In the fast-paced manufacturing world, every second of machine downtime directly impacts productivity and profitability. Unplanned downtime costs manufacturers an average of $260,000 per hour—a staggering figure underscoring the urgency of keeping equipment running smoothly.
Yet, many manufacturers still struggle with unexpected equipment failures, often caught off guard by costly breakdowns that disrupt production schedules and erode profitability.
This challenge has been introduced previously. Manufacturers have relied on reactive or preventive maintenance approaches with inherent limitations for decades. Reactive maintenance, where machines are repaired after a failure, often results in unanticipated production halts, while preventive maintenance, performed on a set schedule, can be time-consuming and inefficient, leading to unnecessary downtime and wasted resources.
Enter Predictive Maintenance (PdM), a cutting-edge solution to address these inefficiencies head-on. Leveraging the power of Artificial Intelligence (AI), PdM uses real-time data to monitor equipment performance and predict failures before they happen.
Rather than reacting to breakdowns or servicing machines based on a fixed schedule, predictive maintenance empowers manufacturers to maintain equipment only when needed, significantly reducing downtime and extending the lifespan of critical assets.
This blog explores how AI-driven predictive maintenance transforms manufacturing by reducing downtime, optimizing operations, and ultimately boosting the bottom line.
Through advanced analytics, AI enables a proactive approach to equipment management that revolutionizes how manufacturers operate, creating a future where downtime becomes a thing of the past.
1. The Evolution of Maintenance Strategies in Manufacturing
Figure 1: Evolution of maintenance
For decades, manufacturers have grappled with the delicate balance between maintaining equipment efficiency and minimizing operational downtime.
Traditionally, two primary maintenance approaches have dominated the industry: reactive and preventive. While both have served their purpose, they come with significant limitations that have prompted the search for more intelligent, efficient solutions.
Reactive maintenance, as the name suggests, involves addressing equipment issues only after a failure. This approach can lead to unanticipated production halts, as equipment is often pushed to its limits before repairs are initiated.
The unpredictability of reactive maintenance frequently results in costly downtime, missed production targets, and potential damage to other systems or processes reliant on the failed machine. Though it might seem cost-effective in the short term, the long-term consequences of unexpected breakdowns can be substantial.
On the other hand, preventive maintenance seeks to mitigate these risks by scheduling regular maintenance based on a fixed timeline or usage milestones. While this approach reduces the likelihood of sudden equipment failure, it introduces its challenges.
Servicing machines that may require little attention often leads to unnecessary downtime and inflated maintenance costs. Equipment is taken offline according to the calendar, not its actual condition, which means valuable production hours may be lost for no substantial gain. Additionally, parts and labor are expended on machinery that might still be operating at optimal levels.
This brings us to the need for a more innovative approach combining the best of both worlds without drawbacks. The limitations of reactive and preventive maintenance underscore the demand for a predictive and proactive strategy.
Enter predictive maintenance (PdM), a revolutionary evolution in maintenance strategies. By leveraging AI and real-time data, PdM allows manufacturers to monitor equipment health and predict failures before they happen.
Rather than reacting to breakdowns or adhering to rigid schedules, predictive maintenance optimizes maintenance activities, ensuring that machines are serviced only when necessary. This reduces downtime, extends the operational life of critical assets, and significantly lowers costs.
As manufacturing becomes more sophisticated, the evolution from reactive and preventive maintenance to AI-powered predictive maintenance is a critical shift, enabling greater efficiency, reliability, and profitability in an increasingly competitive landscape.
2. What is Predictive Maintenance (PdM)?
Predictive Maintenance (PdM) represents a transformative approach to equipment upkeep designed to anticipate and prevent failures before they occur. Unlike reactive maintenance, which waits for a breakdown to initiate repairs, or preventive maintenance, which follows a scheduled timetable regardless of actual equipment condition, PdM continuously monitors the real-time performance of machinery.
It leverages advanced technologies to predict potential issues based on data, allowing manufacturers to perform maintenance only when necessary. This proactive approach minimizes unplanned downtime, optimizes machine usage, and reduces maintenance costs while extending critical assets’ lifespan.
At the heart of predictive maintenance is the ability to collect, analyze, and act upon vast amounts of data. It provides insight into the condition of equipment by monitoring performance metrics such as vibration, temperature, and pressure levels.
Using this real-time data, PdM systems can detect early signs of wear or malfunction, enabling maintenance teams to address potential issues long before they lead to costly breakdowns.
This shift from reactive to predictive strategies empowers manufacturers to maximize efficiency and operational continuity.
Figure 2: Predictive Maintenance (PdM)
Key Technologies Enabling PdM
The effectiveness of predictive maintenance hinges on several core technologies, each vitally involved in gathering, analyzing, and interpreting data.
Internet of Things (IoT): IoT devices are the foundation of PdM, acting as the eyes and ears of the system. Sensors embedded in manufacturing equipment capture vast amounts of operational data, from temperature fluctuations and vibration levels to pressure and fluid dynamics.
These sensors constantly communicate with the central PdM platform, feeding it real-time data that reflects the current condition of the machinery.
IoT technology enables continuous monitoring and seamless integration across multiple systems and machines, creating a network of interconnected devices that provide a comprehensive view of factory operations.
Artificial Intelligence (AI) & Machine Learning (ML): The sheer volume of data generated by IoT devices would be overwhelming without AI and machine learning. AI algorithms can process and analyze this data to detect patterns, anomalies, and trends that human operators might miss.
Machine learning, a subset of AI, allows the system to learn from historical data and refine its predictive models continuously. Over time, these models become increasingly accurate, enabling the system to predict failures more precisely.
Specific techniques such as anomaly detection and predictive analytics are commonly used in PdM, where the system learns what constitutes normal equipment behavior and flags any deviations that might signal an impending failure.
This predictive capability gives manufacturers early warnings, ensuring maintenance is scheduled before issues escalate.
Figure 3: Predictive Maintenance using IoT
Big Data Analytics: The vast quantities of data generated by industrial machines demand potent data processing and storage capabilities, which is where big data analytics comes into play.
Historical data is essential for developing accurate predictive models, as past performance data provides crucial context for detecting trends and anomalies. Advanced analytics platforms process and store this data, enabling AI algorithms to access and analyze it in real-time.
These platforms can handle complex datasets, combining current and historical data to provide comprehensive insights into equipment health. With these analytics, manufacturers can predict future failures and uncover patterns that help optimize overall equipment efficiency and reduce the frequency of future breakdowns.
Together, these technologies form the backbone of predictive maintenance – a system that transforms raw data into actionable insights, allowing manufacturers to stay ahead of equipment failure and significantly reduce downtime.
By integrating IoT sensors, AI-driven analytics, and big data, PdM enables manufacturers to move from a reactive mindset to one that is predictive, proactive, and deeply aligned with efficiency and profitability goals.
3. How AI-Driven Predictive Maintenance Reduces Downtime in Manufacturing Industry
AI-driven predictive maintenance has emerged as a game-changer in modern manufacturing. It offers a proactive approach that minimizes downtime and maximizes efficiency.
By leveraging advanced AI models, manufacturers can detect early signs of equipment degradation before they escalate into full-scale failures. This approach prevents costly disruptions and ensures that maintenance is strategically timed to optimize production schedules.
3.1 Proactive Identification of Issues
AI models excel at detecting subtle patterns and anomalies in equipment performance that may elude human observation. By analyzing real-time data collected from sensors, AI can identify early indicators of wear and tear, such as changes in vibration, temperature, or pressure. These early warning signs allow maintenance teams to intervene before minor issues become major problems.
For example, a leading automotive manufacturer implemented AI-driven predictive maintenance to monitor CNC machines. The AI model detected unusual vibration patterns in a critical machine, signaling potential bearing wear.
By addressing the issue early, the company avoided a complete machine failure, saving over 200 hours of production time and preventing significant revenue loss. This case illustrates the power of AI in spotting early-stage problems, enabling manufacturers to address issues proactively rather than reactively.
Figure 4: Benefits of Machine Learning for Predictive Maintenance
3.2 Minimized Unplanned Downtime
One of predictive maintenance’s primary benefits is its ability to schedule repairs during planned downtime or periods of lower production demand.
Instead of waiting for equipment to fail, often leading to unplanned and costly disruptions, AI-driven systems optimize maintenance schedules to ensure minimal impact on operations.
4. AI Predictive Maintenance in Action: Case Studies and Use Cases
AI-driven predictive maintenance has proven transformative in various industries, allowing companies to dramatically reduce downtime, improve operational efficiency, and optimize maintenance costs.
Here, we explore two real-world examples that demonstrate the power of this technology in action.
4.1 Global Automotive Manufacturer
One of the most notable success stories comes from a leading global automotive manufacturer that adopted AI-powered predictive maintenance across its production lines. Machine uptime is critical to ensuring smooth production in the automotive industry, and any unplanned downtime can have significant financial and operational impacts.
This manufacturer could monitor equipment conditions in real time by incorporating machine learning algorithms into its maintenance strategy.
The AI system collected data from IoT sensors attached to critical machinery, tracking variables such as vibration, temperature, and pressure.
The machine learning model analyzed this data continuously, identifying subtle anomalies that indicated potential future failures. By doing so, the system could flag issues before they become severe, allowing maintenance to be scheduled during non-critical times, such as planned downtime.
This proactive approach resulted in a 20% improvement in machine uptime across the entire production line.
The company also saw a marked reduction in emergency repairs and spare part usage, which led to significant cost savings. These operational improvements translated to increased production efficiency and overall profitability while reducing the risk of supply chain disruptions.
Additionally, AI-driven insights allowed the manufacturer to optimize its spare parts inventory, reducing the need for on-site excess parts and lowering overhead costs.
The predictive maintenance model’s ability to deliver real-time insights into machinery performance provided the company with a new level of control over its production environment, helping it to meet production targets more reliably.
4.2 Food & Beverage Industry
The food and beverage industry, known for its rigorous production demands and the critical importance of maintaining product freshness, has also reaped the benefits of AI-driven predictive maintenance.
A major processed food manufacturer faced repeated challenges with unexpected equipment breakdowns, which caused frequent production stoppages and threatened product quality and shelf life. The company implemented an AI-based predictive maintenance system that monitored key equipment performance, such as mixers, ovens, and conveyor belts to combat this.
By collecting and analyzing data from these machines in real time, the AI system could detect early signs of degradation—such as abnormal temperature changes in ovens or increased energy usage in mixers—before equipment failure occurred.
The benefits were clear. The manufacturer reported a 25% improvement in Overall Equipment Effectiveness (OEE), directly contributing to reducing unscheduled downtime.
Additionally, the AI system allowed the company to predict and schedule maintenance during off-peak hours, ensuring production continuity during critical periods. This uptime improvement enhanced operational efficiency and safeguarded the company’s product quality, essential in an industry where even minor disruptions can impact freshness and delivery schedules.
By enabling proactive rather than reactive maintenance, the company experienced a 30% reduction in maintenance costs. The AI system allowed for better planning and resource allocation, eliminating the need for last-minute emergency repairs, which are typically more expensive due to labor costs and rush parts delivery.
This success has encouraged other food and beverage manufacturers to explore AI-driven maintenance solutions to ensure higher operational reliability.
4.3 Use Cases Across Industries
Predictive maintenance is particularly effective for equipment prone to wear and tear, such as CNC machines, conveyor belts, and turbines.
For example, in energy production, AI systems monitor turbines to identify abnormal vibrations or temperature changes, predicting mechanical failures before they occur.
Similarly, conveyor belts in logistics and manufacturing facilities are monitored for signs of misalignment or overuse, allowing for timely repairs that prevent full-scale breakdowns.
AI-driven predictive maintenance empowers manufacturers to take control of their maintenance strategies. Through proactive issue identification, minimizing unplanned downtime, optimized maintenance scheduling, and significant cost reductions, predictive maintenance represents a key enabler of operational excellence in modern manufacturing.
By adopting this advanced technology, companies can reduce downtime and unlock new efficiency and profitability levels.
5. AI in PdM: Challenges and Considerations
While the benefits of AI-driven predictive maintenance are substantial, successfully implementing such systems requires overcoming several challenges.
Key considerations include ensuring data quality, integrating with existing infrastructure, managing costs, and addressing skill gaps within the workforce.
5.1 Data Quality & Availability
The foundation of predictive maintenance lies in the quality and availability of data gathered from equipment sensors. AI models rely heavily on real-time, accurate data to predict potential failures and optimize maintenance schedules.
However, data gaps, inaccuracies, or poor sensor calibration can hinder the system’s delivery of meaningful insights. Incomplete or incorrect data can lead to false predictions, resulting in unnecessary maintenance or overlooked issues that could lead to equipment failure.
One of the primary challenges is ensuring continuous data flow from all critical machinery. Legacy machines, which may not have built-in sensors or generate minimal data, require retrofitting with IoT sensors to achieve the desired level of data comprehensiveness.
Additionally, data silos across various systems can complicate efforts to collect a unified, holistic view of machine performance. The solution often involves deploying advanced data management and preprocessing systems that can clean and standardize data inputs across the board. This ensures that the AI models work with accurate, relevant information.
5.2 Integration with Existing Systems
Many manufacturers find it challenging to integrate AI-driven predictive maintenance systems with legacy infrastructure. Manufacturing environments often consist of a patchwork of systems, machines of varying ages, and disparate software platforms that have been layered together over time.
Integrating AI solutions into these environments can be challenging, as many older systems cannot communicate with modern IoT sensors and AI platforms.
Ensuring compatibility between existing systems and the new AI-driven maintenance platforms is essential for a seamless transition. This process may involve upgrading legacy equipment, modifying software protocols, or adopting middleware solutions that enable data flow between old and new systems.
Additionally, organizations may face challenges in creating an infrastructure that supports real-time data processing and analysis, particularly if legacy systems were not designed to handle the volumes of data generated by AI-powered predictive maintenance solutions.
5.3 Cost & ROI
Implementing AI-based predictive maintenance solutions requires a significant initial investment. Costs include purchasing and installing IoT sensors, upgrading equipment, and deploying AI platforms capable of analyzing large-scale data.
While these technologies have the potential to generate substantial savings over time by reducing downtime and optimizing maintenance schedules, the upfront expenses can be a barrier for some companies.
To better understand these expenses and plan your investment, explore our guide on the cost of AI development.
Return on investment (ROI) depends on several factors, including the size of the operation, the criticality of the equipment being monitored, and the frequency of equipment failures. It may take months or even years for companies to fully realize the cost benefits of predictive maintenance, mainly if the initial outlay is high.
However, long-term financial gains can justify the initial costs as predictive maintenance improves overall equipment effectiveness (OEE), reduces emergency repairs, and prolongs equipment lifespan. Companies considering AI-driven predictive maintenance must weigh the short-term financial impact against the long-term benefits to understand the full ROI potential.
5.4 Skill Gaps
Introducing AI and IoT into maintenance processes highlights a growing need to upskill the workforce. Maintenance teams accustomed to traditional, hands-on approaches may navigate unfamiliar territory as AI-driven systems take center stage. These systems require a fundamental understanding of data science, machine learning, and digital platforms—skills that may be lacking in the current workforce.
Organizations must invest in training and development to ensure that employees can maintain the new systems and interpret the predictive insights generated by AI. This may involve hiring new talent with expertise in data analytics or upskilling current employees through targeted training programs focused on understanding AI algorithms, interpreting data patterns, and managing IoT infrastructure.
Bridging the skill gap is critical for maximizing the effectiveness of AI-driven maintenance strategies and ensuring smooth operational transitions.
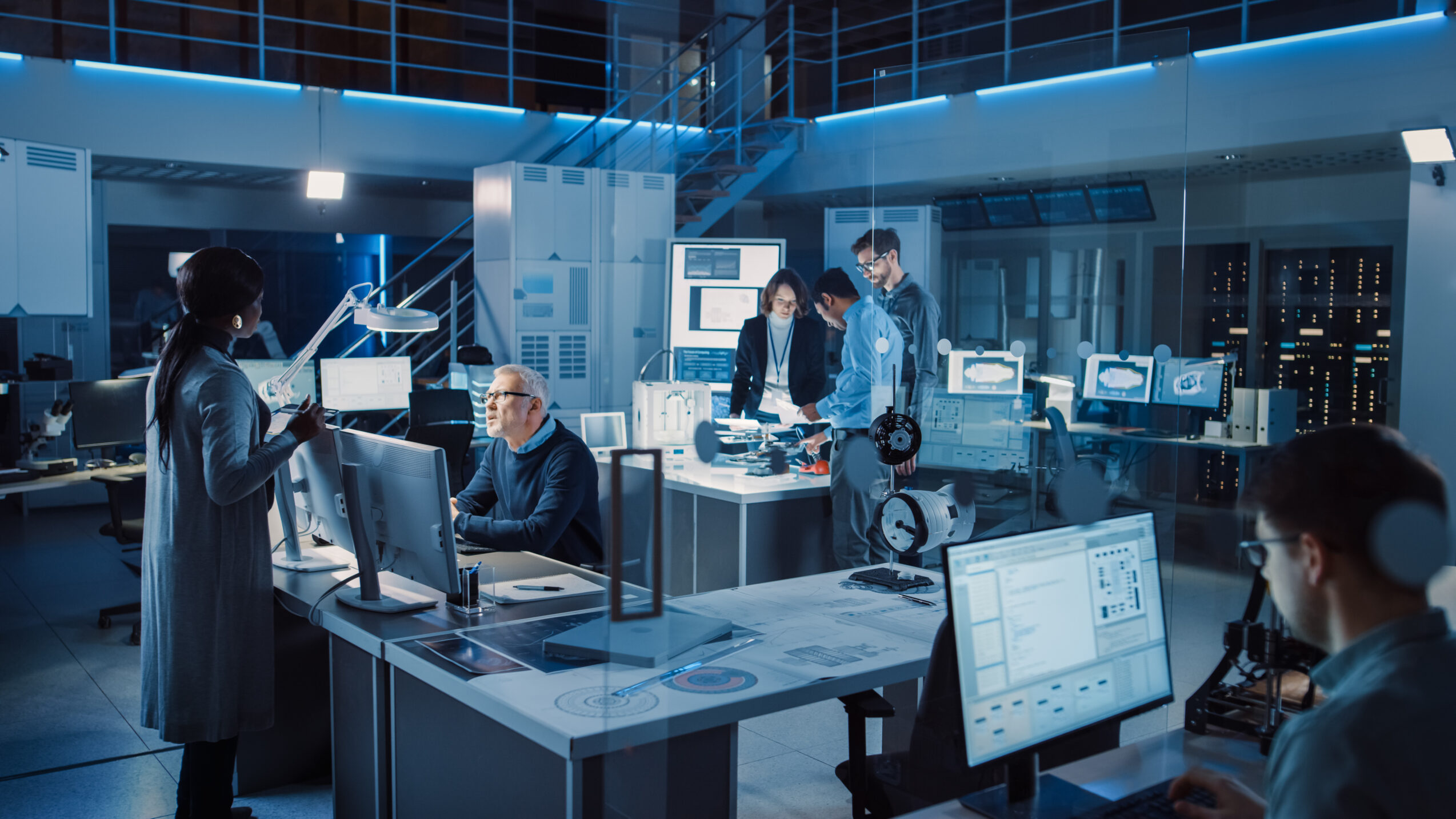
Diverse International Team of Industrial Engineers and Scientists Working in Research Laboratory/Development Center. People Working on New Efficient Engine Concept Design. Professionals Teamwork
In conclusion, while AI-powered predictive maintenance offers substantial advantages, its successful implementation requires addressing data quality, system integration, upfront costs, and workforce readiness challenges. Manufacturers who proactively tackle these hurdles will be better positioned to unlock predictive maintenance’s full potential and drive long-term operational excellence.
6. Summary
AI-driven predictive maintenance is reshaping manufacturing by delivering significant, measurable benefits. By leveraging real-time data and advanced machine learning algorithms, predictive maintenance solutions reduce unplanned downtime, allowing manufacturers to detect equipment issues before they escalate into costly breakdowns. This proactive approach minimizes disruptions, ensuring that production lines remain operational and schedules stay on track.
Moreover, predictive maintenance enhances operational efficiency. Maintenance activities are scheduled only when necessary, eliminating unnecessary maintenance tasks and optimizing resource allocation. This results in more streamlined processes and improved overall equipment effectiveness (OEE), translating into greater production output and consistency.
Regarding cost savings, AI-driven maintenance significantly lowers repair and replacement costs. By predicting failures before they occur, manufacturers avoid expensive emergency repairs and minimize secondary damage to machines. This foresight not only reduces spare parts and labor expenses but also extends the lifespan of critical assets.
Predictive maintenance contributes to higher production quality. By maintaining equipment in optimal condition, manufacturers reduce variability in machine performance, ensuring consistent product quality. This results in fewer defects, higher customer satisfaction, and a more decisive competitive edge in the marketplace.
In sum, AI-powered predictive maintenance is revolutionizing manufacturing by boosting efficiency, cutting costs, and driving sustained improvements in production quality—all while minimizing downtime and paving the way for a more innovative, more resilient future.
7. About SmartDev and Applied AI Lab
As AI continues to revolutionize industries, manufacturers that adopt cutting-edge technologies like predictive maintenance will be best positioned to lead in the rapidly evolving landscape of intelligent manufacturing. Companies that need to integrate AI-driven predictive maintenance risk falling behind, facing increased downtime, inefficiency, and escalating maintenance costs.
Now is the time to explore how AI-powered solutions can predict failures, optimize maintenance schedules, and enhance operational efficiency.
At SmartDev and Applied AI Lab, we specialize in designing tailored AI solutions that address the unique challenges of manufacturing. Whether your goal is to reduce downtime, extend equipment lifespan, or optimize resource allocation, our experts are equipped with the tools and knowledge to guide your organization through the journey of AI-driven predictive maintenance.
Our AI Readiness Assessment helps identify critical areas for AI integration, ensuring a smooth and effective implementation that aligns with your operational goals.
Transform your maintenance strategy with our bespoke AI solutions. Speak with our AI experts today to receive a customized roadmap designed to maximize AI’s impact on improving your production processes and minimizing downtime. CONTACT US HERE to get started.
Future Outlook
As we look to the future, AI will continue to play a pivotal role in shaping manufacturing. The rise of deep learning, edge computing, and cloud-based analytics will further enhance predictive maintenance, providing even greater precision and scalability.
Manufacturers that invest in AI-powered maintenance now will minimize downtime and position themselves as leaders in Industry 4.0, prepared to tackle the challenges of tomorrow’s production environment.
At Applied AI Lab, we believe that AI powers the future of manufacturing, and the future is already here. Let us help you unlock new possibilities for efficiency, innovation, and resilience in your operations.