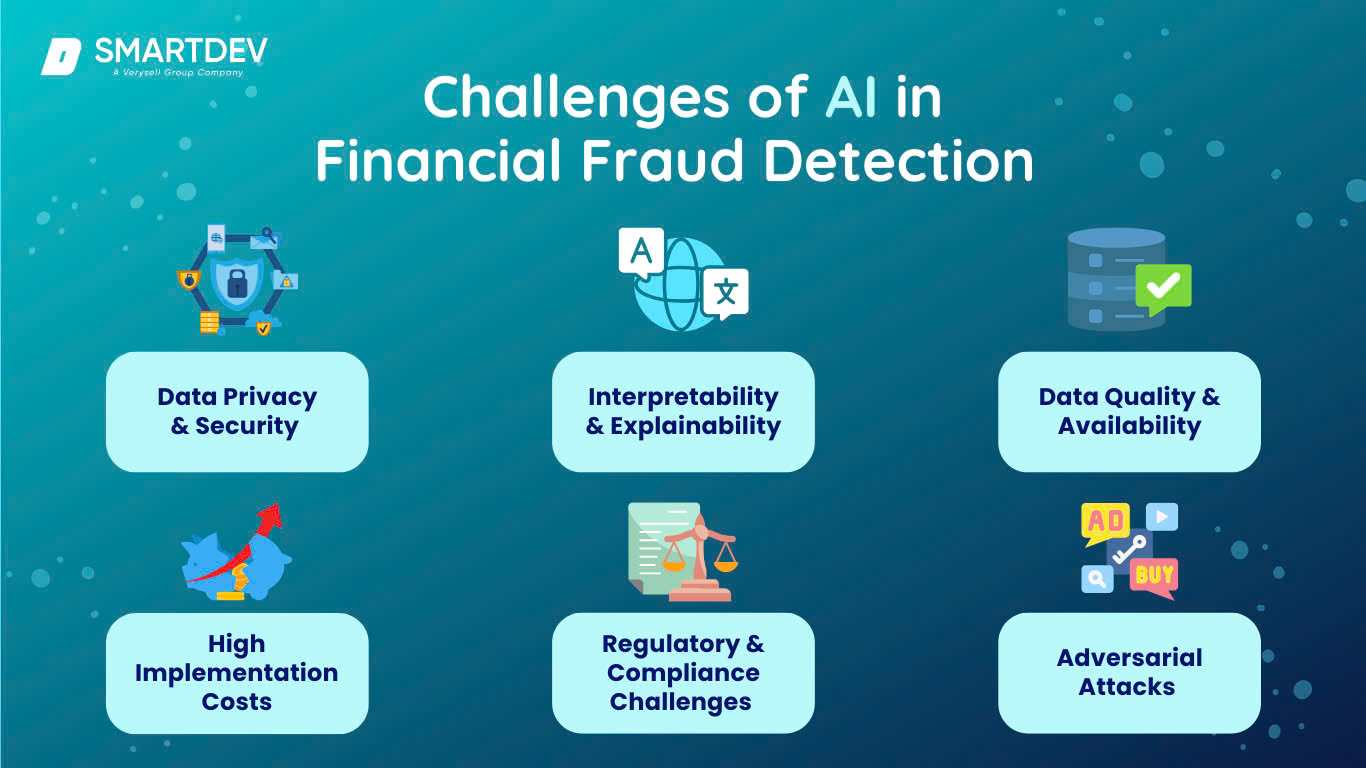
Data Privacy and Security
Implementing AI-driven fraud detection requires access to sensitive customer data. Financial institutions need to ensure that appropriate measures are in place to protect this data and comply with relevant data privacy regulations. Security breaches or unauthorized access to customer data can have severe consequences, damaging the reputation and trust of the institution.
Interpretability and Explainability
AI-driven fraud detection systems often operate as black boxes, making it difficult to interpret and explain their decision-making process. This lack of transparency can be a challenge in situations where explanations are required, such as legal proceedings or customer inquiries. Financial institutions need to strike a balance between the accuracy and interpretability of the system’s outputs.
Data Quality and Availability
AI systems are only as good as the data they’re trained on. Poor-quality data—such as incomplete, outdated, or inconsistent records—can lead to inaccurate predictions or missed fraud cases. Additionally, access to sufficient labeled data for training supervised learning models can be a hurdle, especially in new or emerging markets where historical fraud patterns may not be well-documented.
High Implementation Costs
Adopting AI for fraud detection requires significant investment in technology, infrastructure, and expertise. Smaller financial institutions or startups may struggle to allocate the resources necessary for integrating AI systems, creating a disparity in fraud prevention capabilities across the industry.
Understanding the AI development cost is essential for financial institutions to effectively plan their investment in fraud detection systems. By gaining insights into the factors influencing these expenses, organizations can allocate resources more efficiently and ensure that even smaller institutions can leverage AI to enhance their fraud prevention capabilities.
Regulatory and Compliance Challenges
AI systems must operate within the framework of financial regulations, such as anti-money laundering (AML) and data privacy laws like GDPR. Ensuring AI models comply with these regulations can be complex and may require regular audits, transparency, and documentation of how decisions are made.
Adversarial Attacks
Fraudsters are not immune to the advancements in AI technology. They can employ adversarial attacks to deceive AI-driven fraud detection systems and bypass their security measures. Financial institutions need to be aware of these attacks and continuously update their systems to mitigate the risk.
6. Case Study: PayPal’s AI-Powered Fraud Detection System
PayPal, one of the largest and most widely used online payment platforms in the world, handles millions of transactions daily. With such a massive volume of financial activity, preventing fraud and ensuring the safety of user data are critical priorities for the company. PayPal needed a solution that could scale with its growing user base while effectively detecting and preventing fraud in real-time.
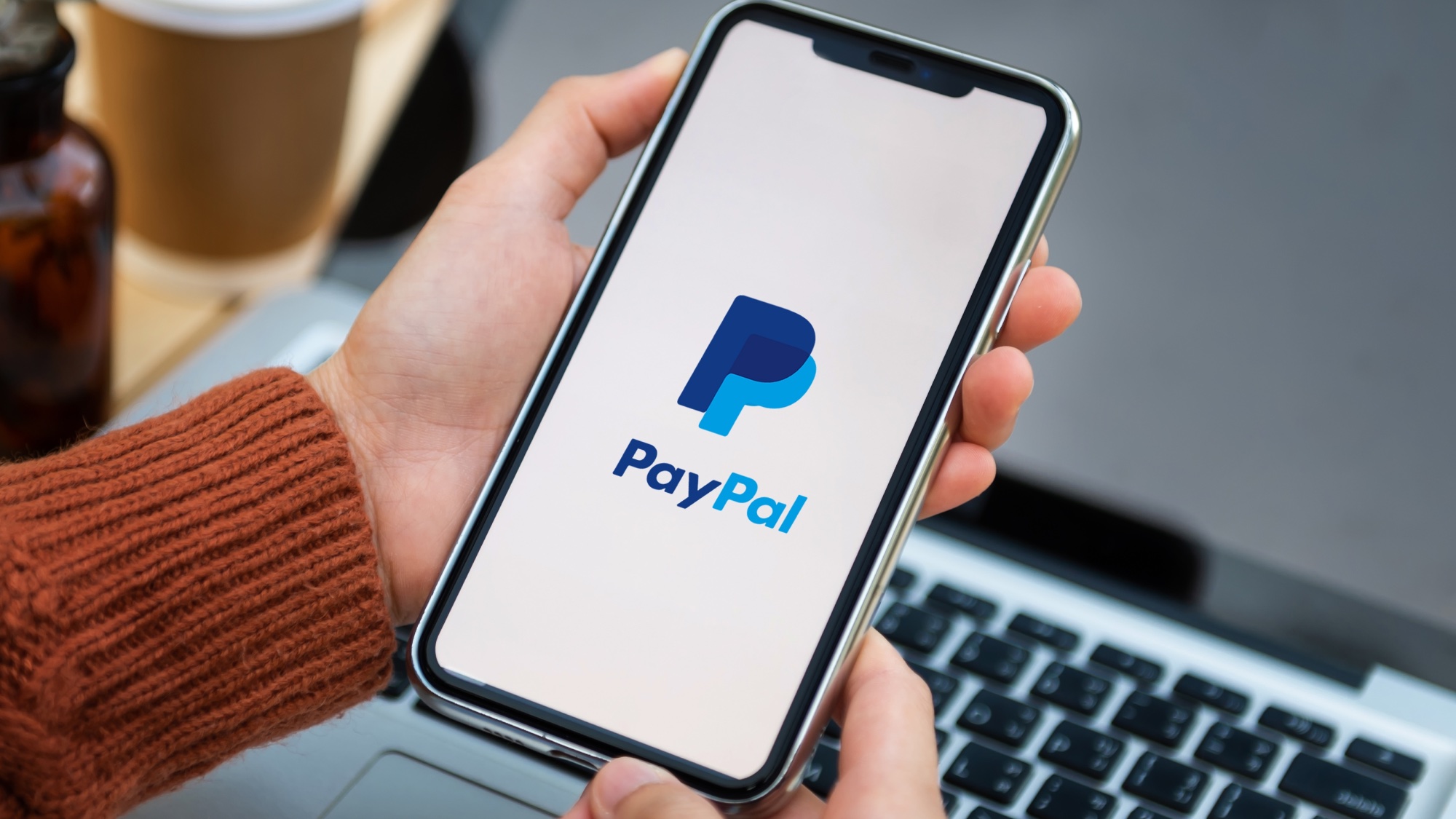
PayPal’s Challenge
Prior to implementing AI-powered fraud detection, PayPal relied on traditional fraud prevention methods that involved a combination of manual reviews and rule-based systems. While these methods were effective to some extent, they were not capable of keeping up with the volume of transactions or the increasingly sophisticated tactics employed by fraudsters. PayPal needed a solution that could automatically detect fraud with higher accuracy, improve operational efficiency, and provide better security for its users.
The AI Solution
In response to these challenges, PayPal integrated an AI-based fraud detection system that leverages machine learning algorithms and neural networks. The system was designed to analyze transaction data in real-time, flagging potentially fraudulent activities based on various data points, such as transaction amount, user location, and device information.
Key elements of the AI-driven system included:
- Real-Time Fraud Detection: PayPal’s AI models continuously monitor each transaction, using a combination of supervised and unsupervised machine learning to identify anomalies. If a transaction deviates from established patterns, the AI system flags it for review.
- Behavioral Analytics: Using AI-powered behavioral analytics, PayPal could track user behavior patterns, such as login times, IP addresses, and device types. Any significant deviation from a user’s usual behavior could indicate fraud, such as account takeovers.
- Adaptive Learning: The AI system continuously learns from new data, evolving to recognize emerging fraud patterns. This adaptability is a significant advantage over traditional fraud detection systems, which can become outdated and less effective over time.
The Results
The implementation of AI-powered fraud detection has led to significant improvements in PayPal’s fraud prevention efforts:
- Higher Accuracy and Lower False Positives: The AI system’s ability to distinguish between legitimate and fraudulent transactions has drastically reduced false positives. As a result, legitimate transactions are less likely to be flagged, improving the user experience.
- Faster Response Time: With real-time fraud detection, PayPal can block fraudulent transactions as they occur, preventing financial losses before they escalate. This has led to faster and more effective responses to potential fraud, minimizing the impact on both customers and the company.
- Scalability: The AI system has scaled effortlessly with PayPal’s growing transaction volume, handling millions of payments daily without compromising on accuracy or speed.
- Reduced Fraudulent Transactions: PayPal reported a significant reduction in fraudulent transactions due to the enhanced fraud detection system. This not only protected users but also helped PayPal maintain trust and customer satisfaction.
PayPal’s case is a prime example of how AI can be leveraged to transform fraud detection in the financial sector. PayPal has successfully improved its fraud detection capabilities, providing better security for its users and reducing the financial losses associated with fraud. As fraudsters become more sophisticated, the ability of AI systems to adapt and evolve makes them a critical tool for safeguarding the financial ecosystem.
READ MORE at PayPal AI Fraud Detection Case Study
7. Future Trends in AI for Financial Fraud Detection
By the end of 2024 and beyond, several trends are expected to shape the landscape of AI-driven fraud detection. These trends will not only define how financial institutions operate but also highlight the critical role of regulatory bodies in supporting and governing AI-based solutions.
Explainable AI (XAI)
As regulators and stakeholders demand greater transparency, Explainable AI (XAI) will become a top priority. AI systems will need to provide clear, understandable justifications for their decisions, such as why a transaction was flagged or a risk score assigned. This will help bridge the gap between sophisticated algorithms and the human users who rely on them.
Increased Use of Advanced AI Models
- Deep Learning Expansion: The use of deep learning models, such as Convolutional Neural Networks (CNNs) and Graph Neural Networks (GNNs), will grow, enabling systems to analyze even more complex patterns and behaviors.
- Hybrid Models: Combining different AI approaches—like supervised learning with anomaly detection—will enhance accuracy, reduce false positives, and adapt to emerging fraud tactics more effectively.
These advancements will make fraud detection systems more robust, and capable of handling the ever-increasing scale and complexity of fraudulent activities.
Real-Time, Cross-Border Collaboration
- Global Data Sharing: As financial transactions increasingly cross borders, AI systems will integrate global threat intelligence feeds to detect fraud patterns that span multiple countries.
- Blockchain Integration: AI solutions are expected to work in tandem with blockchain technologies to enhance transparency and traceability in financial transactions, further reducing opportunities for fraud.
Real-time collaboration across jurisdictions and organizations will be critical in identifying and disrupting fraud schemes on a global scale.
Greater Focus on Behavioral Biometrics
Behavioral biometrics—such as analyzing typing patterns, mouse movements, and touchscreen behaviors—will play a more significant role in fraud detection. Tools like BioCatch are already leading this trend, and their adoption is expected to grow rapidly in areas like online banking and e-commerce fraud prevention.
The role of regulatory bodies in supporting AI solutions
The rise of AI in fraud detection also brings challenges related to ethics, fairness, and compliance. Regulatory bodies will play a critical role in ensuring that AI solutions are not only effective but also ethical and transparent.
- Establishing AI Guidelines and Standards: the guidelines should cover ethical AI practices, transparency, and data privacy compliance.
- Encouraging Innovation: this could include grants, research funding, or tax benefits for financial institutions investing in AI-based compliance and fraud prevention solutions.
- Supporting Cross-Border Collaboration: initiatives like the Financial Action Task Force (FATF) already highlight the need for global cooperation in tackling money laundering and other financial crimes.
- Continuous Oversight and Auditing: these audits will evaluate the accuracy, fairness, and transparency of AI models used in fraud detection.
Together, they can create a secure, transparent, and trustworthy financial ecosystem that is resilient to the ever-evolving tactics of fraudsters.
8. How to Implement AI Fraud Detection in Financial Institutions
Implementing AI for fraud detection in financial institutions involves several key steps. Let’s explore the critical steps involved in implementing AI fraud detection, and how these steps help overcome the challenges faced by traditional setups.
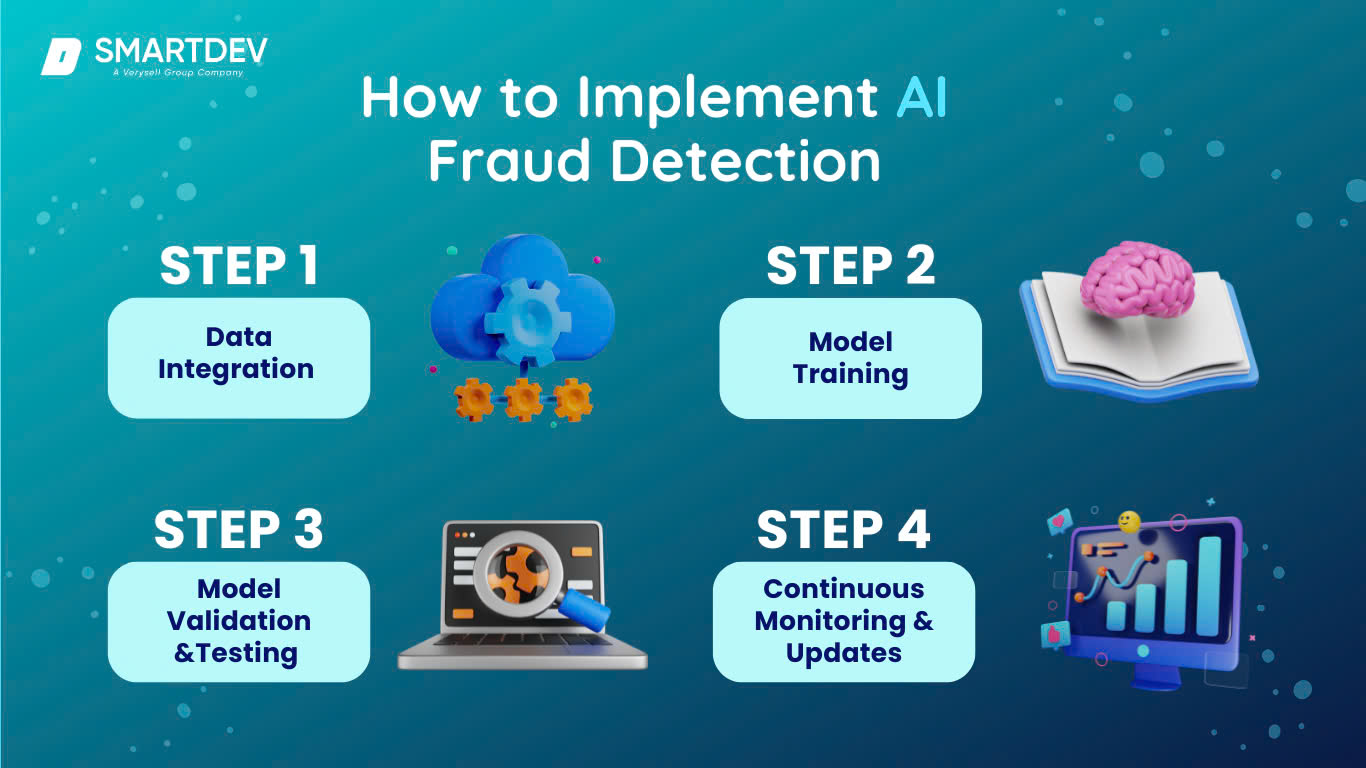
Step 1: Data Integration
The first step in implementing AI for fraud detection is collecting and integrating data from various sources within the institution. This includes transaction records, customer profiles, login histories, and external threat intelligence feeds. Data quality is paramount here, as AI models rely on accurate, up-to-date information to make reliable predictions.
Overcoming Traditional Challenges
In traditional setups, fraud detection often relies on siloed data sources and manual audits, leading to slower response times and increased risk of oversight. By adopting AI solutions, financial institutions can streamline data integration, creating a centralized repository for real-time transaction monitoring. This enables faster and more accurate detection of suspicious activities across all channels.
Best Practice
Automate the data collection and normalization process to ensure that all information, whether structured or unstructured, is processed uniformly. Tools like TensorFlow or Neo4j can help integrate and visualize complex datasets in a way that is actionable for fraud detection.
Step 2: Model Training
Once the data is integrated, the next step is training machine learning models to recognize patterns of fraudulent activity. This step involves using historical data (e.g., past fraud cases, customer behavior) to teach the AI system what constitutes “normal” vs. “suspicious” behavior. The machine learning model is fed vast amounts of data and gradually learns to detect anomalies, identify fraud networks, and assign risk scores to transactions.
Overcoming Traditional Challenges
Traditional fraud detection often relies on rule-based systems or manual monitoring, which can be rigid and limited in scope. Machine learning models, on the other hand, adapt as they are exposed to new data, learning from both past and emerging fraud tactics. This allows AI to identify sophisticated fraud schemes that would have otherwise gone unnoticed.
Best Practice
Use diverse datasets to train models, including transaction history, social behavior patterns, device fingerprinting, and even external databases (e.g., blacklists of known fraudsters). Ensure that the training data reflects a wide range of scenarios to make the AI more adaptable to various types of fraud. Tools like Scikit-learn or XGBoost can help build and refine machine learning models efficiently.
Step 3: Model Validation and Testing
Once the AI models are trained, they must undergo thorough validation and testing. This step ensures the model’s predictions are accurate and reliable. The AI system should be tested on a separate set of data (which it hasn’t seen before) to evaluate its performance, including its ability to correctly identify fraudulent activities while minimizing false positives.
Overcoming Traditional Challenges
In traditional systems, fraud detection effectiveness can be hindered by outdated or limited rule sets. AI-driven fraud detection models are continually tested and refined, ensuring they remain relevant as fraud tactics evolve. Validation should be an ongoing process, with continuous feedback loops to improve the model’s accuracy over time.
Best Practice
Regularly test the AI models on new data and real-world scenarios to ensure they are adapting to emerging fraud trends. Use techniques like cross-validation to assess the model’s robustness and fine-tune it for optimal performance.
Step 4: Continuous Monitoring and Updates
One of the key advantages of AI is its ability to continuously learn and improve. Fraud detection is not a one-time setup; it requires constant monitoring to stay ahead of fraudsters. AI models need to be updated regularly to ensure they adapt to new fraud techniques, changing customer behaviors, and evolving financial landscapes.
Overcoming Traditional Challenges
In traditional setups, fraud detection is often reactive, with delays in identifying new threats. AI, however, provides a proactive approach, allowing financial institutions to quickly detect, flag, and block new fraud schemes as they emerge. Continuous monitoring helps ensure that AI systems remain effective and resilient over time.
Best Practice
Establish an automated process for retraining models with new data, feedback from investigations, and any regulatory changes. Implement real-time monitoring dashboards to track the performance of the AI system and detect any dips in its accuracy or effectiveness. This ensures the system can adapt to emerging fraud tactics without any manual intervention.
Overcoming Traditional Setup Challenges with AI Solutions
Traditional fraud detection methods often rely on rule-based systems, manual audits, and static algorithms, which struggle to keep up with the dynamic nature of fraud. AI-powered fraud detection systems address these limitations in several ways:
- Adaptability: AI systems continuously learn from new data and evolving fraud patterns, ensuring they stay relevant and effective.
- Speed: AI can process large volumes of data in real time, reducing the time taken to detect fraud and minimize losses.
- Accuracy: By analyzing complex datasets and detecting patterns, AI can significantly reduce false positives and false negatives, offering more reliable fraud detection.
- Scalability: AI solutions scale effortlessly with increasing transaction volumes, ensuring that financial institutions can handle growing data loads without compromising on detection accuracy.
9. Conclusion: Securing the Financial Industry with AI
AI-driven fraud detection is reshaping financial security, providing financial institutions with the tools to combat increasingly sophisticated fraud techniques. By leveraging advanced algorithms and machine learning capabilities, these systems can detect fraud faster, reduce false positives, and adapt to emerging threats.
However, implementing AI-driven fraud detection comes with challenges and limitations. Financial institutions need to address data privacy and security concerns, ensure interpretability and explainability of the system’s outputs, and protect against adversarial attacks.
References
- 9 of the Biggest Financial Fraud Cases in Recent History | UN News
- Survey: 80% of Organizations Experienced Payments Fraud in 2023, Significant Uptick from Previous Year | AFP
- ACFE Report to the Nations: Organizations Lost an Average of More Than $1.5M Per Fraud Case | ACFE
- Who is Bernie Madoff? History’s Largest Ponzi Scheme Explained | Finbold
- Top 10 AI-Powered Fraud Detection Software in 2024 | Effectiv
- Top 7 Powerful AI Fraud Detection Tools for Secure Finances | Hyscaler
- The Digital Sentry: How AI Will Revolutionize Financial Fraud Investigation | Forbes
- Transparency and Privacy: The Role of Explainable AI and Federated Learning in Financial Fraud Detection | Awosika, T., Shukla, R. M., & Pranggono, B. (2023) | Cornell University
- Artificial Intelligence and Machine Learning in Financial Services | Congressional Research Service
- AI Compliance and Regulation: What Financial Institutions Need to Know | ABA Banking Journal
- Financial Firms Need to Focus on Cyber Risks Posed by AI, New York Regulator Says | The Wall Street Journal
- Harnessing machine learning fraud detection technologies | PayPal