Introduction to AI Credit Scoring
Definition and Evolution of Credit Scoring
Credit scoring is the process by which financial institutions assess the creditworthiness of an individual or entity. Traditionally, credit scores have been based on data such as payment history, credit utilization, length of credit history, and recent inquiries into the individual’s credit profile. While this method has been the cornerstone of lending for decades, it has its limitations, often leaving out valuable insights.
Credit scoring systems have evolved significantly over time, from basic FICO scores to more complex models. However, the latest evolution is AI-based credit scoring, which uses advanced machine learning algorithms to create a more nuanced and dynamic understanding of a borrower’s financial behavior.
The Role of AI in Modernizing Credit Evaluations
AI is now transforming credit scoring by going beyond just historical data. It introduces the ability to analyze vast amounts of real-time data, identify patterns, and provide more predictive, fair, and personalized assessments. AI can process non-traditional data points, such as social media activity or e-commerce transactions, which traditional systems simply cannot accommodate. This provides a more comprehensive view of a borrower’s financial behavior, especially for those with limited or no traditional credit history.
The Limitations of Traditional Credit Scoring Methods
1. Dependence on Historical Data
Traditional credit scoring methods rely heavily on historical data, such as past credit usage, payment history, and outstanding debt. While this is useful, it can be restrictive. For individuals without a long credit history, like young adults or newcomers to a country, these methods might fail to provide a reliable picture of their creditworthiness.
Furthermore, the emphasis on past behavior may not account for current financial circumstances or changes in behavior. A person who has recently lost a job or experienced a significant financial setback may have a low credit score, even if they are now financially stable.
2. Lack of Real-Time Updates
Another shortcoming of traditional credit scoring is its lack of real-time updates. Once a score is calculated, it remains static until the next reporting cycle. This delay can be problematic, as an individual’s financial situation may change rapidly. For instance, a borrower may receive a pay raise or improve their debt-to-income ratio, but their credit score will not reflect these changes until the next reporting cycle, which could be months away.
3. Bias and Accessibility Issues in Conventional Systems
Traditional credit scoring models are also known to be biased. Studies have shown that these models can disproportionately disadvantage certain demographic groups, such as racial minorities or low-income individuals. This is often due to systemic biases present in the data itself, which may not reflect the broader financial behavior of different groups.
Moreover, traditional systems can be inaccessible to people who lack a formal credit history, such as young people, immigrants, or individuals who prefer using cash over credit. These individuals are often deemed “credit invisible” and are excluded from financial services, despite having the ability to manage debt responsibly.
How AI Transforms Credit Scoring
Machine Learning and Data-Driven Insights
Machine learning (ML) algorithms are the backbone of AI-based credit scoring. Unlike traditional models, ML algorithms are designed to continuously learn and improve as they process more data. By analyzing a larger set of factors, including spending behavior, income trends, and even how a person interacts with various digital platforms, AI can predict an individual’s future creditworthiness with much higher accuracy.
These algorithms can also identify hidden patterns in data that might be overlooked by humans, making them more effective in spotting potential risks or opportunities that would be invisible in conventional systems.
The Role of Alternative Data Sources
One of the most significant advantages of AI in credit scoring is its ability to integrate alternative data sources. In addition to traditional credit reports, AI systems can analyze data from non-traditional sources such as social media profiles, e-commerce transactions, utility bills, and even mobile phone usage.
For example, a person who regularly pays their utility bills on time or has a history of steady income through freelance work can be assessed for creditworthiness, even if they have never taken out a loan or used credit cards. By tapping into this wealth of alternative data, AI can offer a much broader and fairer credit assessment.
AI’s Ability to Enhance Predictive Accuracy and Fairness
AI systems are designed to be more predictive than traditional models. They don’t just evaluate past behavior; they forecast future actions based on real-time data. This allows lenders to make more informed decisions, improving the chances of extending credit to individuals who may have been overlooked by traditional scoring systems.
Additionally, AI reduces bias by incorporating a wider range of data points. This creates a more accurate reflection of a borrower’s financial behavior and reduces the discriminatory effects that can arise from relying solely on credit scores.
AI Credit Scoring in Practice
AI-powered credit scoring is being implemented across various industries, transforming how credit is extended and how risk is assessed.
1. Banking and Lending Institutions
In traditional banking, credit scoring has long been a key component of loan origination and approval processes. However, AI is changing the way lenders assess borrowers. By analyzing alternative data sources, AI can provide more accurate assessments, especially for individuals with thin or no credit files. As a result, more people are gaining access to credit, and lenders are able to make better, more informed lending decisions.
2. Insurance Providers
AI is also making waves in the insurance sector. Insurers use AI to predict a customer’s ability to pay premiums and assess overall risk more effectively. AI models can analyze the relationship between credit scores and other factors, such as lifestyle choices or spending patterns, to provide more tailored insurance products. This leads to fairer, more personalized pricing for customers, while helping insurers better manage their portfolios.
3. E-commerce Platforms and Fintech
E-commerce and fintech companies are increasingly leveraging AI to assess creditworthiness in real-time. By analyzing customer purchasing behavior, transaction history, and even social data, AI can quickly determine whether to approve financing or credit. This technology makes it easier for consumers to get instant access to loans or credit options, while also helping businesses mitigate lending risks and enhance customer satisfaction.
4. Rental Property and Tenant Risk Management
Landlords and property managers are adopting AI-based credit scoring models to assess tenant applications more effectively. By incorporating alternative data like rental history, utility payments, and even social media activity, AI provides a more accurate picture of a tenant’s ability to meet their financial obligations. This helps reduce tenant turnover and ensures that landlords select tenants who are more likely to pay rent on time.
Case Studies: Real-World Examples of AI Credit Scoring Success
Upstart (Lending Industry):
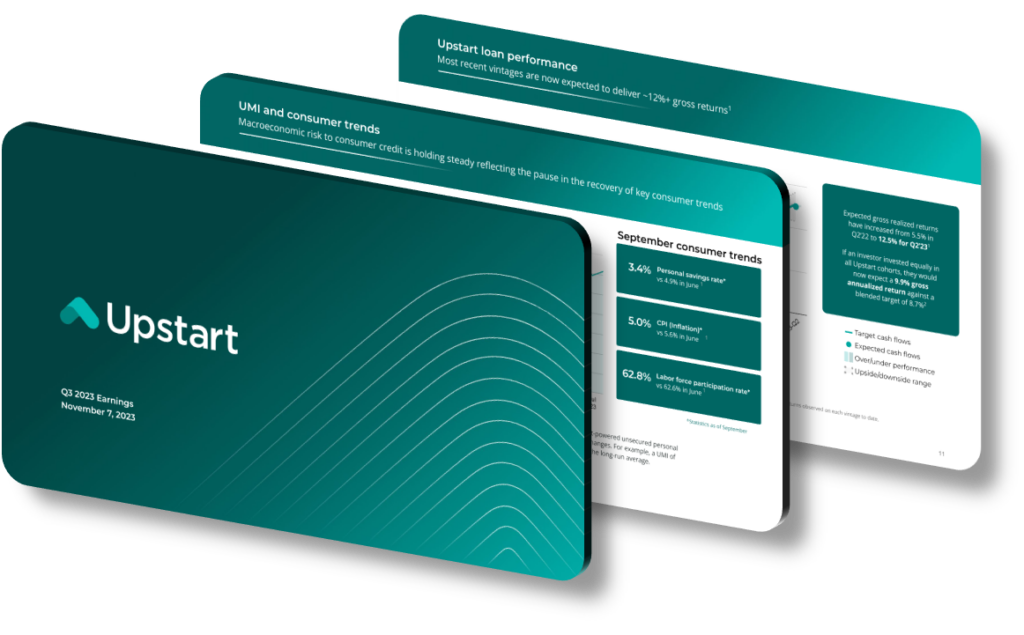
Upstart’s AI Lending Success
Upstart is a lending platform that uses AI to assess credit risk and determine loan eligibility. Unlike traditional credit scoring, which relies on a limited set of data, Upstart incorporates factors like education, work experience, and employment history into its evaluation process. This enables Upstart to approve loans for individuals who may lack traditional credit histories, such as recent graduates. By doing so, the platform has been able to approve more loans and reduce defaults by 75%, making lending more inclusive and accessible to a broader range of borrowers.
Zest AI (Financial Services Industry):
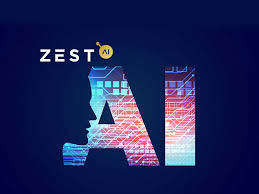
Zest AI
Zest AI is a company that uses machine learning to enhance the accuracy of credit scoring within the financial services industry. Their technology analyzes alternative data sources—such as utility payments, rental history, and other financial behaviors typically excluded from traditional models—to provide a more comprehensive evaluation of a borrower’s creditworthiness. This results in better lending decisions for financial institutions and more favorable terms for consumers, particularly those who may have limited credit histories. Zest AI’s model improves lending accuracy by 20%, helping financial institutions reduce defaults and offer fairer loan conditions.
LenddoEFL (Fintech and Emerging Markets):
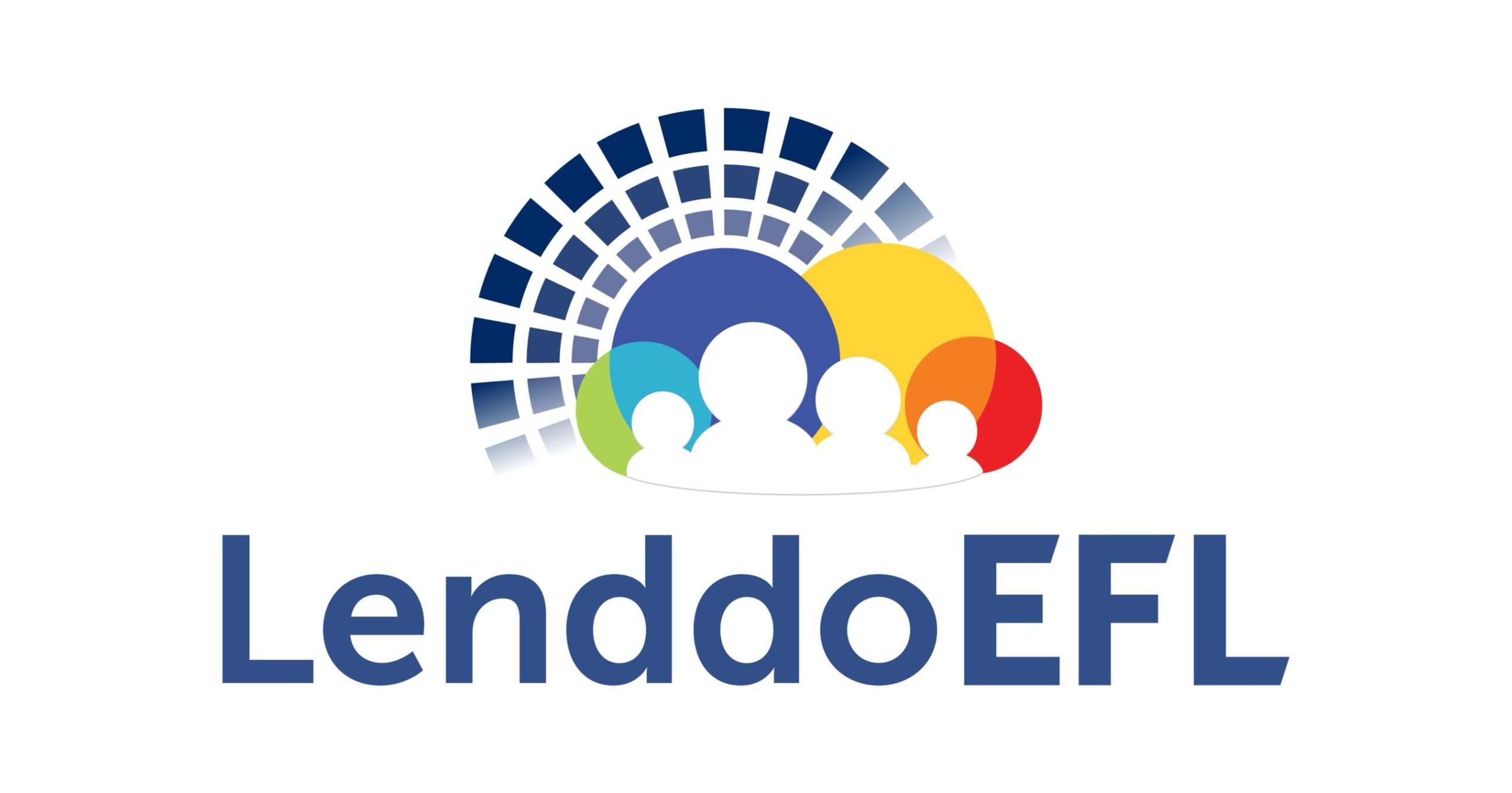
LenddoEFL
LenddoEFL operates in the fintech industry, focusing on integrating non-traditional data sources, such as social media activity and online behaviors, to assess creditworthiness. This approach is particularly useful for individuals who are “credit invisible,” meaning they don’t have access to traditional credit data. By analyzing data from online behavior and social interactions, LenddoEFL provides more inclusive credit scores, helping underserved populations, particularly in emerging markets, access financial products. This innovative method has led to lower default rates and expanded credit access for people previously excluded from the traditional credit system.
These case studies demonstrate the transformative power of AI in credit scoring, offering more accurate, inclusive, and fair lending practices across different industries.
Key Features of AI-Based Credit Scoring Systems
AI-powered credit scoring systems offer numerous advantages that transform how financial institutions assess creditworthiness and manage risk. These systems utilize advanced technologies to automate and optimize the credit evaluation process, ensuring faster, more accurate, and more secure outcomes.
1. Automation and Scalability
AI credit scoring systems are highly automated, reducing manual intervention and speeding up the evaluation process. These systems can handle large volumes of applications efficiently, making them ideal for high-transaction industries like banking and e-commerce. By automating routine tasks, AI enables more strategic decision-making, boosting overall efficiency and cost-effectiveness.
2. Advanced Fraud Detection
AI-powered systems excel at detecting fraud in real-time by analyzing patterns in large datasets. This capability allows AI to identify irregularities faster than traditional methods, continuously evolving to better detect suspicious behavior. This added layer of security protects both lenders and borrowers from fraud and identity theft.
3. Real-Time Decision-Making
AI credit scoring enables real-time decision-making, unlike traditional methods that can take days. This is especially useful in sectors like e-commerce and fintech, where fast approvals are crucial. Instant assessments improve customer experience, increase satisfaction, and boost conversion rates, while also helping businesses mitigate risks with up-to-date data.
4. Customization for Industry Needs
AI credit scoring is highly customizable to meet the specific needs of various industries. Whether for personal loans, insurance premiums, or rental property management, AI can incorporate sector-specific criteria, such as health data or rental history, to offer more accurate and relevant credit assessments.
Challenges of AI in Credit Scoring
Despite the many advantages, the use of AI in credit scoring comes with its own set of challenges that must be addressed to ensure responsible, ethical, and secure implementation.
1. Ethical Concerns: Bias and Fairness
One of the key challenges with AI credit scoring systems is the potential for bias and unfairness. AI models are only as good as the data they are trained on. If the data used to train AI systems contains biases—whether racial, gender-based, or socioeconomic—the resulting credit scores may also reflect these biases. For instance, if a model is trained on historical data that excludes certain demographics, it could unintentionally disadvantage groups with little to no credit history. To combat this, businesses must implement bias detection measures and ensure that their AI models are continually updated with diverse, representative datasets.
2. Data Privacy and Security Risks
AI-driven credit scoring relies on vast amounts of sensitive personal data, raising concerns about data privacy and security. Protecting this data is critical, as breaches can lead to identity theft, fraud, and loss of consumer trust. Financial institutions must comply with privacy regulations such as GDPR and CCPA to ensure that consumer data is handled responsibly. Additionally, businesses should implement robust cybersecurity measures, such as encryption and multi-factor authentication, to safeguard the data used in AI models and prevent unauthorized access.
3. Transparency in AI Decision-Making
Another significant challenge is the opacity of AI decision-making processes. Many AI models operate as “black boxes,” meaning that their inner workings are not easily understood, even by the companies that deploy them. This lack of transparency makes it difficult for consumers to understand how their credit score was determined, potentially eroding trust in the system. To address this, AI systems should be designed with explainability in mind, allowing businesses to provide clear, understandable reasons for credit decisions to consumers.
Ensuring Responsible Use of AI Credit Scoring
To ensure that AI credit scoring systems are used responsibly, businesses must adhere to ethical guidelines and comply with regulatory standards.
1. Regulatory Frameworks and Compliance
As AI becomes more integrated into financial services, governments and regulators must establish clear frameworks for its use. Regulatory bodies need to set standards for how AI is applied in credit scoring, ensuring that these systems are used in ways that are fair, transparent, and nondiscriminatory. Compliance with privacy laws like GDPR and CCPA is also essential to maintaining consumer trust and safeguarding data.
2. Ethical AI Principles and Practices
To build trust in AI-powered credit scoring, businesses must follow ethical principles in their AI practices. This includes ensuring that AI systems are transparent, equitable, and free from discrimination. Regular audits of AI models and the use of diverse data teams during development can help ensure fairness. Additionally, businesses should prioritize accountability in their AI processes by implementing mechanisms for human oversight and intervention when needed.
3. Strategies for Bias Mitigation
To address bias in AI credit scoring systems, businesses can incorporate bias detection and fairness algorithms during the training phase. It’s also important to regularly update and diversify training datasets to include a broad range of consumer behaviors. By ensuring that AI models are exposed to diverse and representative data, businesses can reduce the risk of discrimination and provide fairer credit assessments to all consumers.
Future Trends in AI Credit Scoring
As AI continues to evolve, several trends are shaping the future of credit scoring.
1. Integration of Blockchain for Transparency
One of the most exciting innovations in AI credit scoring is the potential integration of blockchain technology. Blockchain’s decentralized, immutable ledger offers an opportunity to increase transparency and security in the credit scoring process. With blockchain, consumers can have more control over their credit data and access verifiable records of how credit decisions were made. This added layer of transparency could revolutionize how credit scoring is perceived and trusted by both consumers and businesses.
2. Expansion of Alternative Data Utilization
AI systems are increasingly using alternative data to assess creditworthiness, and this trend is expected to grow. In addition to traditional credit history, alternative data sources such as utility payments, rent history, and even social media activity are being used to build a more complete picture of an individual’s financial behavior. As AI technology advances, the scope of alternative data will likely expand, enabling even more accurate and inclusive credit scoring models.
3. Innovations in Real-Time Scoring and Global Adoption
Real-time credit scoring is expected to become even more sophisticated, allowing businesses to assess creditworthiness instantly. With real-time access to credit data, lenders can make faster, more informed decisions, benefiting consumers with immediate credit approval. As AI-powered credit scoring systems gain acceptance worldwide, we may also see increased global adoption, facilitating cross-border lending and promoting financial inclusion in emerging markets.
Conclusion
AI is transforming the credit scoring landscape, offering more accurate, fair, and efficient ways to assess creditworthiness. With its ability to analyze large datasets, incorporate alternative data, and provide real-time assessments, AI provides significant advantages over traditional credit scoring methods. However, to ensure the responsible and ethical use of AI, businesses must prioritize transparency, fairness, and data security. As AI continues to evolve, businesses that adopt AI-based credit scoring systems will be better positioned to provide improved financial services and enhance risk management.
At SmartDev, we are committed to helping businesses leverage cutting-edge AI technologies. If you’re looking to enhance your credit scoring system or explore AI innovations for your financial services, contact us today to see how we can help you optimize your operations with AI-powered solutions.