Introduction
Operations teams face mounting pressure to optimize processes, reduce costs, and enhance efficiency in today’s fast-paced business environment. With complex supply chains, shifting customer demands, and increasing competition, AI is transforming how operations are managed. From predictive maintenance to process automation, AI is positioning itself as a critical driver of operational excellence. This guide will explore the various AI use cases revolutionizing operations in businesses today.
What is AI and Why Does It Matter in Operations?
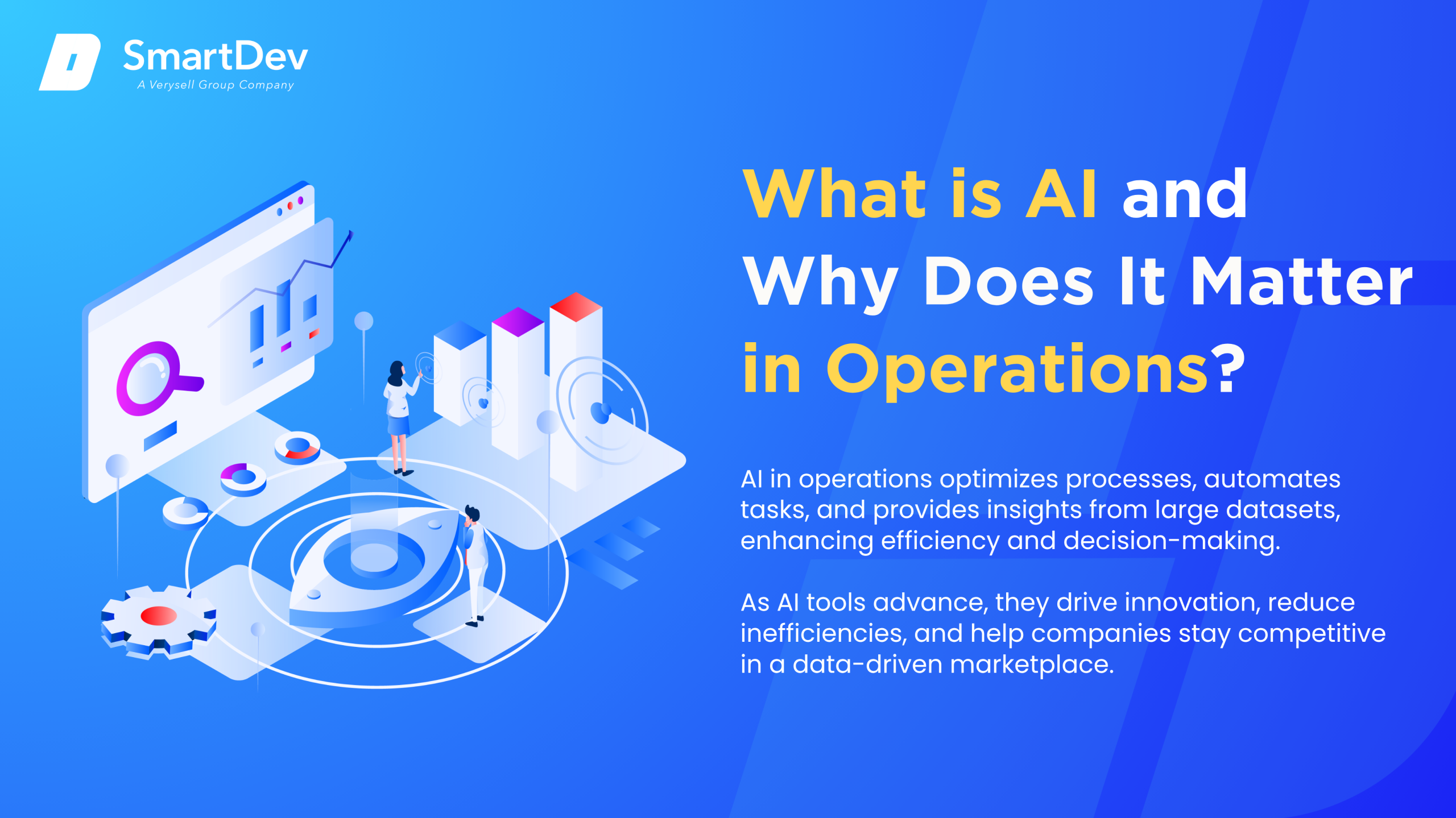
Definition of AI and its core technologies
Artificial Intelligence (AI) refers to the development of computer systems that can perform tasks typically requiring human intelligence, such as decision-making, problem-solving, and pattern recognition. Key AI technologies, including machine learning (ML), natural language processing (NLP), and computer vision, enable systems to learn from data, understand language, and even recognize images, respectively. According to IBM, AI is about equipping machines with the ability to “sense, comprehend, act, and learn” like humans, but with greater accuracy and speed.
In operations, AI takes on a specialized role by optimizing processes, automating repetitive tasks, and providing insights from massive datasets. Machine learning helps businesses predict demand, identify bottlenecks, and optimize workflows. NLP allows for advanced communication with systems, and computer vision assists with quality control and monitoring in manufacturing. AI in operations helps organizations make smarter, data-driven decisions while improving efficiency.
The Growing Role of AI in Transforming Operations
AI is rapidly becoming indispensable for operations teams. By leveraging vast amounts of data, AI helps companies monitor and streamline their operations, transforming business models and leading to significant improvements in performance. The adoption of AI tools for tasks like process automation, predictive maintenance, and inventory management is reshaping operations strategies.
For instance, AI-powered predictive analytics in operations allows for proactive decision-making by forecasting demand patterns, helping companies prepare in advance for fluctuations. Operations managers can also leverage AI to manage supply chain risks and optimize inventory levels, reducing overhead and waste. These advancements lead to more efficient operations and a competitive edge.
As AI tools become more sophisticated, they are playing an increasingly strategic role in driving operational innovation. They provide insights into how processes can be improved, reducing inefficiencies and boosting productivity. More companies are now integrating AI into their operations infrastructure, enabling them to stay competitive in an increasingly data-driven marketplace.
To see how AI can be applied in real-world workflow optimization, check out our case study on Smart Consulting for Efficient Inventory and Workflow Management.
Key Statistics and Trends Highlighting AI Adoption in Operations
AI adoption in operations is gaining momentum, with industries across the board reaping significant benefits. According to a report by McKinsey, 63% of companies implementing AI in operations have experienced a measurable improvement in performance. Specifically, AI adoption in supply chain and manufacturing has been shown to reduce operational costs by up to 25%, a major competitive advantage in high-margin industries.
Furthermore, the global market for AI in operations is expected to grow rapidly, with AI-driven process automation projected to reach $12 billion by 2025, according to a report by MarketsandMarkets. This exponential growth highlights the increasing reliance on AI to streamline operations and enhance efficiency across industries.
Business Benefits of AI in Operations
AI provides transformative benefits that solve key pain points in operations. These include automating routine tasks, improving forecasting accuracy, and driving process optimization. Below are the main benefits AI brings to operational teams.
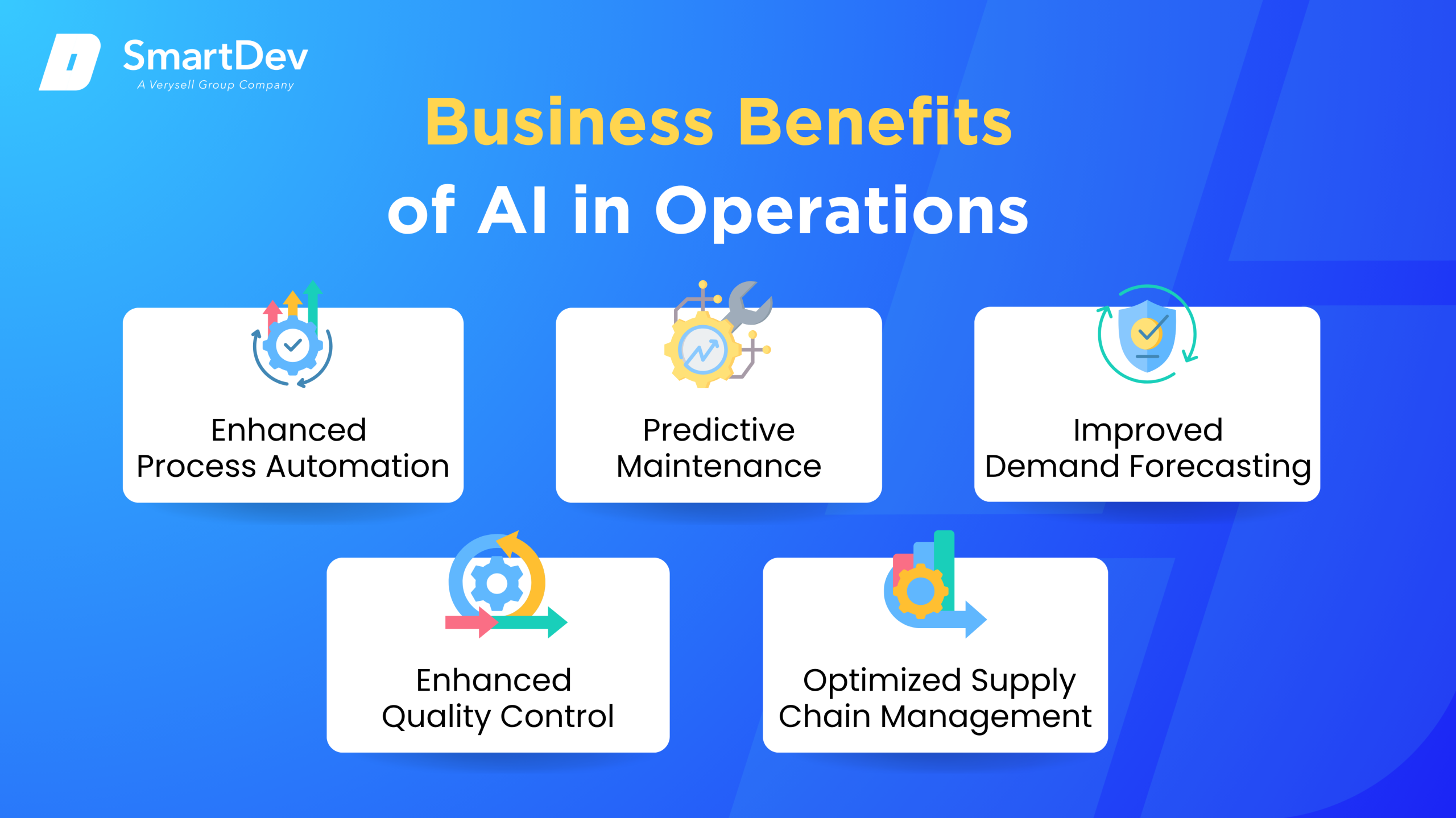
1. Enhanced Process Automation
AI enables automation across a wide range of operational tasks, from inventory management to scheduling. This reduces the need for manual intervention, which can lead to errors and inefficiencies. With AI, businesses can automate repetitive workflows, such as order fulfilment and quality control, improving the speed and consistency of operations.
For instance, AI-powered robotic process automation (RPA) systems can be used to process invoices, update inventory, or even respond to customer inquiries. These automations free up time for employees to focus on strategic tasks, which improves both productivity and decision-making.
To see how workflow automation powered by AI is revolutionizing business processes, explore our in-depth article on AI Workflow Automation.
2. Predictive Maintenance
In operations, equipment downtime can be costly and disruptive. Predictive maintenance, powered by AI, allows businesses to anticipate equipment failures before they happen, significantly reducing unplanned downtime. AI systems use sensors to gather real-time data on machine performance, and then machine learning algorithms analyze this data to predict potential issues.
In a manufacturing setting, AI tools can monitor machinery for abnormal vibrations, temperature fluctuations, or other anomalies, alerting managers to perform maintenance before costly breakdowns occur. This not only reduces operational disruptions but also extends the lifespan of equipment.
3. Improved Demand Forecasting
Accurate demand forecasting is essential to optimizing inventory and ensuring the smooth operation of the supply chain. AI-powered forecasting models analyze historical sales data, market trends, and other variables to predict future demand patterns with greater accuracy than traditional methods. These models help operations teams plan for peak periods, adjust inventory levels, and mitigate the risk of stockouts or overstocking.
AI-driven supply chain optimization tools can predict demand fluctuations, allowing for real-time inventory adjustments. This enables companies to meet customer demand without overstocking, improving cash flow and reducing storage costs.
4. Enhanced Quality Control
AI is revolutionizing quality control in manufacturing and production environments. With the help of computer vision, AI systems can inspect products at a much higher speed and accuracy than human inspectors. These systems can detect defects, measure product dimensions, and ensure that products meet strict quality standards.
In a practical scenario, AI systems can scan assembly lines for defective products or irregularities, alerting operators before faulty items reach customers. This improves product quality, reduces returns, and ensures compliance with regulatory standards.
5. Optimized Supply Chain Management
AI optimizes supply chain management by offering real-time visibility and providing actionable insights to reduce inefficiencies. AI-powered tools can analyze vast amounts of data from suppliers, logistics, and inventory systems to recommend optimal routes, manage risks, and forecast disruptions.
AI tools can predict potential supply chain disruptions due to weather, geopolitical events, or other factors. With this foresight, operations teams can adjust supply chain strategies in real time, ensuring that materials are delivered on time and costs are kept under control.
Challenges Facing AI Adoption in Operations
While AI offers significant potential in transforming operations, the adoption of AI-driven solutions is not without its challenges. Below are some of the key barriers businesses face in implementing AI in their operations.
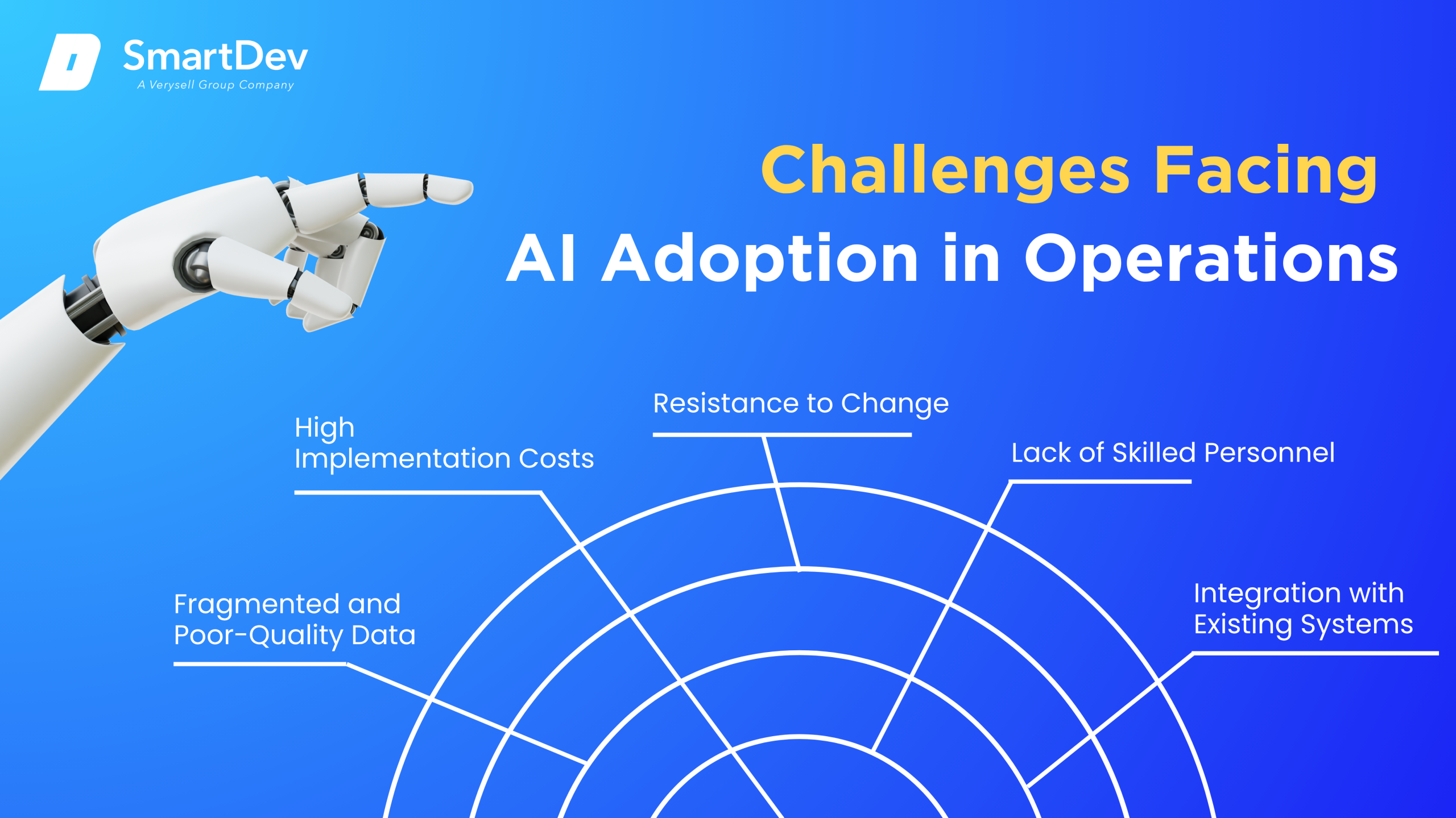
1. Fragmented and Poor-Quality Data
AI systems rely on high-quality, organized data to generate accurate insights. In many operations environments, data is scattered across various systems, spreadsheets, and formats, making it difficult for AI tools to process and analyze. Without centralized, high-quality data, AI models cannot deliver reliable results.
Organizations need to invest in data governance and integration tools to unify their data sources, ensuring that AI algorithms can work with accurate, consistent data. Without this foundation, businesses risk underutilizing AI or making decisions based on flawed insights.
2. High Implementation Costs
While the benefits of AI in operations are significant, the initial investment required for AI tools can be substantial. The costs associated with developing, testing, and implementing AI solutions can be a barrier for smaller organizations or those operating on tight budgets. Additionally, companies must invest in the necessary infrastructure and expertise to support AI integration.
To overcome this challenge, businesses must evaluate the long-term ROI of AI investments and consider phased implementations, starting with smaller AI projects that can deliver immediate value before scaling up.
3. Resistance to Change
Despite the clear benefits, some organizations struggle with employee resistance to AI adoption. Employees may fear job displacement or be reluctant to adopt new technologies. This resistance can slow down the integration process and hinder the potential advantages AI offers.
Companies must prioritize change management efforts, including employee training, transparent communication, and clear demonstrations of AI’s role in enhancing, not replacing human work. This ensures that AI becomes a tool for employees to use, not something to fear.
4. Lack of Skilled Personnel
AI adoption requires specialized knowledge in data science, machine learning, and AI algorithms. Many businesses face a shortage of skilled personnel capable of managing AI implementation and ongoing optimization. Without this expertise, organizations may struggle to derive maximum value from their AI investments.
To address this challenge, companies should invest in upskilling their current workforce and consider partnerships with AI consultants or third-party providers who can guide the implementation process and provide ongoing support.
5. Integration with Existing Systems
Integrating AI into existing operational systems can be a complex and time-consuming process. Legacy systems may not be compatible with AI tools, requiring significant upgrades or replacements. This integration process can disrupt normal operations and lead to downtime.
For smooth AI adoption, businesses must prioritize careful planning and phased integration, ensuring that AI tools complement existing systems rather than complicate them. Additionally, partnerships with AI solution providers who understand industry-specific requirements can ease the transition process.
Specific Applications of AI in Operations
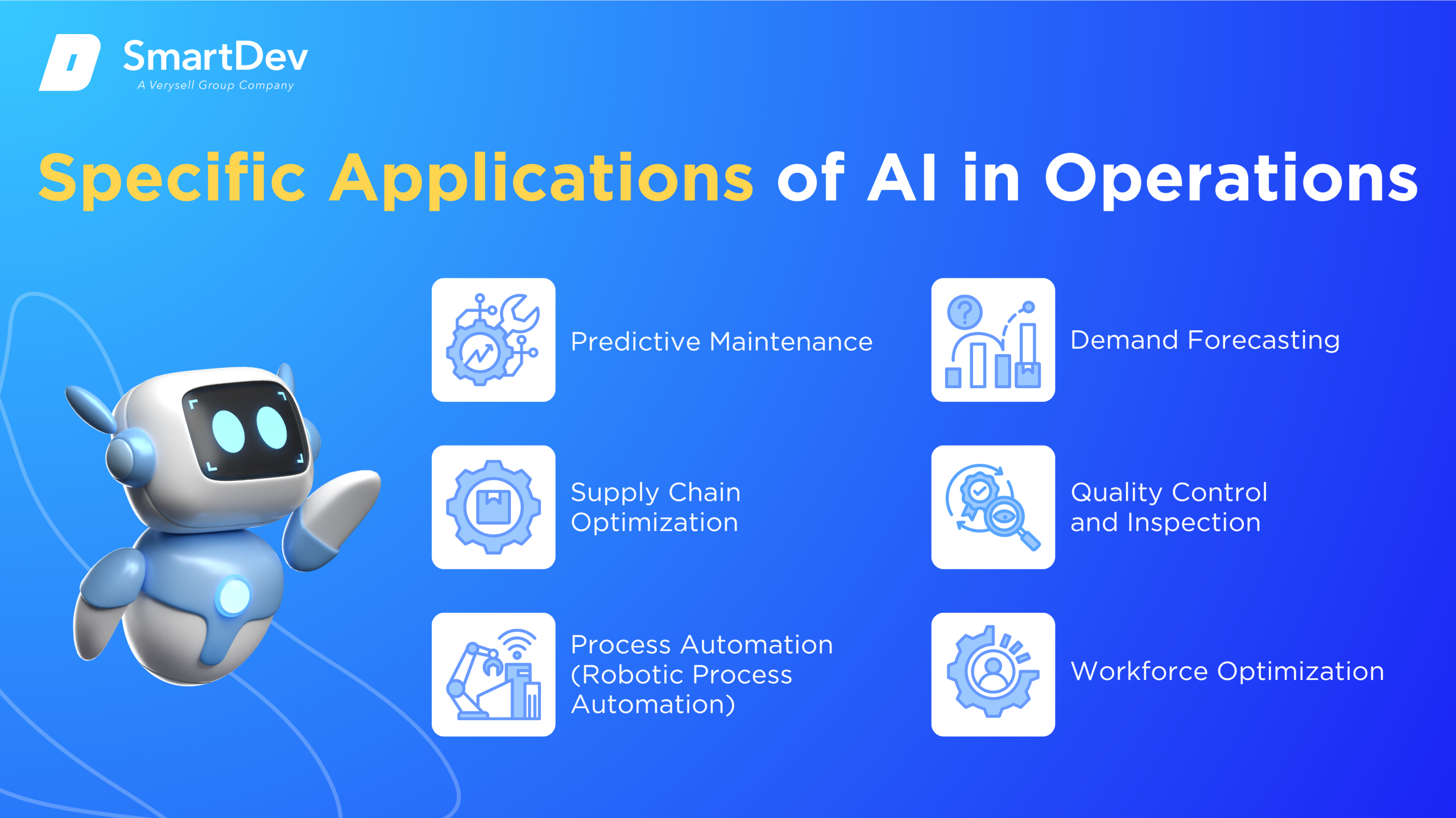
1. Predictive Maintenance
Predictive maintenance uses AI to forecast equipment failures and schedule maintenance activities before issues arise. It analyzes data from sensors, historical performance, and environmental factors to detect patterns and predict when maintenance is needed. This approach helps businesses avoid unplanned downtime and extends the life of their machinery, ensuring smoother operations.
AI models rely on machine learning algorithms that learn from historical data, recognizing early signs of wear and tear or other anomalies. The system integrates with the organization’s workflow by alerting maintenance teams about potential issues, allowing them to act proactively. By scheduling maintenance at the right time, companies can optimize resources and reduce repair costs.
The operational value of predictive maintenance lies in reducing unplanned downtime, improving equipment reliability, and minimizing maintenance costs. It allows businesses to shift from reactive to proactive maintenance strategies, resulting in better asset management and increased productivity. Additionally, it enhances safety by preventing unexpected breakdowns.
Real-World Example: General Electric (GE) uses AI for predictive maintenance through its Digital Wind Farm program, which analyzes turbine data to predict when maintenance is needed. This has significantly reduced maintenance costs and improved turbine uptime. GE reports that this predictive approach has saved millions in operational costs by minimizing unplanned downtime and ensuring optimal performance.
2. Supply Chain Optimization
AI is transforming supply chain management by predicting demand, optimizing inventory, and improving logistics. AI-powered systems analyze historical data, real-time sales, and market trends to forecast demand with higher accuracy. These insights help companies optimize their supply chains by adjusting production schedules and stock levels to meet fluctuating demand.
AI algorithms also optimize logistics by analyzing transportation routes and finding the most efficient paths for delivery. This reduces delivery times, minimizes transportation costs, and helps companies maintain a steady flow of goods. As AI learns from data patterns, its predictions become more accurate over time, ensuring that supply chains are agile and efficient.
The strategic value of AI in supply chain optimization includes cost reduction, better resource allocation, and improved responsiveness to customer needs. By anticipating demand and adjusting operations accordingly, companies can minimize excess inventory and prevent stockouts. AI also enhances decision-making by providing real-time visibility into supply chain operations.
Real-World Example: Walmart uses AI to optimize its supply chain by predicting demand and adjusting inventory levels accordingly. With machine learning algorithms, Walmart can forecast product demand at a granular level, reducing excess stock and improving order fulfilment rates. This AI-driven approach has led to better inventory management, cost savings, and enhanced customer satisfaction.
3. Process Automation (Robotic Process Automation)
Robotic Process Automation (RPA) powered by AI automates repetitive, rule-based tasks that were traditionally performed by humans. This includes tasks such as data entry, invoice processing, and payroll management. By automating these processes, organizations can reduce human error, increase efficiency, and free up employees to focus on more strategic tasks.
AI-powered RPA systems use machine learning and natural language processing (NLP) to handle more complex workflows, such as processing unstructured data or managing exceptions. These bots learn over time and improve their performance by adapting to new processes and tasks. RPA can be integrated into various business functions, including HR, finance, and customer service, to streamline operations.
The operational value of AI in RPA is in its ability to reduce operational costs, enhance accuracy, and accelerate workflows. AI-driven automation can handle high volumes of repetitive tasks at scale, improving efficiency while minimizing human resource expenditure. It also enables quicker decision-making by processing data faster and more accurately than human workers.
For a detailed explanation of how robotic process automation and machine learning synergize to drive intelligent automation, explore our technical guide on RPA and Machine Learning.
Real-World Example: Siemens leverages AI in RPA to automate back-office processes such as invoicing and payroll management. By implementing AI-powered bots, Siemens has automated over 40% of its administrative workflows, resulting in cost savings and faster processing. The company has seen a 30% reduction in operational costs in certain departments, enhancing productivity and efficiency.
4. Demand Forecasting
Demand forecasting is a crucial operational function that can be greatly enhanced by AI. AI models predict future customer demand by analyzing historical data, market trends, seasonality, and external factors such as economic shifts or weather patterns. These predictions help businesses adjust production plans and stock levels to meet customer demand more accurately.
AI uses machine learning algorithms to continuously learn from new data, improving the accuracy of its forecasts over time. It integrates seamlessly with inventory management and production planning systems, ensuring that businesses can meet customer demand without overstocking. AI-driven demand forecasting allows businesses to make smarter decisions and optimize supply chain operations.
The operational benefits of AI in demand forecasting include more accurate predictions, reduced inventory costs, and improved service levels. By predicting demand more accurately, companies can avoid excess inventory or stockouts, ensuring products are available when customers need them. This leads to improved operational efficiency and customer satisfaction.
Real-World Example: Coca-Cola uses AI for demand forecasting to optimize its inventory and production schedules. By analyzing consumer purchasing data and market trends, Coca-Cola’s AI models predict demand with greater accuracy. This AI-driven approach has helped the company reduce excess inventory and improve its ability to meet customer demand on time, resulting in significant cost savings.
5. Quality Control and Inspection
AI is revolutionizing quality control and inspection by automating the process of detecting defects in products during production. Computer vision systems, powered by AI, analyze images or videos of products to identify defects such as scratches, dents, or other imperfections. These AI-powered systems can perform inspections much faster and more accurately than human inspectors.
AI-driven quality control systems use deep learning algorithms that can detect subtle defects that may be missed by the human eye. These systems are trained on large datasets of images to identify patterns and variations that indicate defects. In addition to detecting problems, AI systems can also provide real-time feedback to production lines, ensuring that defects are corrected promptly.
The operational value of AI in quality control includes improved product consistency, reduced waste, and faster detection of defects. By automating inspections, businesses can maintain high-quality standards while increasing production speed. This leads to better product quality, higher customer satisfaction, and cost savings from fewer returns and defects.
Real-World Example: Tesla uses AI-driven computer vision to inspect its electric vehicles during production. The AI system scans for defects in the car’s body, identifying imperfections that would otherwise require manual inspection. This automation speeds up the quality control process and reduces the likelihood of defects reaching customers, ensuring higher product quality and customer satisfaction.
6. Workforce Optimization
AI is transforming workforce management by optimizing employee scheduling, task assignments, and resource allocation. AI systems analyze employee data, project requirements, and business needs to ensure that the right people are assigned to the right tasks at the right time. This improves productivity, reduces costs, and ensures that workforce resources are maximized effectively.
AI models use historical data, machine learning algorithms, and optimization techniques to create efficient work schedules that balance employee availability and skillsets. These systems also help businesses plan for fluctuations in workload by predicting staffing needs based on demand forecasts. By automating scheduling and task assignments, businesses can ensure smoother operations and better employee engagement.
The operational benefits of AI in workforce optimization include increased efficiency, reduced labor costs, and improved employee satisfaction. AI enables businesses to allocate resources more effectively, ensuring that the right skills are available when needed. This results in greater operational efficiency and cost savings across various departments.
Real-World Example: Target uses AI to optimize workforce scheduling and task assignments in its retail stores. By using AI to analyze customer traffic and sales data, Target can optimize shift scheduling to meet demand while keeping labor costs low. The result is a more efficient workforce, with improved customer service and better overall productivity in stores.
Examples of AI in Operations
Real-World Case Studies
AI is not just a theoretical concept in operations; it’s being implemented in real-world business scenarios, proving its value across various industries. Real-world applications of AI in operations highlight how it can drive measurable results, streamline workflows, and support smarter decision-making processes.
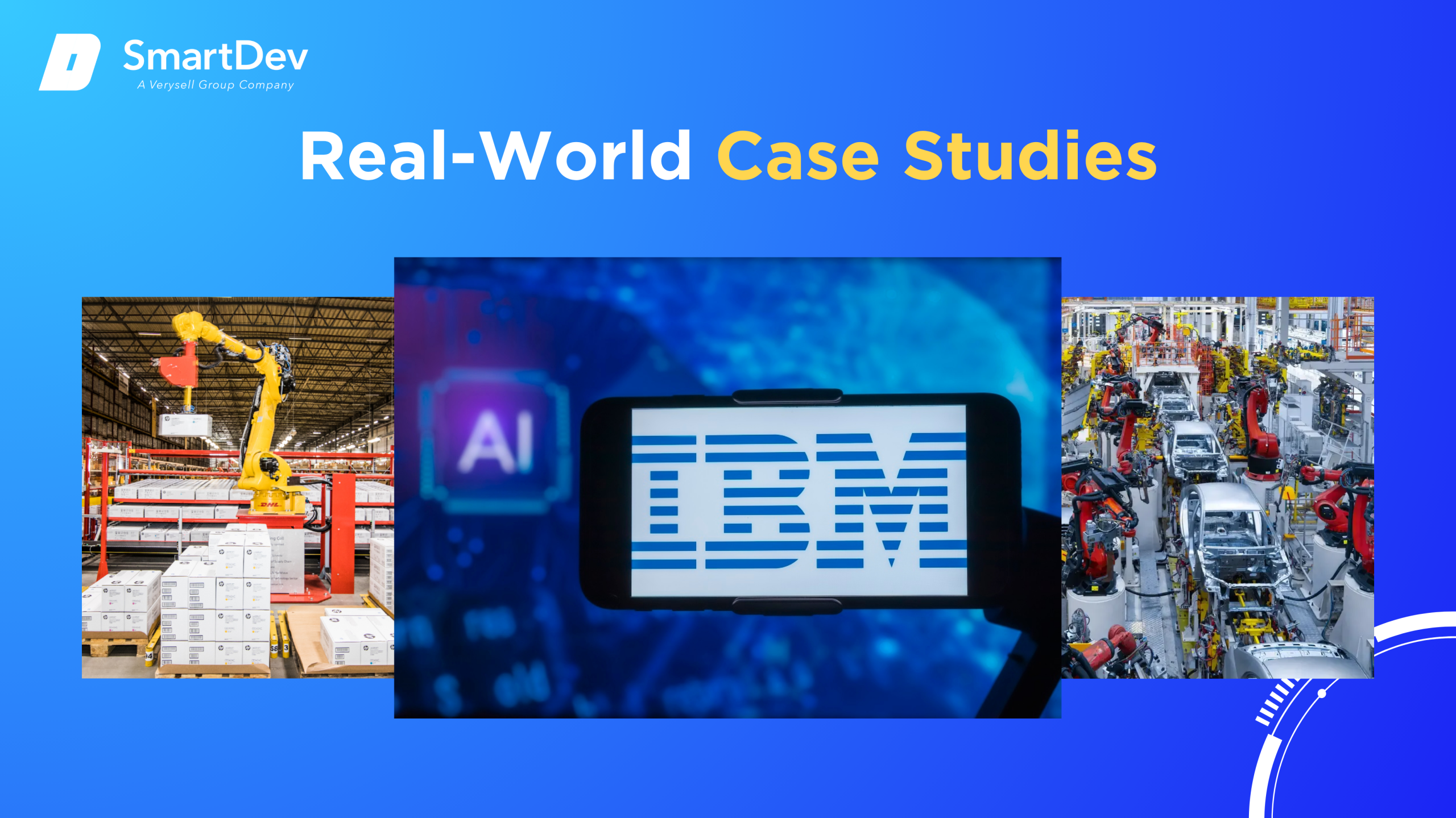
1. IBM: AI for Operational Efficiency in IT Infrastructure
IBM utilizes AI in its IT infrastructure operations through its Watson AI platform. By integrating AI-powered predictive analytics and automation tools, IBM has enhanced its ability to manage complex IT systems, reducing downtime and improving system performance. The use of Watson’s machine learning algorithms enables the company to identify and resolve issues before they impact operations, saving considerable costs related to system maintenance and unexpected failures.
Through AI-driven tools, IBM has improved operational efficiency, reduced response times, and increased the speed of issue resolution. The company reports a significant reduction in operational costs while maintaining service reliability. IBM’s AI-enhanced infrastructure solutions are pivotal in delivering reliable, cost-efficient IT services to clients worldwide.
2. DHL: AI for Supply Chain Management Optimization
DHL, a leader in logistics, uses AI-driven solutions to streamline its supply chain management processes. The company leverages AI for route optimization, demand forecasting, and warehouse management to improve efficiency and reduce delivery times. By utilizing predictive analytics, DHL can more accurately forecast demand and adjust inventory levels in real time, ensuring the right products are in the right place at the right time.
This AI-powered optimization has led to reduced transportation costs, faster deliveries, and improved customer satisfaction. As a result, DHL has significantly reduced supply chain disruptions and seen a notable increase in on-time deliveries, strengthening customer loyalty and driving operational savings.
3. Toyota: AI in Manufacturing and Production Lines
Toyota has adopted AI in its manufacturing and production lines to increase operational efficiency and reduce defects. By employing machine learning algorithms, Toyota has automated quality control processes, allowing real-time detection of defects on the production line. AI systems identify potential flaws in parts and components, ensuring that only products that meet Toyota’s stringent quality standards reach the market.
This AI-driven approach has significantly reduced defects and increased production line throughput. Toyota has seen a decrease in quality-related recalls, improved efficiency, and a reduction in waste, all contributing to better operational performance and greater profitability.
Innovative AI Solutions
AI is continually evolving, introducing innovative solutions that are transforming operations in unprecedented ways. These advanced technologies help companies automate complex processes, enhance predictive capabilities, and significantly boost operational efficiency. As AI continues to advance, even more sophisticated and integrated solutions are expected to revolutionize various aspects of business operations.
AI-powered autonomous vehicles are revolutionizing logistics, especially in warehouses and distribution centers. These vehicles utilize machine learning to navigate complex environments and optimize delivery routes, leading to faster and more cost-effective operations. By incorporating autonomous systems, companies can lower labor costs, reduce human error, and improve accuracy in deliveries. This ultimately makes logistics more efficient.
Emerging AI applications like process mining and decision support systems are reshaping how businesses optimize workflows and make data-driven decisions. Process mining uses AI to track and analyze business processes, identifying inefficiencies and bottlenecks that can be streamlined for better performance.
Meanwhile, AI-driven decision support systems analyze large datasets in real time, providing actionable insights that help executives optimize strategies and respond swiftly to market changes.
Learn more about our manufacturing tech solutions to see how we help factories integrate AI, automation, and smart systems into their operations.
AI-Driven Innovations Transforming Operations
Emerging Technologies in AI for Operations
Generative AI is revolutionizing operations by helping design new products, improve content creation, and optimize workflows. In manufacturing, it aids engineers in designing efficient components and predicting machine failures before they occur, reducing costly downtime. By harnessing predictive AI capabilities, businesses can improve equipment longevity and operational reliability.
Another key technology is computer vision, which enhances visual data analysis for real-time quality control and anomaly detection. In logistics, AI-powered computer vision systems track inventory, monitor warehouse conditions, and ensure proper handling, minimizing human error. This technology boosts operational effectiveness while promoting safety and compliance across industries.
AI’s Role in Sustainability Efforts
AI is playing a key role in helping businesses meet their sustainability goals by using predictive analytics to reduce waste and optimize resource use. By analyzing past consumption patterns, AI can predict demand, ensuring that materials are used efficiently without overproduction or excess stock. This approach not only supports sustainability but also drives long-term cost savings.
Additionally, AI is optimizing energy consumption through smart systems that manage heating, cooling, lighting, and equipment. These systems adjust operations based on real-time data, leading to reduced energy usage and lower operational costs. For instance, AI in smart grids enables businesses to track and optimize power usage, reducing their carbon footprint and enhancing overall sustainability.
How to Implement AI in Operations
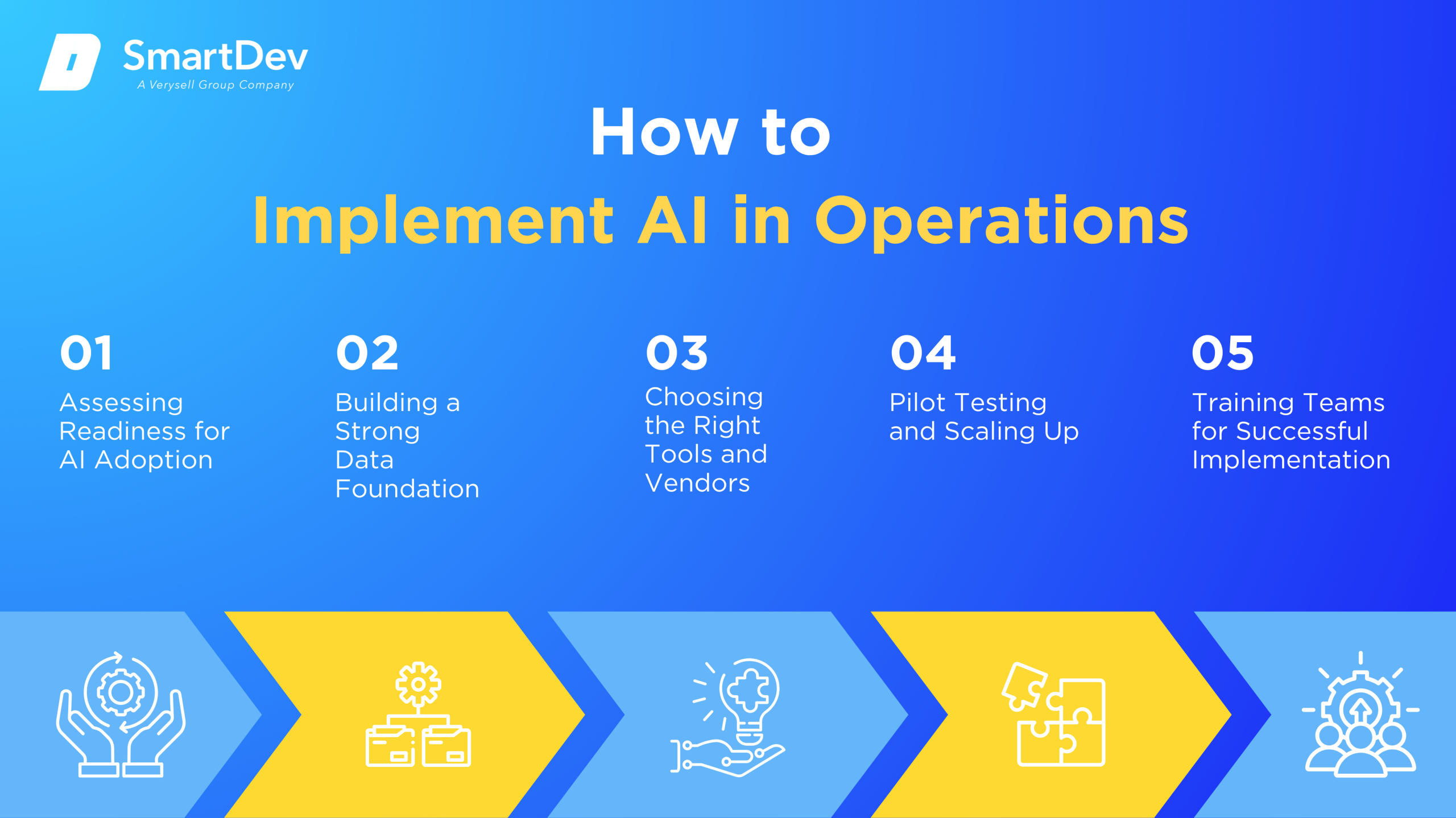
Step 1. Assessing Readiness for AI Adoption
The first step to adopting AI is determining if your business is ready to integrate this technology effectively.
This involves pinpointing areas within operations where AI can alleviate inefficiencies, such as automating repetitive tasks or optimizing resource management.
By analyzing current workflows and identifying bottlenecks, businesses can uncover where AI can have the most significant impact, whether through automation or enhanced decision-making.
Step 2. Building a Strong Data Foundation
A solid data foundation is essential for successful AI implementation, as AI thrives on high-quality, well-organized data.
Companies must gather relevant data from various operational sources, such as customer feedback, production lines, and sales, ensuring it is accurate and reliable.
However, simply collecting data is not enough; it must be cleaned, standardized, and maintained regularly to ensure that AI algorithms can function optimally and continuously provide actionable insights.
Learn more about best practices in building a scalable data infrastructure in our guide on Data-Driven Success.
Step 3. Choosing the Right Tools and Vendors
The success of AI implementation hinges on selecting the right tools and vendors for your operations.
Businesses must evaluate AI platforms that align with their specific needs, ensuring easy integration with existing systems and scalability for future growth.
Additionally, it’s important to assess the support offered by vendors, including training, customization, and post-implementation services, to guarantee the AI solution remains effective over time.
Step 4. Pilot Testing and Scaling Up
Before fully deploying AI across your operations, pilot testing is critical to evaluate how well the technology performs in real-world scenarios.
These tests identify potential challenges, allowing businesses to refine AI systems and ensure they integrate seamlessly with existing processes.
After a successful pilot, businesses can gradually scale AI adoption across wider operations, continuously monitoring performance and addressing any issues to maintain smooth operations and foster continuous improvement.
Step 5. Training Teams for Successful Implementation
AI implementation is not just about technology, it’s about empowering employees to make the most of it.
Businesses must invest in training programs that teach employees how AI tools integrate into their workflows and how these technologies can boost productivity.
By cultivating a culture of continuous learning and providing resources for ongoing development, businesses ensure employees feel confident using AI, which drives greater adoption and maximizes the technology’s potential.
Measuring the ROI of AI in Operations
Measuring the return on investment (ROI) of AI in operations is essential to determine the effectiveness of AI technologies and justify further investments.
Businesses need to track various key performance indicators (KPIs) to assess the impact of AI on operational efficiency, productivity, and cost savings.
Key Metrics to Track Success
To measure AI’s ROI, businesses should track several metrics, starting with productivity improvements. AI can automate tasks that were previously time-consuming and prone to human error, leading to more efficient workflows and higher output. By quantifying time savings, increased throughput, and improved accuracy, businesses can calculate the productivity gains from AI integration.
Cost savings are another key metric. AI can significantly reduce operational costs by automating routine tasks, optimizing resource utilization, and minimizing waste. By comparing the costs of AI deployment with the savings achieved through automation, businesses can determine the overall cost-effectiveness of AI technologies in operations. Moreover, AI can lead to savings in areas like energy consumption and supply chain management, offering businesses the potential for long-term financial benefits.
Case Studies Demonstrating ROI
Real-world case studies offer tangible proof of AI’s ROI in operations. For instance, a global manufacturer used AI-driven predictive maintenance to monitor equipment, reducing downtime by 30% and saving millions in maintenance costs. This example shows how AI can proactively prevent issues, leading to cost savings and better resource use.
Similarly, a major retailer implemented AI to optimize inventory management, reducing overstocking by 20% and automating stock replenishment. This resulted in lower inventory costs and increased sales, demonstrating AI’s ability to enhance efficiency and profitability.
Common Pitfalls and How to Avoid Them
While AI presents significant opportunities for operational improvements, there are common pitfalls businesses should avoid. One major challenge is the over-reliance on AI without proper human oversight. While AI can automate many tasks, human expertise is still needed for strategic decision-making and managing unexpected situations. Ensuring a balance between AI-driven automation and human judgment is crucial for achieving optimal outcomes.
Another pitfall is failing to align AI implementation with business goals. To maximize ROI, businesses must ensure that AI tools are aligned with their operational objectives, whether it’s reducing costs, improving quality, or increasing throughput. Clear communication and a well-defined strategy are key to avoiding these pitfalls and ensuring that AI delivers the expected benefits.
Future Trends of AI in Operations
Predictions for the Next Decade
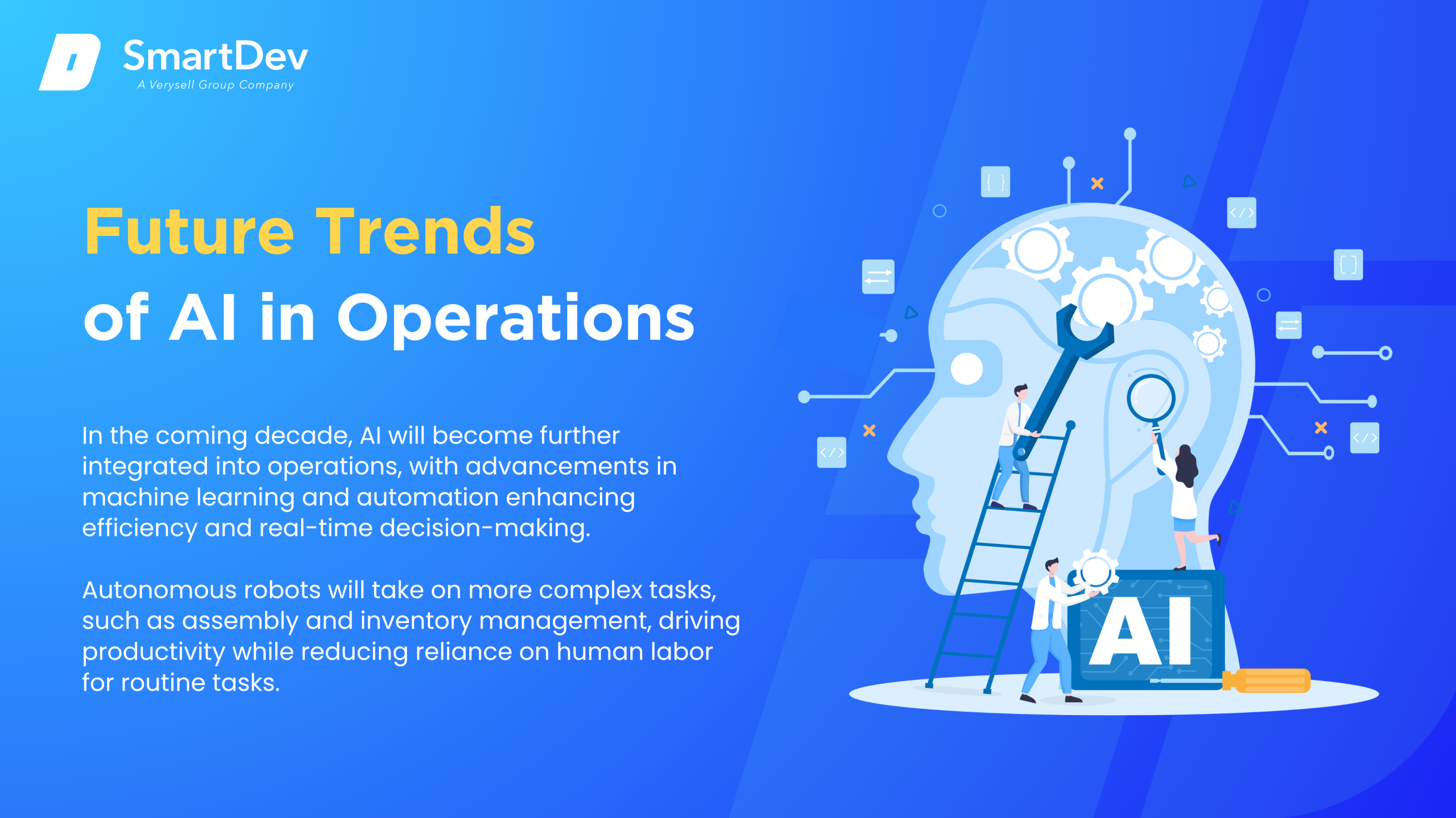
Over the next decade, AI will become even more integrated into operations, with advancements in machine learning and automation driving greater efficiency. Predictive analytics will improve, allowing businesses to forecast trends and respond to changes in real-time. AI’s role in supply chain optimization will also expand, with smart systems adjusting production schedules based on demand and disruptions.
Additionally, autonomous robots will increasingly take on complex tasks across warehouses and production floors, such as assembly, inventory management, and customer service. These innovations will help businesses boost productivity and reduce dependence on human labor for routine tasks.
How Businesses Can Stay Ahead of the Curve
To stay ahead of the curve, businesses must prioritize continuous learning and be flexible in adopting new AI technologies. By regularly evaluating AI tools and staying updated on industry trends, businesses can quickly adapt and implement the latest AI applications. Creating an innovation-driven culture encourages experimentation and helps companies remain agile as technology evolves.
Moreover, forming strategic partnerships with AI vendors and research institutions can provide businesses with early access to cutting-edge advancements. These collaborations ensure that businesses are equipped to adopt the most advanced AI solutions, allowing them to stay competitive and lead in their respective industries.
Conclusion
Key Takeaways
AI is transforming operations by streamlining processes, improving decision-making, and enhancing efficiency across various industries. With technologies like predictive analytics and automation, businesses can optimize resources, reduce costs, and automate routine tasks, driving greater productivity.
AI’s role in operations will expand, making it a critical tool for businesses looking to stay competitive and succeed in a fast-changing market.
Moving Forward: A Path to Progress
As AI technology continues to evolve, the future of operations lies in continuous innovation and strategic adoption. To stay ahead, businesses must invest in robust data foundations, stay informed about the latest AI advancements, and foster a culture of adaptability and learning. By integrating AI into key operational processes, companies can drive efficiency, reduce costs, and improve decision-making to maintain a competitive edge.
At SmartDev, we specialize in delivering cutting-edge AI solutions that transform operations and drive business success. From predictive analytics to automated processes, our team empowers businesses to harness AI to streamline operations, boost productivity, and remain agile in a fast-paced market.
Contact us today to explore how AI can revolutionize your operations, optimize resources, and help your business thrive. Let us partner with you in building a smarter, more efficient future powered by AI.
—
References:
- What is artificial intelligence (AI)? | IBM
- Superagency in the workplace: Empowering people to unlock AI’s full potential | McKinsey & Company
- AI Agents Market Size, Share and Global Forecast to 2030 | MarketandMarkets
- Artificial intelligence in supply chain management: A systematic literature review | Journal of Business Research
- How Walmart Uses AI to Optimize Inventory Management | Redress Compliance
- Siemens GBS China Boosts Operational Efficiency by Deploying RPA | UiPath
- Tesla retreats from next-generation ‘gigacasting’ manufacturing process | CNBC
- AI Is No Longer an Option-It’s the Future of Retail | PwC